State of artificial intelligence
Everything you need to know about the fastest-growing sector
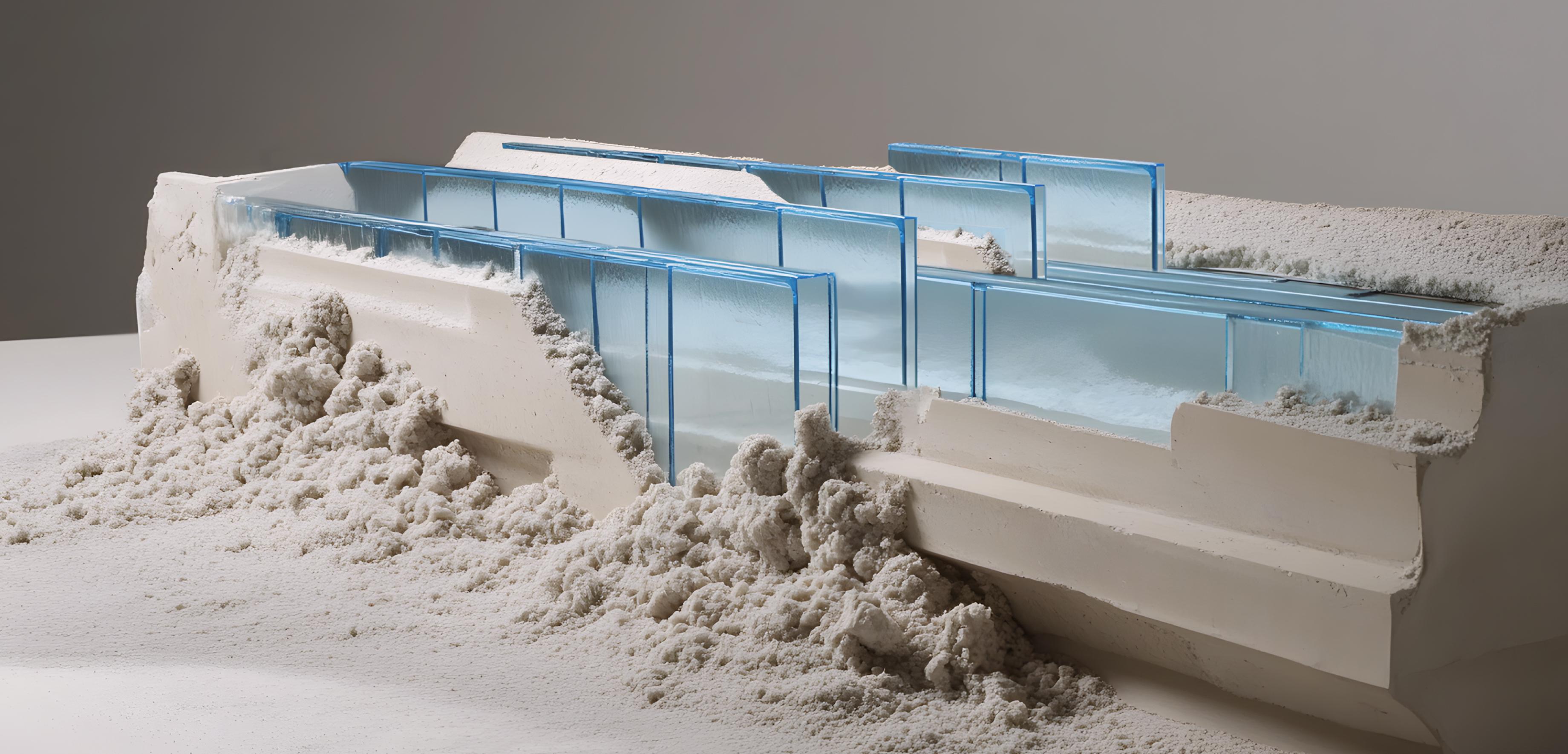
In a world moving at lightning speed, the rise of artificial intelligence brings both opportunities and challenges. No need to worry, though. Vention is here to cut through the noise.
With over 20 years in business and more than a decade leading AI software development projects, we’re right at the heart of this revolution. This report will guide you through the latest AI trends and statistics, delivering deep insights and expert opinions.
Whether you’re a business aiming to boost operations with AI, a developer wondering what the future holds for your role, or a tech enthusiast eager to see where AI is headed, we’ll give you the edge you need to navigate this ever-evolving landscape.
How it all started: key AI milestones
AI hasn’t sprung up overnight. Its origins trace back to early visionaries, including Alan Turing and John McCarthy, who dreamed of intelligent machines. From the first chatterbots to AI capable of beating chess champions, we've come a long way.
Fast forward to today, and AI tools like ChatGPT and DALL-E are transforming industries and everyday life, moving from sci-fi to tangible, productivity-boosting assets.
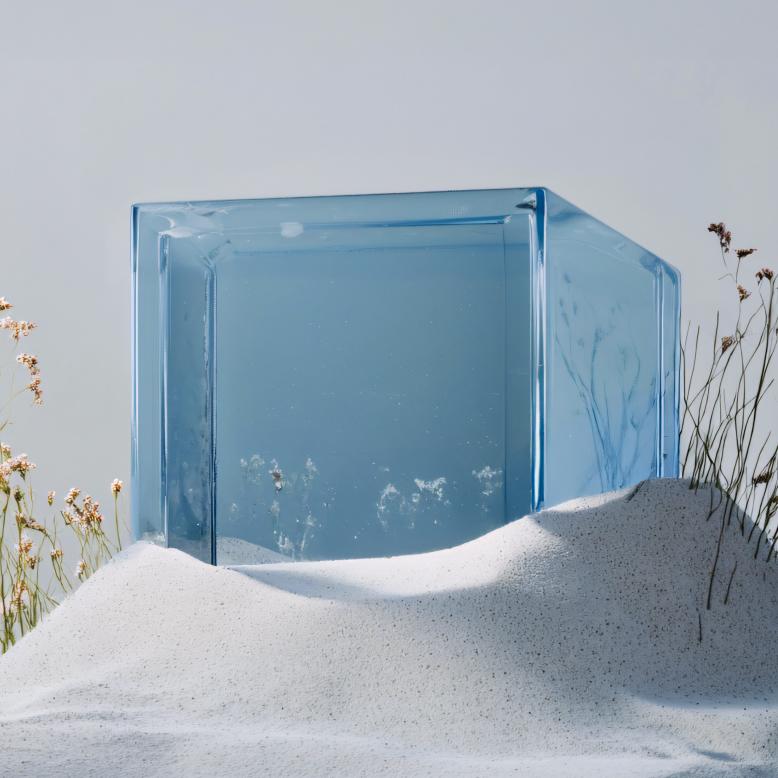
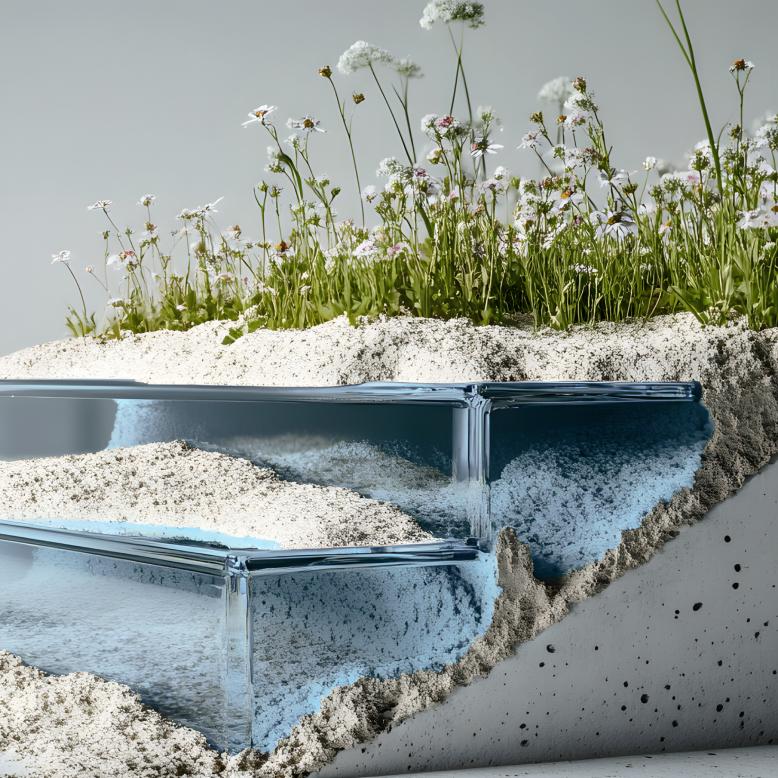
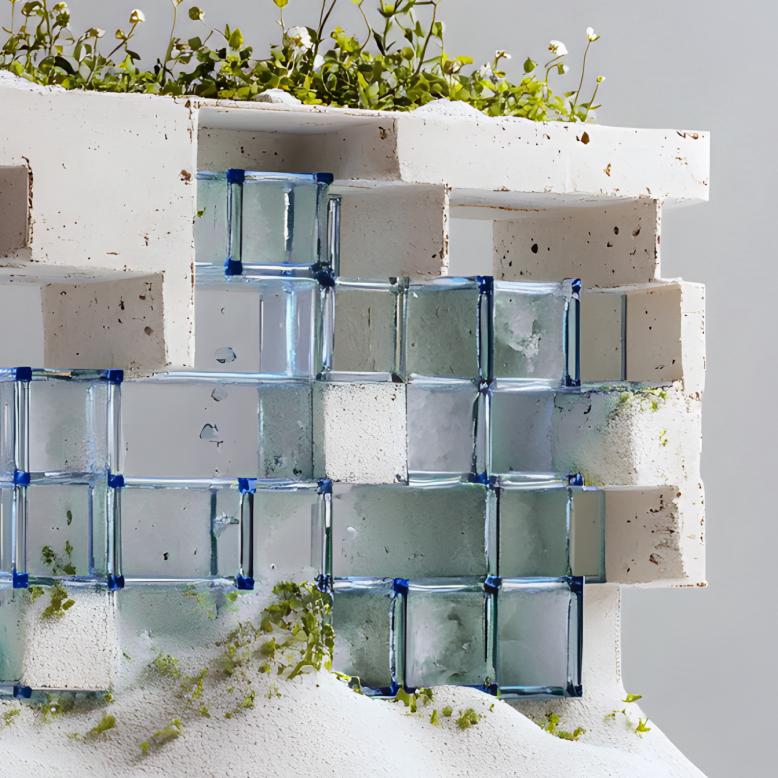
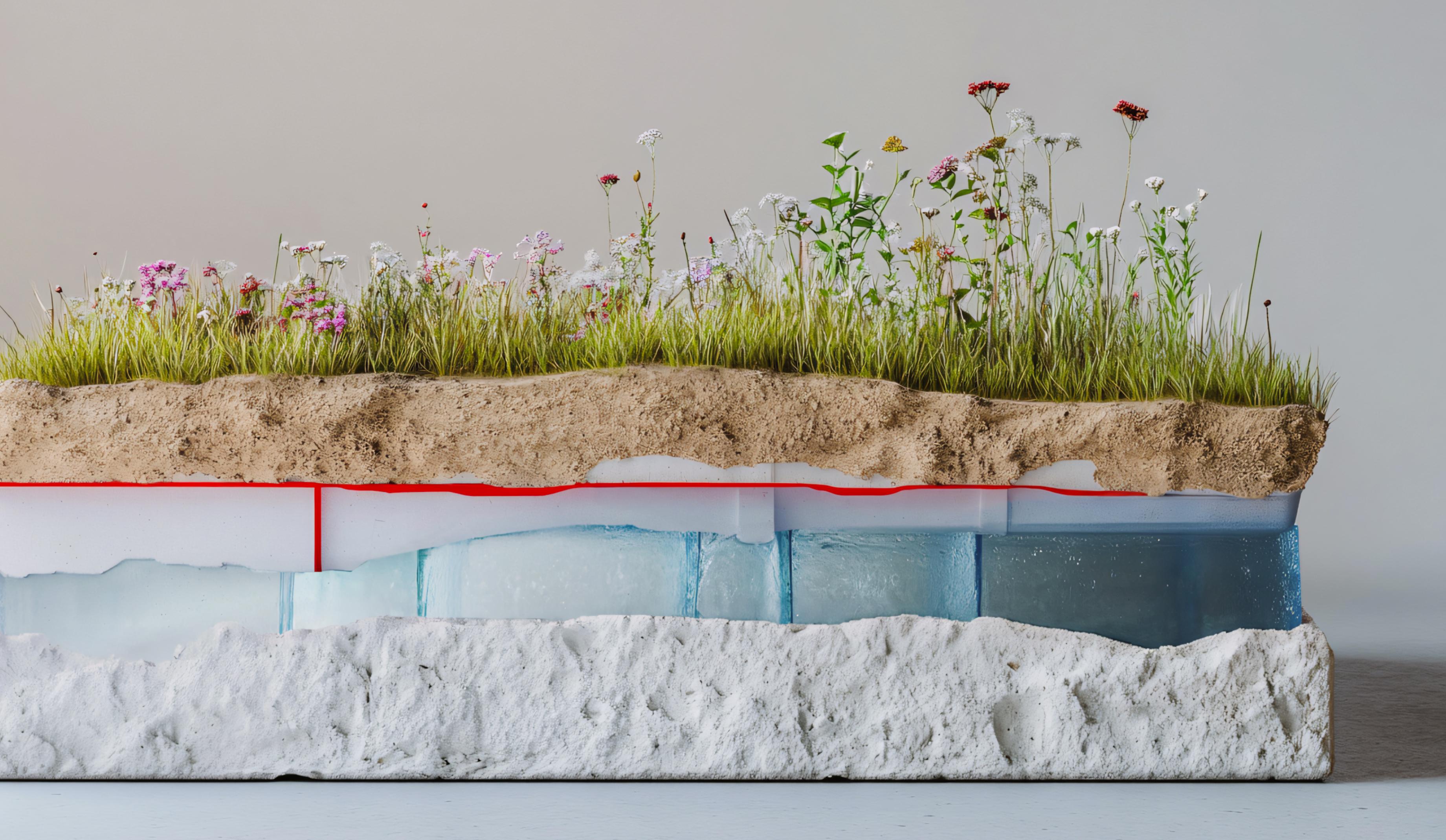
We all know AI is booming — but what does that really look like? One major clue lies in the market itself: its behavior, growth, and cash flow tell the story of just how fast this industry is taking off.
AI funding and deals (2020-2024 YTD)
2024 marked a peak in AI deals, with 4,505 transactions worth $100.4B. The reduction in deal count and value in 2022 and 2023 suggests a strategic shift — companies were focusing on refining and scaling existing products rather than expanding their portfolios indiscriminately.
This indicates a maturing AI industry where sustainable growth and return on investment are prioritized over speculative expansion.
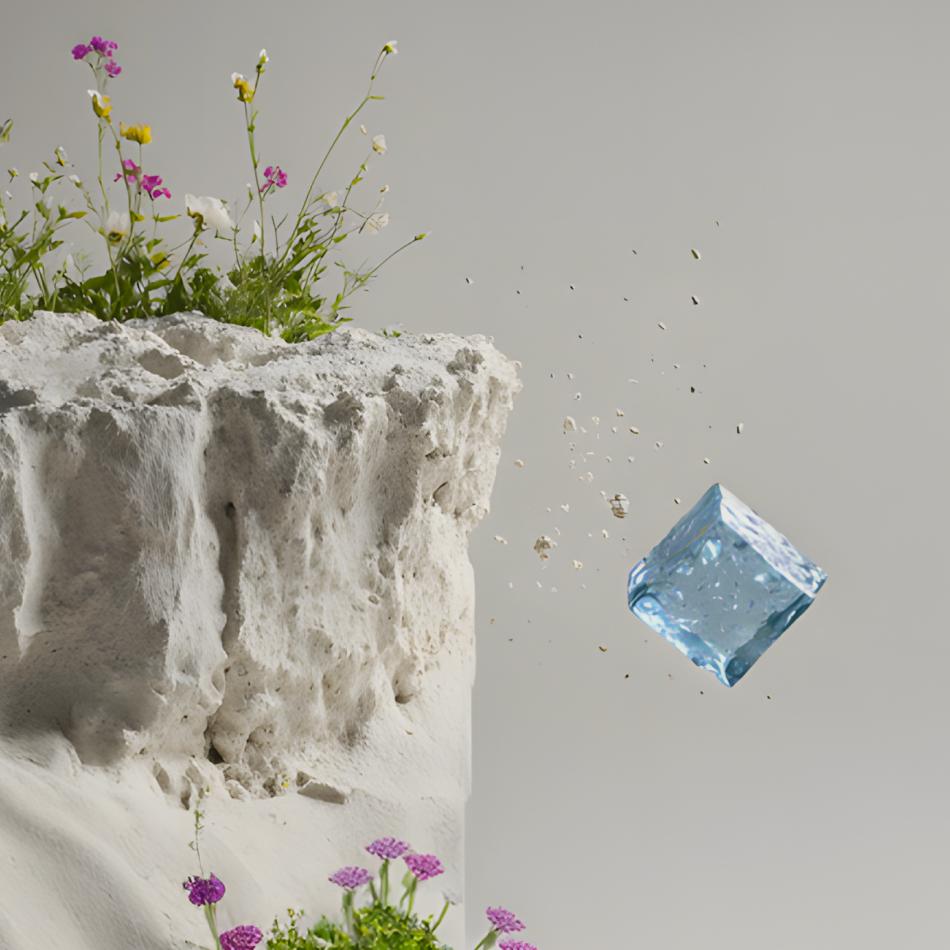
Do you think there are no opportunities for new projects? Think again.
Sustainable growth doesn’t mean the market has slowed down. In fact, 2024 set a new record for funding volumes, reaching $43.8 billion in Q4 — the highest quarterly level ever recorded.
Quarterly AI funding and deals
Deal activity in Q4 2024 also rose by 12.7% QoQ, reaching 1165 transactions. This trend reflects a focus on fewer but more impactful investments, driving meaningful innovation within the industry.
In 2024, most AI deals were closed within the US (1979 deals worth $74B). In Europe, 926 deals were evaluated at $10.9B, while in Asia, $8.8B was spent on 967 investments and acquisitions.
Notably, several high-profile funding rounds in Q4 2024 underscored this focus:
-
Databricks: $10B Series J on December 17, securing a $62B valuation.
-
OpenAI: $6.6B Series B on October 2.
-
xAI: $6B venture round on November 20.
-
Anthropic: $4B corporate round on November 22.
Established companies — think Google and Amazon — have been pivotal in setting this trend, leveraging their resources to make strategic, high-impact bets on AI projects that promise sustainable growth.
Y Combinator companies 2020-2024
While established companies have been key drivers of the AI boom, they are not the only ones benefiting. AI startups like OpenAI, Cohere, and Anthropic are thriving and receiving increased attention from accelerators and investors.
In Y Combinator, the share of AI-related companies surged from 13% in 2020 to 67% in 2024, underscoring the growing appetite for AI innovation across various business stages and sizes. This shift demonstrates that both seasoned players and new entrants see immense potential in AI, paving the way for diverse advancements in the field.
“We can see that AI investment is currently concentrated within larger enterprises. However, this landscape is poised to shift, with AI availability and investment becoming more diverse and distributed across a wider range of companies. As AI technology continues to mature, we anticipate increased adoption and innovation from organizations of all sizes.”
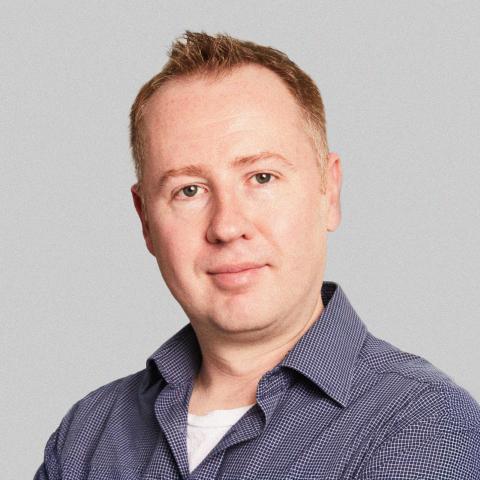
Glyn Roberts, CTO at Vention
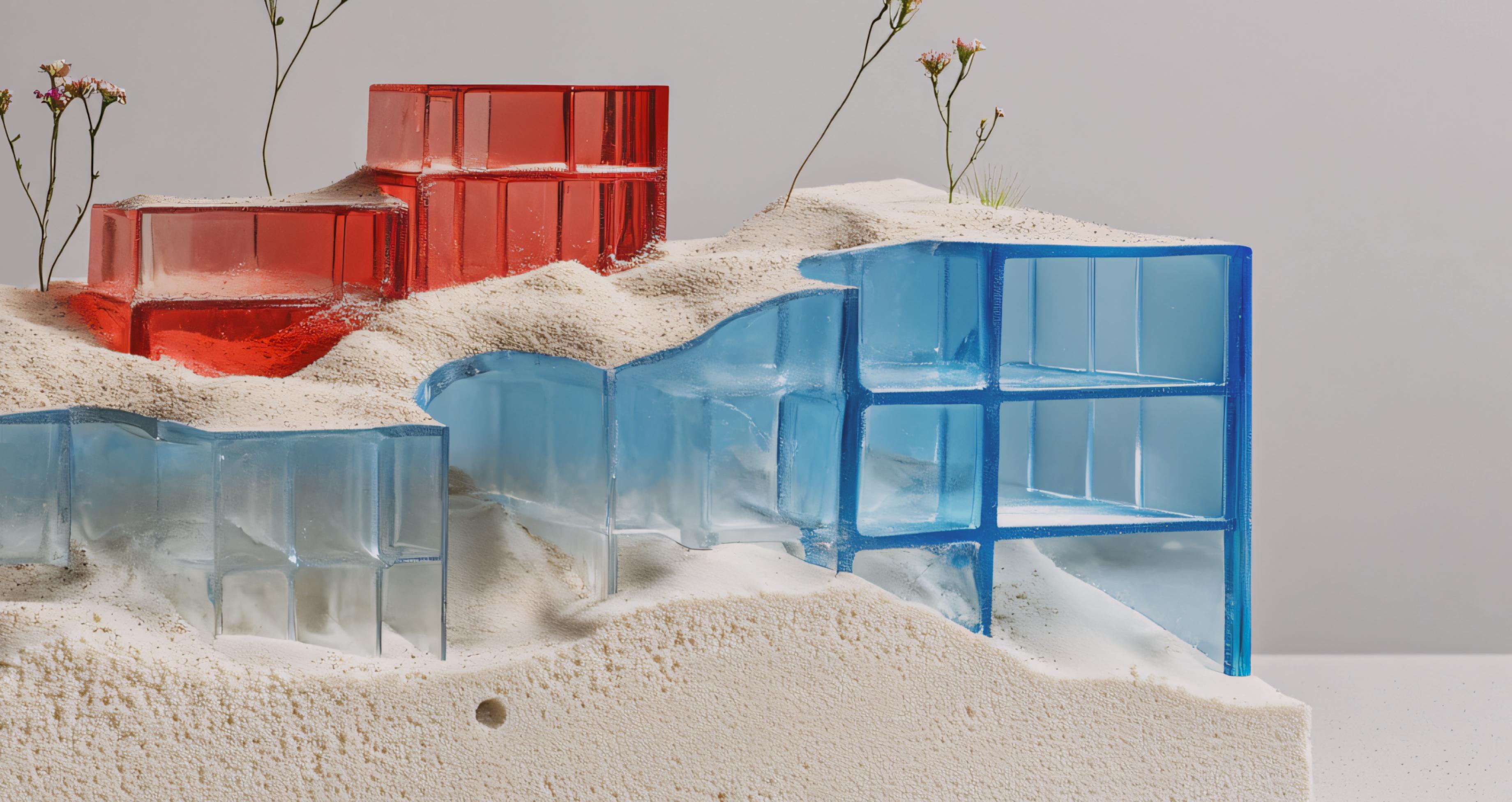
AI: market stats and outlook
The whole market size for AI in 2024 surpassed $184B and will reach $826.7B by 2030, showing an annual growth rate of 28.46%.
Regionally speaking, the largest part of this market is in the US today, accounting for $50.16B.
Who invested in AI the most?
Big tech, of course. In 2024, Amazon poured money into AI 17 times, Google 26 times, Microsoft 23 times, and Nvidia 47 times.
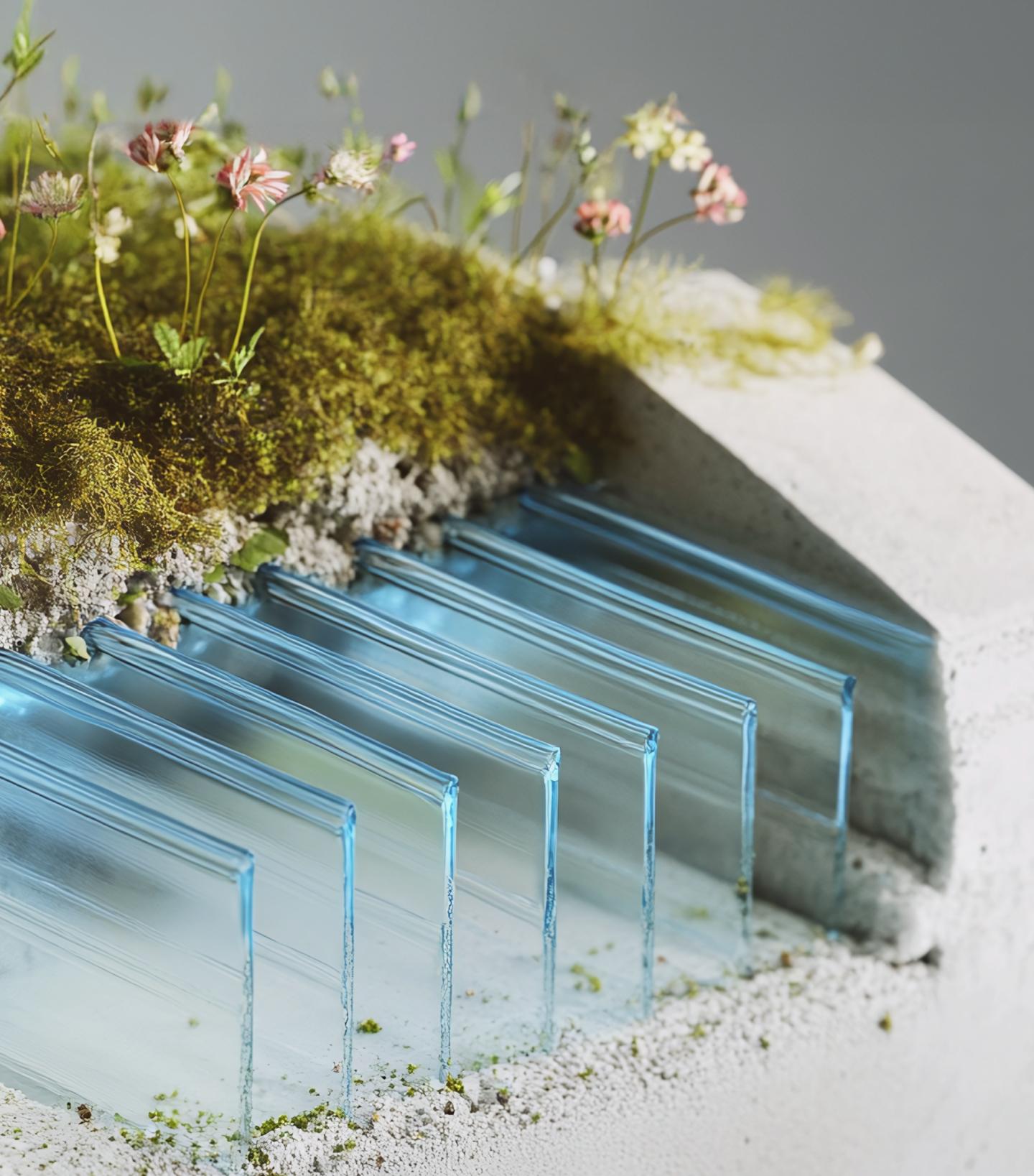
Generative AI: growth potential and real-world impact
In 2024, the generative AI market reached $36.06B and is projected to grow at a remarkable CAGR of 46.47%, surging to $356.10B by 2030. Its share in the broader AI market will expand from 19.59% in 2024 to 43.07% by 2030, highlighting its increasing dominance.
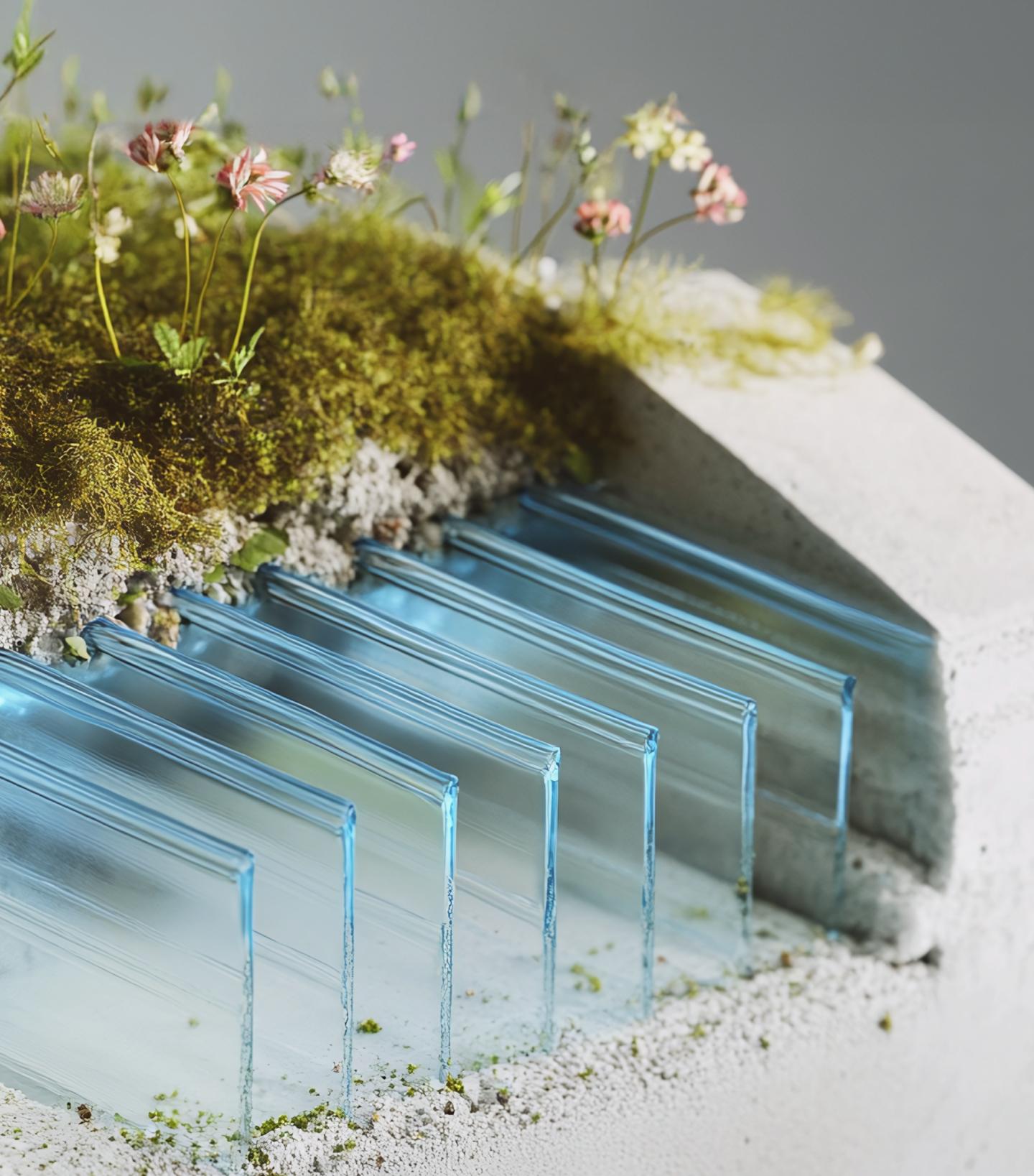
Major tech giants are heavily investing in generative AI. Microsoft holds a 49% stake in OpenAI, Amazon has Amazon Q, Google offers Bard, and Meta launched Meta AI. These investments underline the immense potential of generative AI — not just in creating new solutions, but in transforming the capabilities of entire industries.
The adoption of generative AI is driven by its ability to significantly boost productivity and creativity. Industries such as entertainment, healthcare, and marketing are leveraging generative AI to automate content generation, personalize experiences, and assist in diagnostics. This surge in practical applications demonstrates that generative AI is not only a cutting-edge tool, but also a major driver of AI's overall market growth.
Generative AI market growth
2020
2021
2022
2023
2024
2025
2026
2027
2028
2029
2030
From code to coin: AI impact on business
A market of this magnitude has no chance of leaving anything untouched. For AI, that means a profound influence on both local and global economies. But just how big could that impact be?
Huge. By 2030, AI is estimated to contribute between $17.1T and $25.6T to global GDP. North America and China will be the biggest beneficiaries, with AI adding 14.8% to the US GDP and 26.1% to China's GDP by then.
Which regions will gain most from AI?
The positive impact of AI could boost global productivity by 0.5% to 3.4% by 2040. Why such an enormous range? Because it depends on how early and actively we start implementing AI at the global business level.
AI’s impact on jobs and job market shifts
What’s more tied to the global economy than the job market? Virtually nothing. The job market serves as a pulse check on both global and regional economic health and where they’re headed next.
"AI isn’t just about replacing jobs; it’s about redefining them. While machines take over repetitive tasks with speed and precision, the future belongs to those who can leverage AI to create, innovate, and tackle challenges we haven’t yet imagined. The real danger isn’t losing jobs to AI — it’s not knowing how to work with it effectively.
As AI advances, it will revolutionize sectors like healthcare, education, and climate solutions, opening up entirely new opportunities. At Vention, we’re already bringing AI-driven innovation to these industries, using AI to benefit both businesses and people."
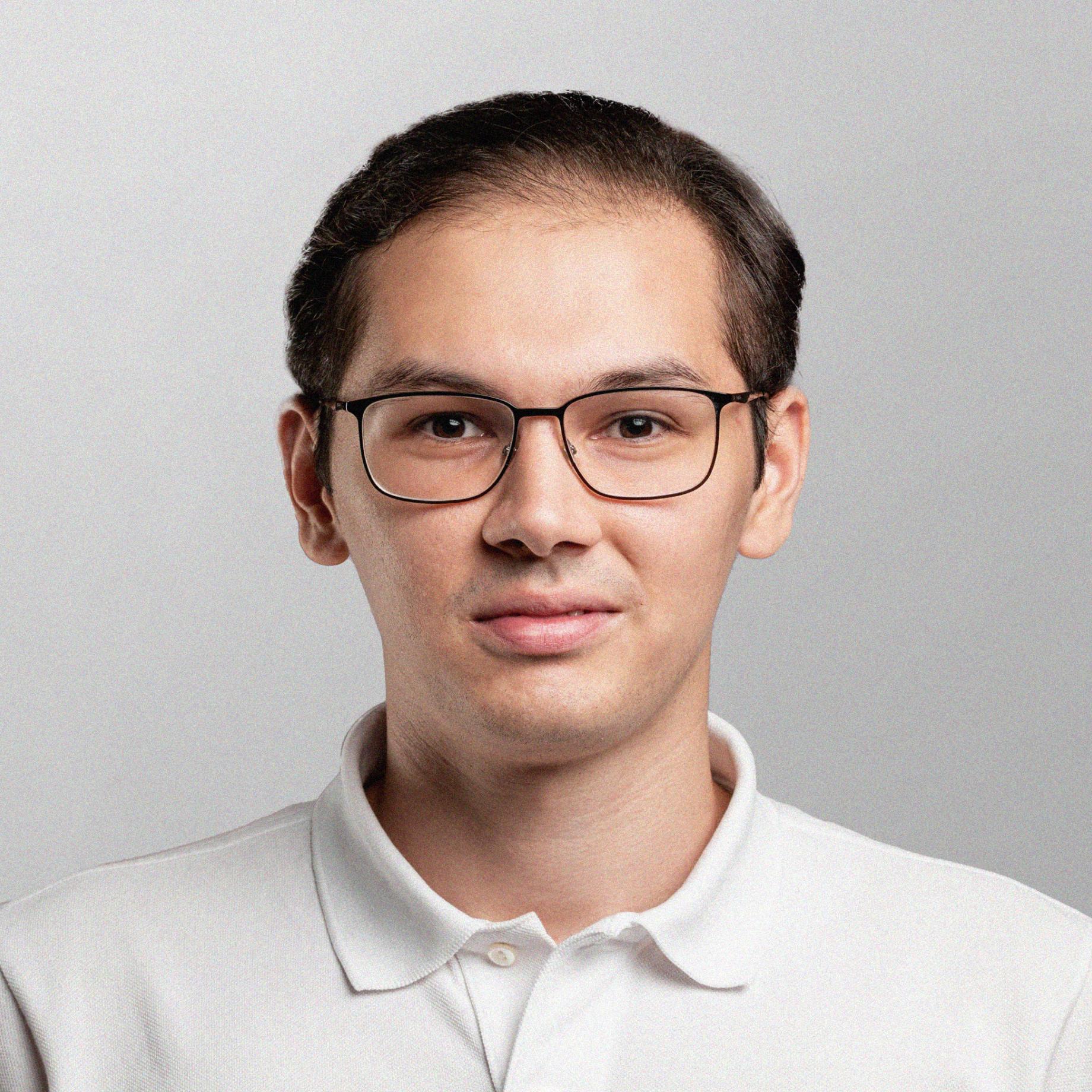
Makhmudjon Sodikov, ML Engineering Manager at Vention
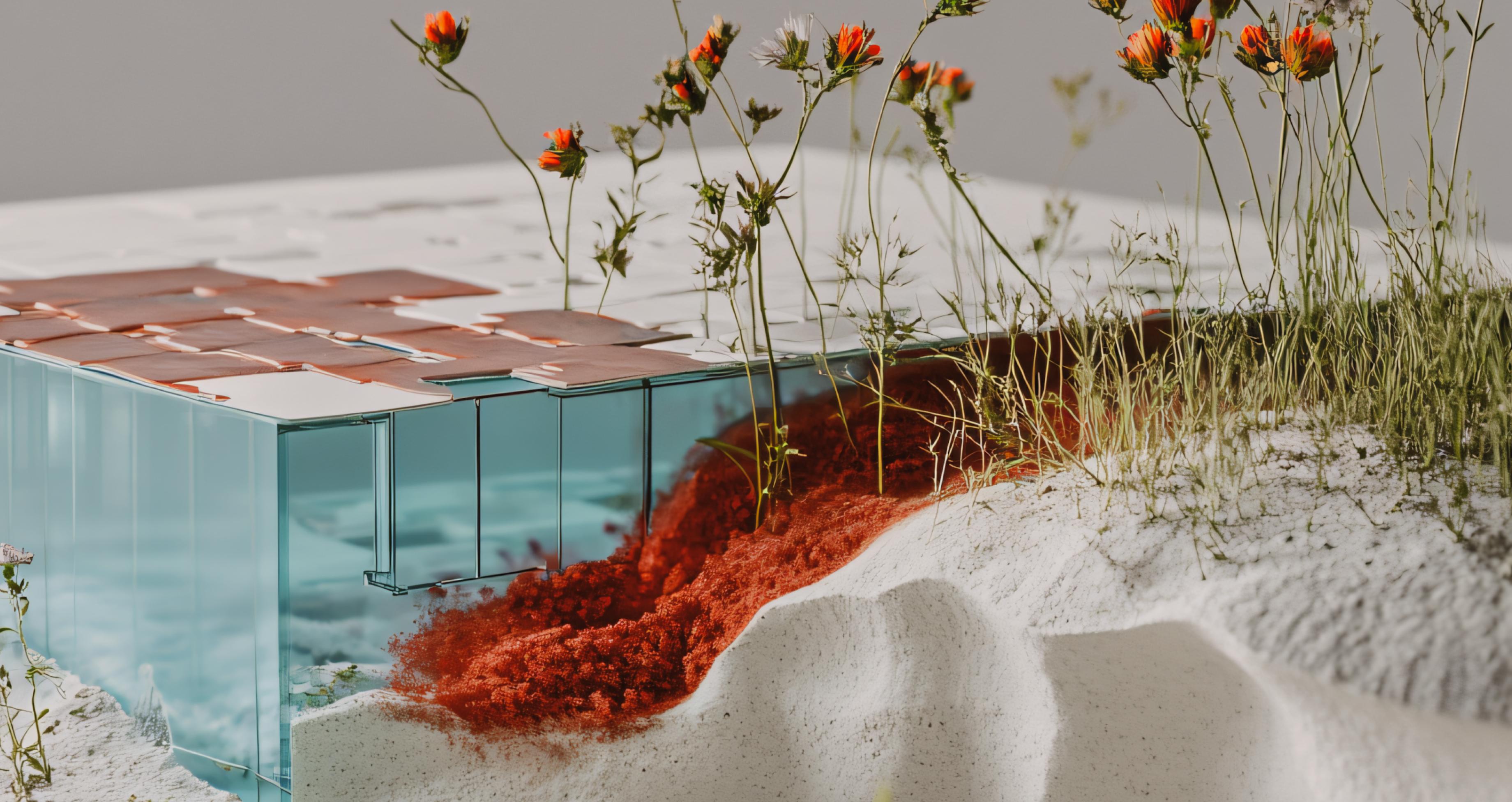
The AI-driven job market is expected to grow by 38% annually from 2024 to 2030 as AI adoption expands across different sectors.
AI-related roles, such as data scientists, machine learning engineers, and AI specialists, are projected to be among the top 10 fastest-growing job categories globally by 2030.
In healthcare alone, AI-driven jobs could rise by 45%, focusing on diagnostics, AI-assisted surgeries, and personalized treatment plans.
By 2030, over 55% of non-tech industries are projected to integrate AI solutions, creating hybrid roles that blend technical skills with industry-specific expertise.
By 2028, 60% of global companies will require employees to have basic AI skills to stay competitive, making upskilling a workforce necessity.
These statistics reveal the profound impact AI will have on the global workforce. But it’s not just about the numbers; the nature of work itself is evolving.
Jobs AI will replace and create
By 2025, AI is expected to replace 16% of jobs globally while creating 9% of new roles — which leads to a net 7% loss in jobs. AI has already directly displaced 3,900 jobs in the US as of 2023.
However, while AI may eliminate some jobs, it will also open the door to new opportunities. By 2027, 69 million jobs are expected to be created, many of them in AI development, ethics, and data curation. And it’s not just about technical roles. Analysts, creatives, and professionals from non-tech backgrounds will all find opportunities in this AI-driven economy. The fastest-growing jobs will be in AI-powered operations management, data analysis, and roles that bridge AI and human collaboration.
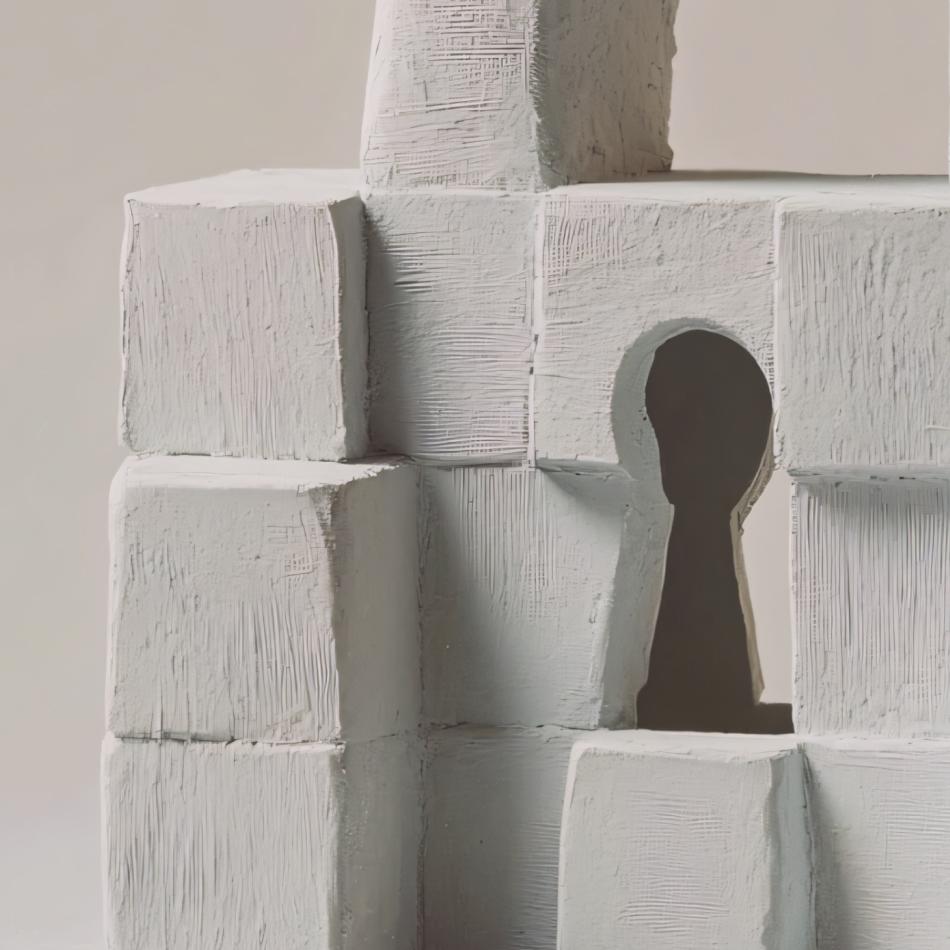
(Translation: Taking an AI course or two today could be the key to securing a future-proof career.)
"For industries beyond tech, AI is more likely to extend jobs rather than replace them. As AI enters sectors that have not yet been tech-enabled, new roles and opportunities will emerge, driving innovation and growth across fields.”
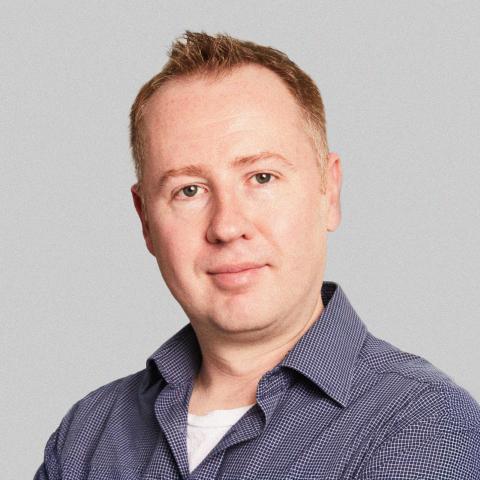
Glyn Roberts, CTO at Vention
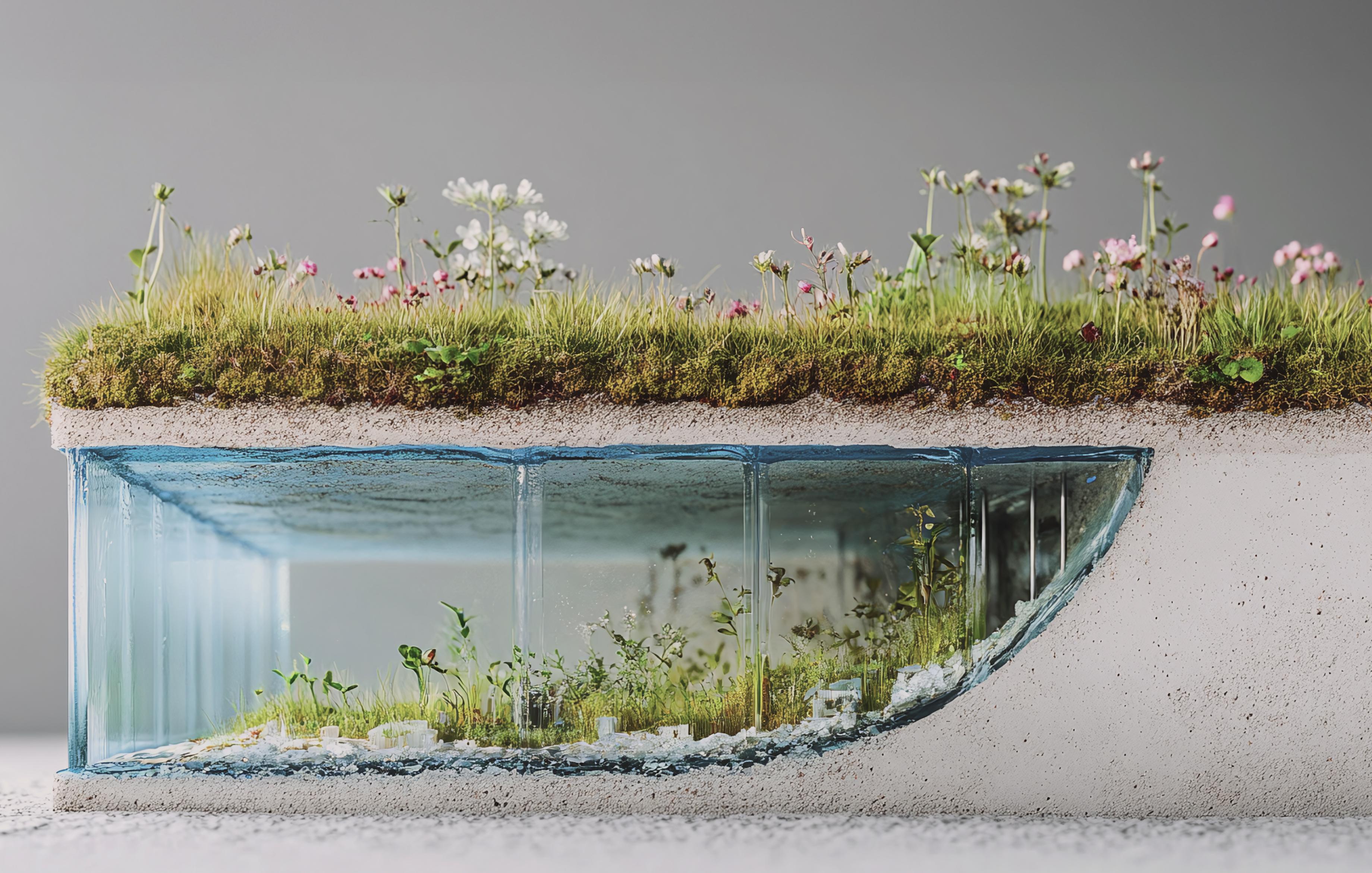
How many AI engineers are there?
In 2017, Tencent stated that there were around 300,000 AI developers while the market demand was at millions. In 2024, the number of AI engineers has grown to approximately 1.2 million, and the demand continues to soar. Estimates suggest that an additional 4 million AI engineers are needed globally to meet current market needs.
India holds nearly 10% of the global AI talent, with notable concentrations of experts in Korea (6.6%), Israel (6.5%), Japan (6.3%), and Germany (3.5%). These AI professionals are working across industries, from tech startups to global giants, and the demand for their expertise spans sectors ranging from healthcare to finance to autonomous systems.
Despite this growth, the gap between supply and demand widens, offering vast opportunities for those looking to enter the field. AI is reshaping the job market, and skilled professionals who can work with AI will be in ever-increasing demand.
AI engineer salaries by region and seniority
With the soaring demand for AI talent, salaries for AI engineers have seen a sharp rise across regions and experience levels. Here's a breakdown of AI engineer salaries based on seniority and location:
Entry level ($)
Mid-level ($)
Senior level ($)
Lead level ($)
United States
Entry level ($)
100,000
Mid-level ($)
140,000
Senior level ($)
180,000
Lead level ($)
220,000
Europe
Entry level ($)
75,000
Mid-level ($)
100,000
Senior level ($)
140,000
Lead level ($)
170,000
Asia
Entry level ($)
50,000
Mid-level ($)
70,000
Senior level ($)
90,000
Lead level ($)
120,000
India
Entry level ($)
30,000
Mid-level ($)
50,000
Senior level ($)
70,000
Lead level ($)
85,000
Canada
Entry level ($)
60,000
Mid-level ($)
85,000
Senior level ($)
110,000
Lead level ($)
135,000
In the United States, lead-level AI engineers earn up to $220,000 annually. At the same time, Asia and India offer lower but rapidly growing salary levels, reflecting the expanding AI job market globally.
These salary trends underline the lucrative opportunities available to AI specialists, reinforcing the importance of acquiring AI-related expertise for career growth and staying competitive in the job market.
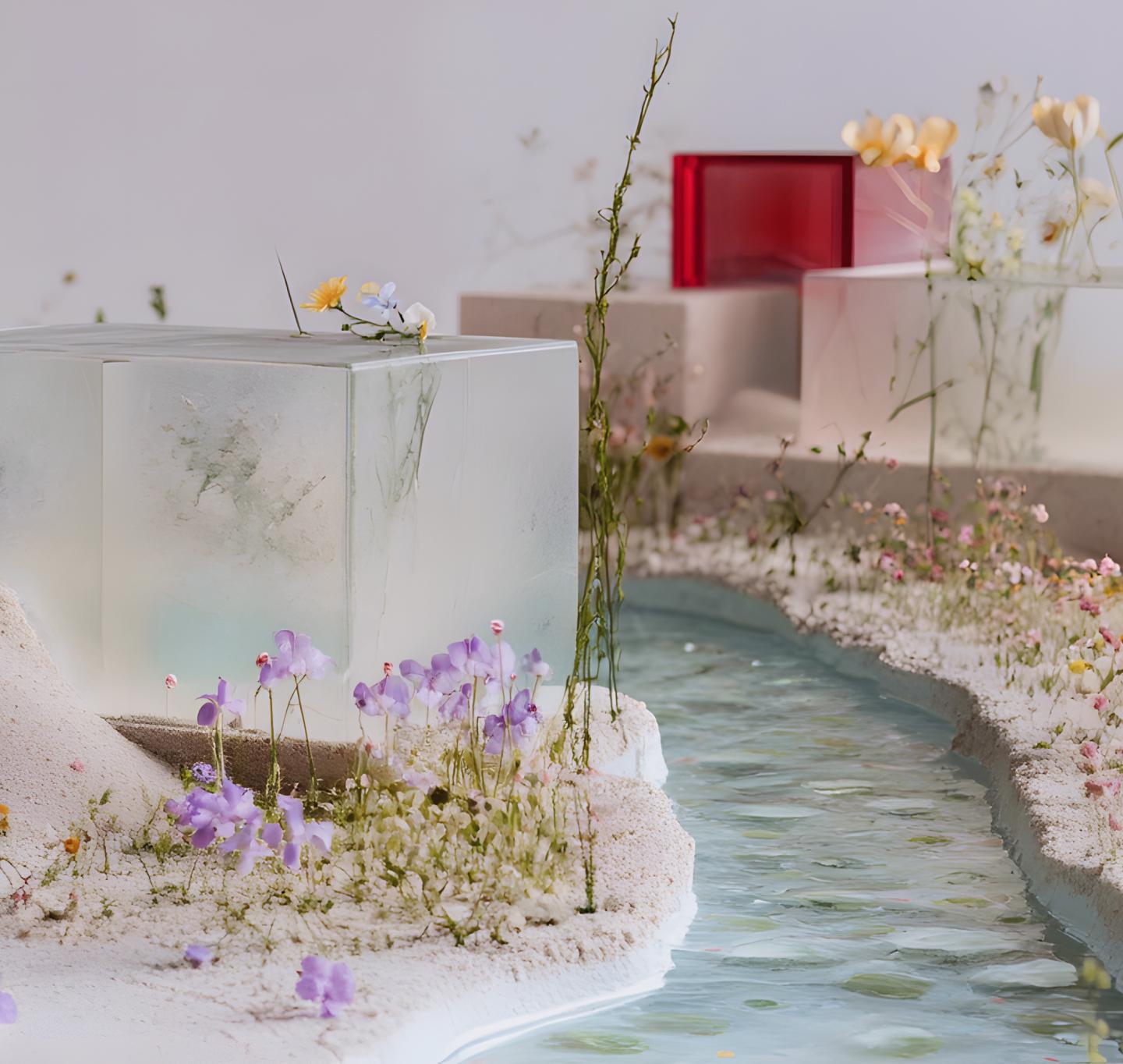
Will AI replace software engineers?
Yes and no. AI can assist in generating code, but it lacks the ability to understand businesses' unique needs or handle real-world complexities. What’s more, AI lacks strategic thinking, which means it still requires human oversight and guidance.
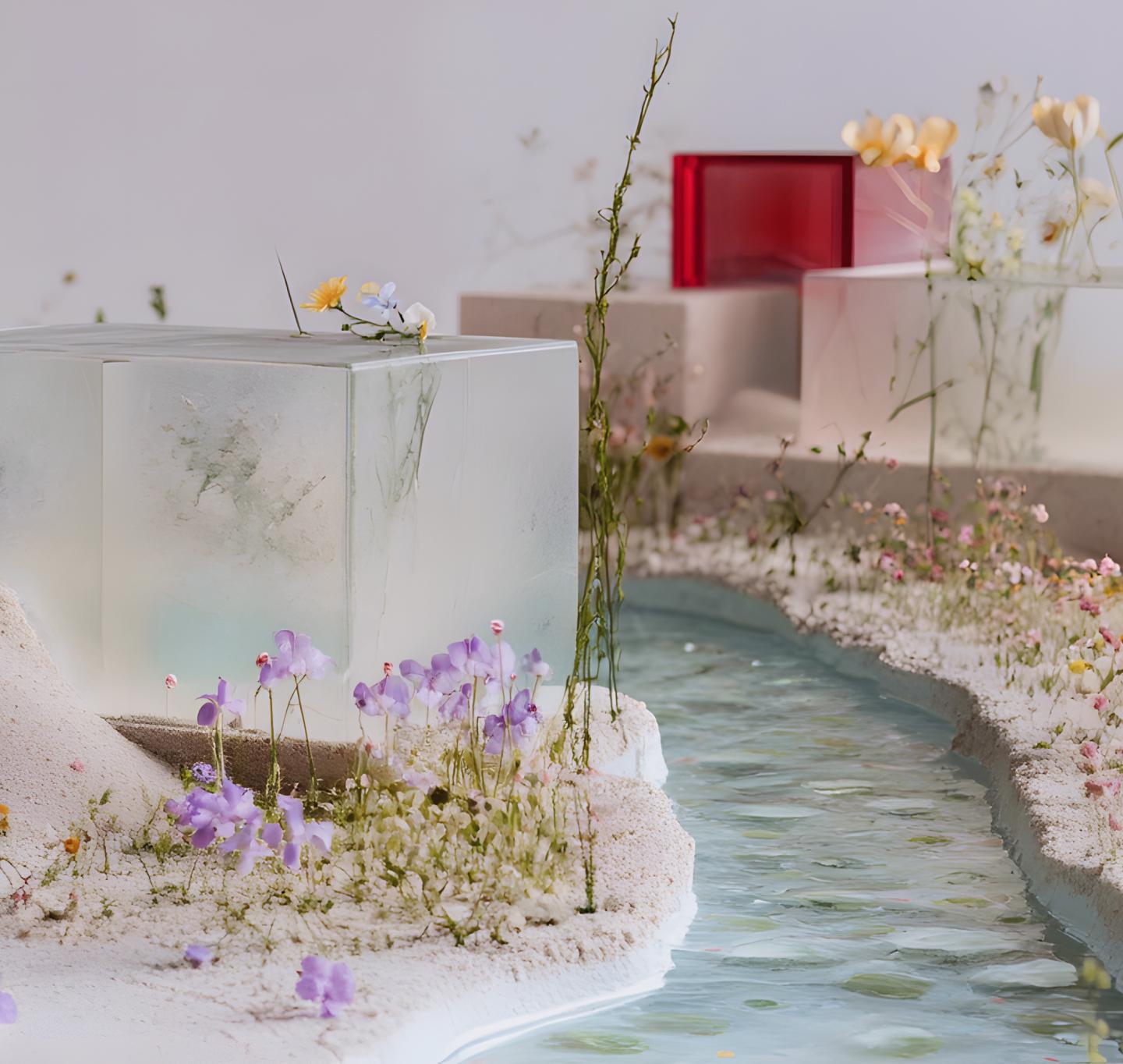
"According to Gartner, by 2028, 75% of enterprise software engineers are expected to use AI code assistants, a massive jump from less than 10% in 2023," notes Glyn Roberts.
This rapid shift means that developers need to sharpen their AI-related skills to remain competitive in an industry evolving at breakneck speed. The future of software engineers will be more about strategy than execution. In this AI-driven landscape, they will become visionaries — guiding, designing, and ensuring that AI is implemented effectively rather than simply writing code.
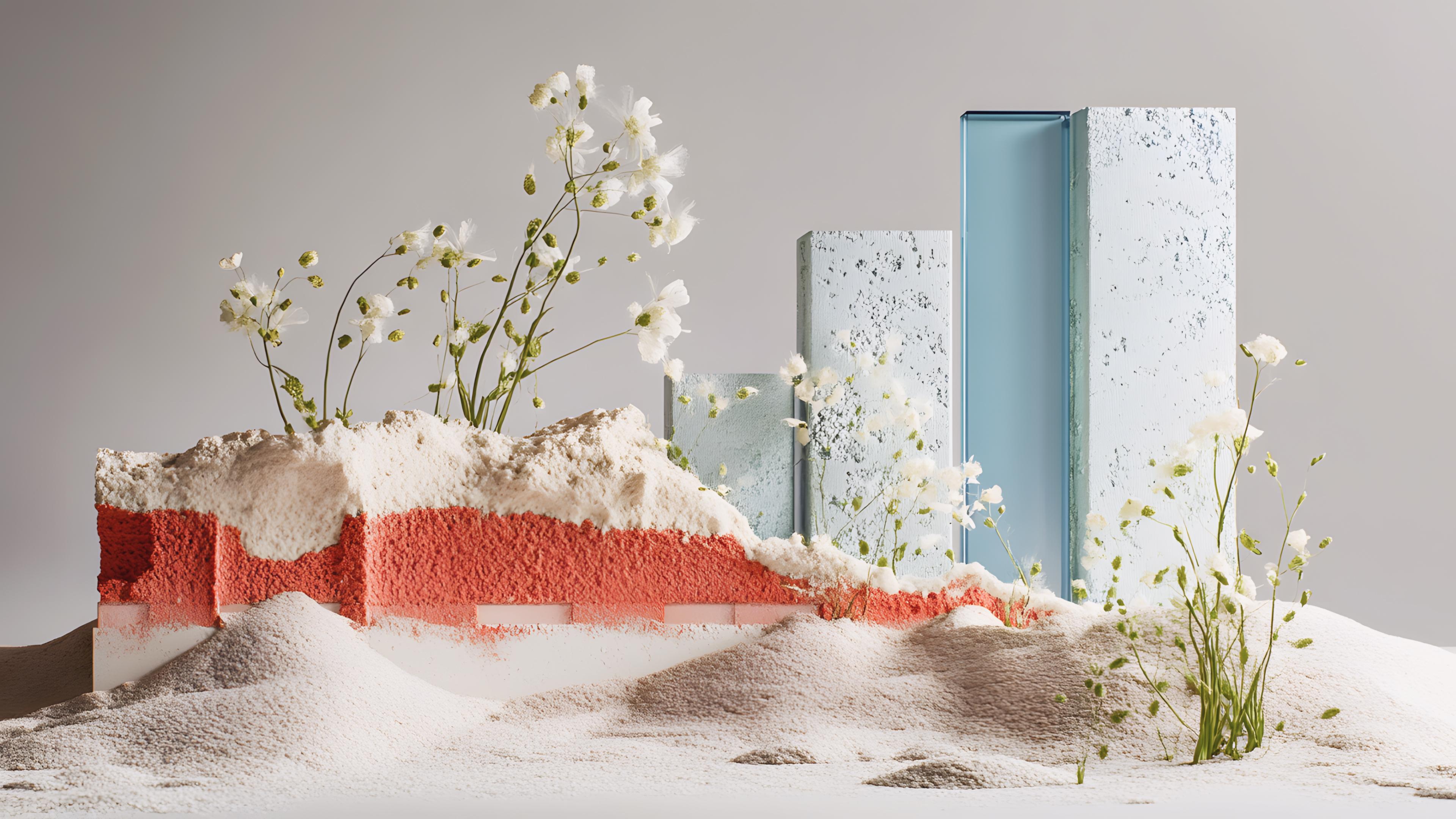
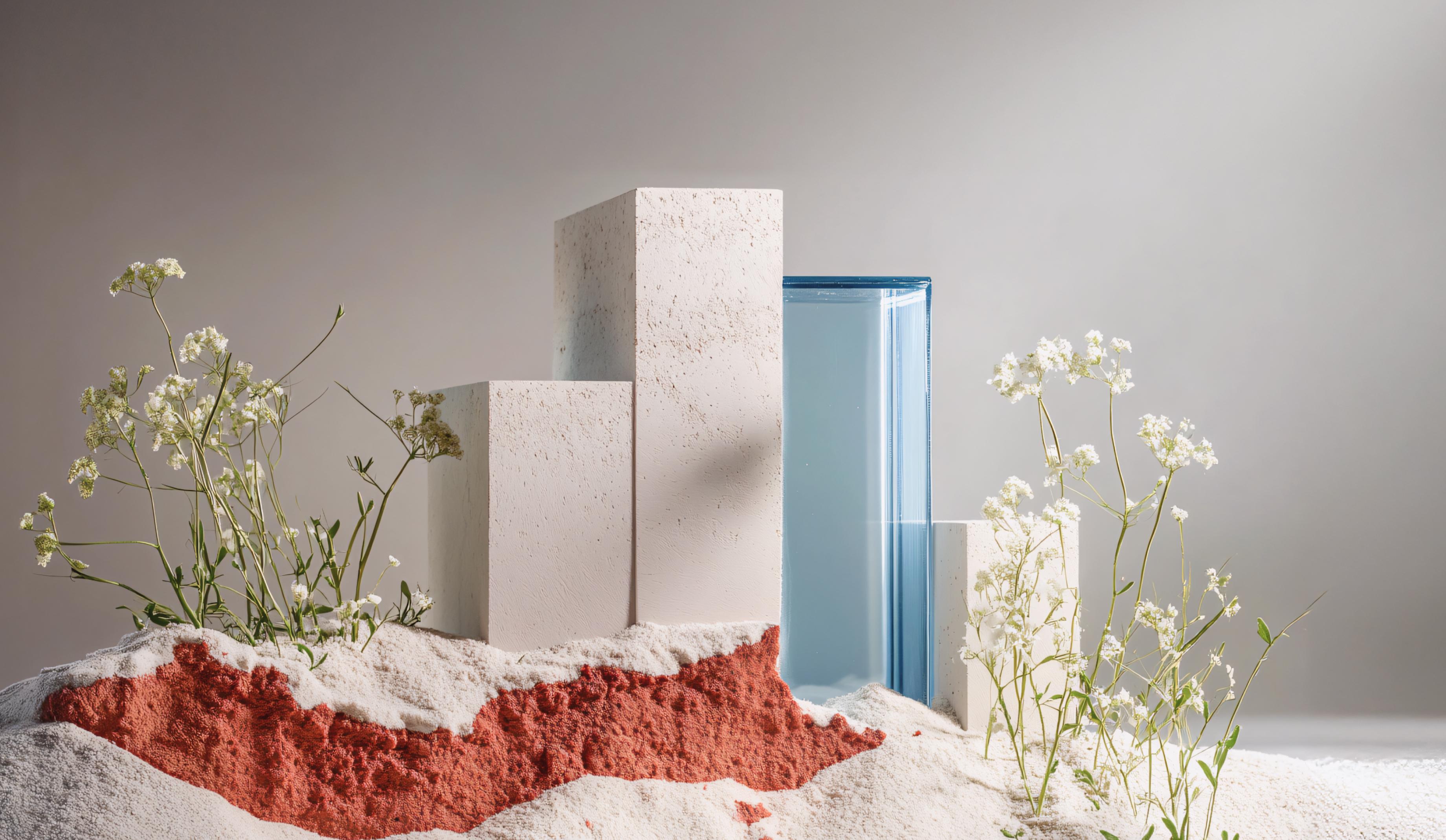
The rapid expansion of AI technology isn’t just evident in projections or investment figures — it’s seen in the surge of AI-powered apps and companies flooding the market. As AI becomes ingrained in core business operations globally, adoption has skyrocketed, with enterprises leveraging its potential for strategic advantage.
But with so many players jumping in, how many are truly leading the revolution?
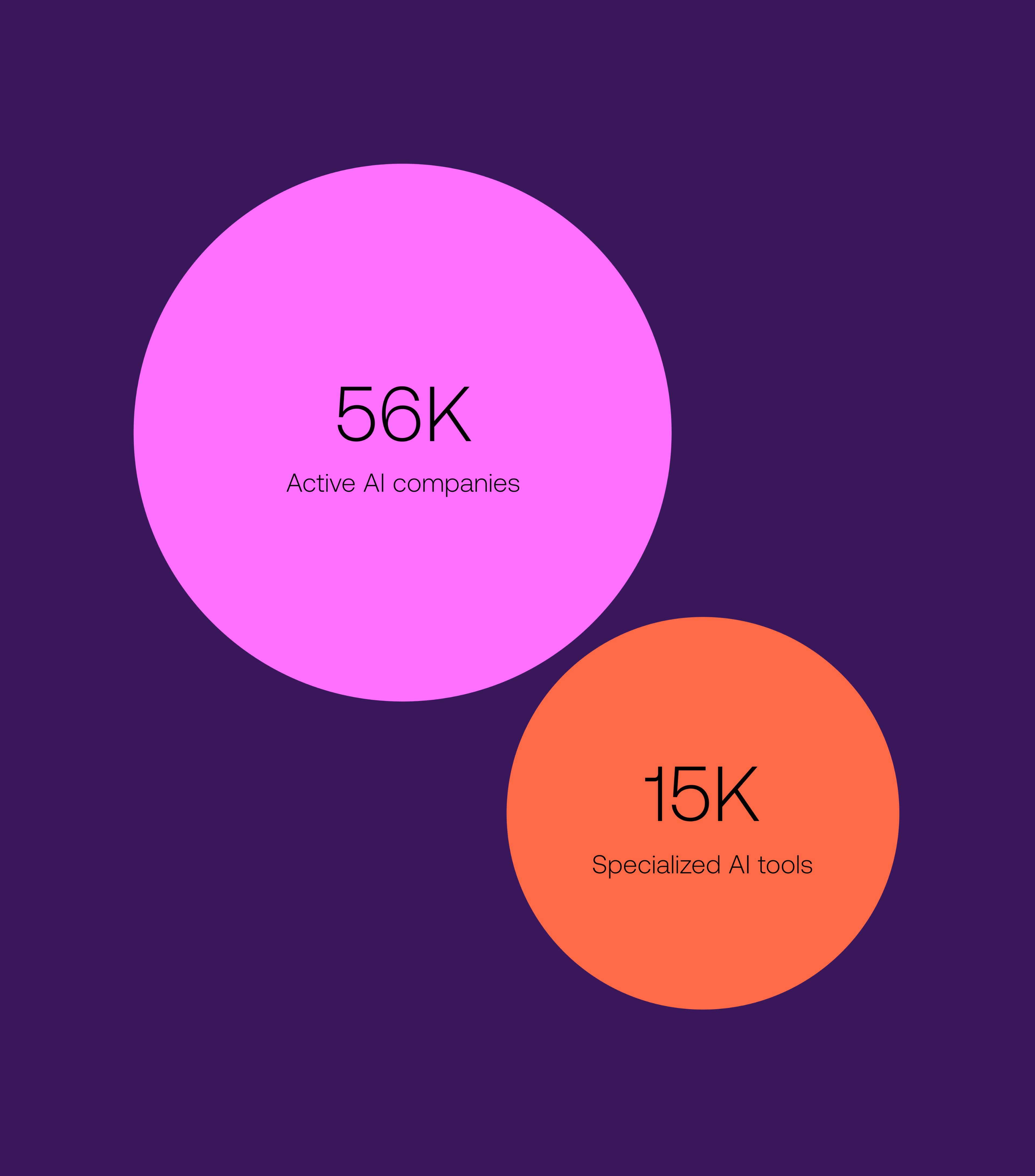
How many AI apps and companies are out there?
As of 2024, there are over 56,000 active AI companies worldwide and 15,000 specialized AI tools designed to automate specific tasks, such as fraud detection, supply chain optimization, and content creation.
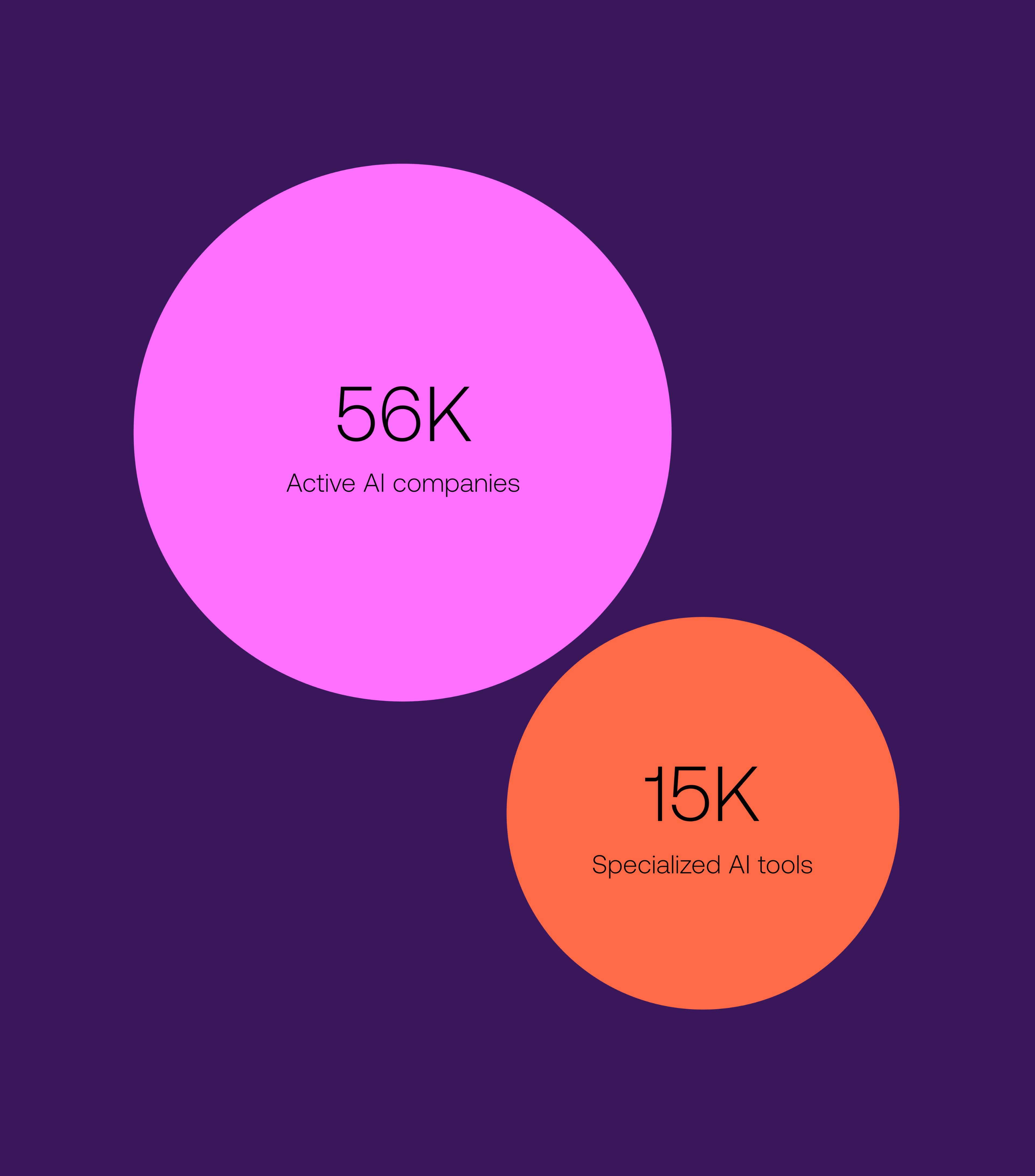
By 2028, the AI ecosystem is projected to expand exponentially, reaching an astounding 38.5 billion AI-powered apps across industries like healthcare, finance, manufacturing, and education. This boom reflects AI’s shift from niche applications to everyday business tools, driving productivity and innovation.
The number of AI companies by HQ region
The geographic distribution of AI companies reveals that the United States is leading with 19,392 firms, followed closely by Europe with 14,518 and Asia with 13,767.
These numbers signal robust global competition, with AI innovation thriving on nearly every continent.
AI usage data and trends
The scale of AI adoption has reached impressive heights. In 2024, 72% of companies globally reported using AI in at least one business function, with 85% of C-suite executives listing AI as a critical driver of their growth strategies.
In the US, 25% of companies are already leveraging AI at scale, while another 43% are in exploratory stages, preparing to integrate AI solutions across their operations.
Furthermore, 65% of companies globally use generative AI, highlighting the widespread acceptance of tools like natural language models and creative AI systems that drive content generation, marketing campaigns, and design processes.
AI and GenAI adoption across organizations, %
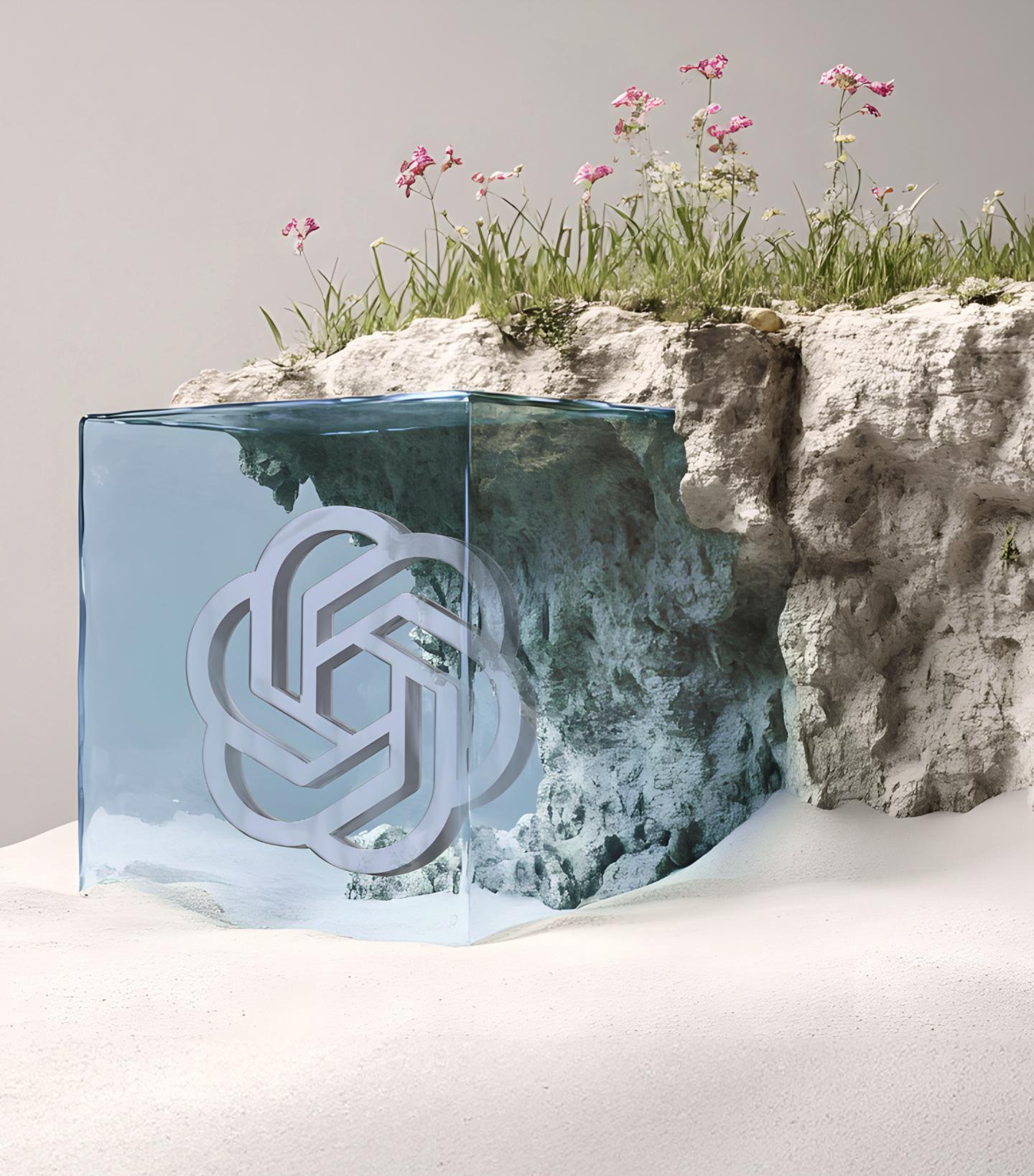
Most popular AI companies, apps, and tools
AI dominance is driven by several key players who have consistently pushed the boundaries of innovation. In 2024, ChatGPT by OpenAI leads the charge, holding over 60% of the market in AI-based conversational tools.
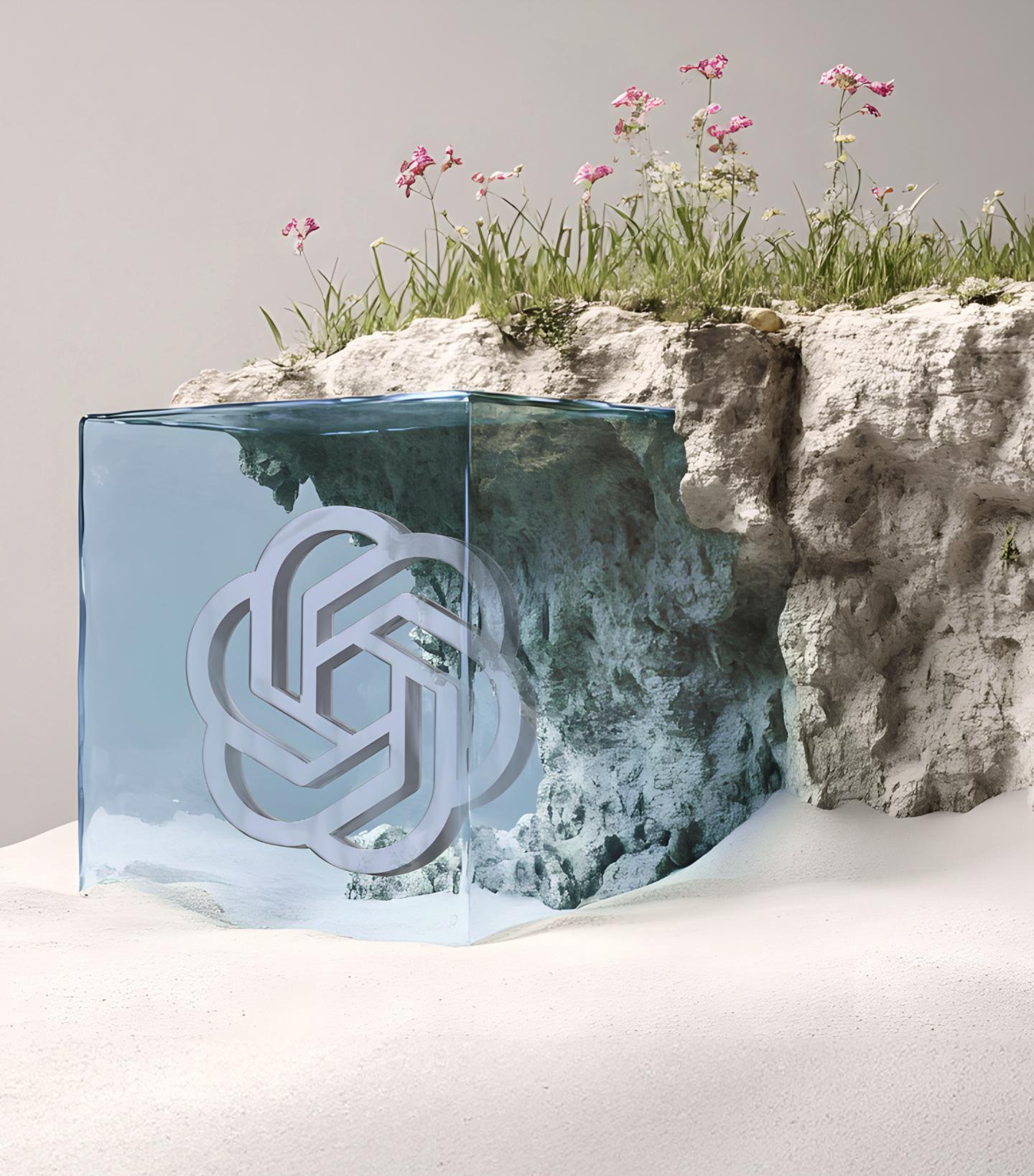
Its impact goes far beyond chatbots — ChatGPT has revolutionized how businesses manage customer interactions, automate support, and generate creative content.
With over 1.6 billion interactions recorded monthly, OpenAI's models have set new benchmarks for AI integration across industries.
Other titans are shaping the AI landscape just as dramatically:
-
Google with Bard dominates the search engine and assistant space, processing over 6 billion queries through AI-based search algorithms in 2024. Bard’s integration into everyday tools has redefined the search experience, enabling AI-powered hyper-personalized results.
-
Microsoft with Copilot, integrated into Office 365, has enabled over 350 million users to automate workflows, from generating reports to writing code.
-
Amazon, with its AI-powered cloud services, controls 55% of all AI workloads in the cloud computing market, offering scalable and adaptable AI solutions.
Then there’s Netflix, a standout example of how AI has transcended traditional tech industries. While most know Netflix for its AI-driven content recommendations, its use of AI goes much deeper.
In 2024, a staggering 87% of Netflix’s content decisions were driven by AI-powered data analytics. This helps predict which shows and movies will resonate with audiences and shapes content production strategies from the ground up, allowing Netflix to forecast trends and even greenlight projects with precision.
“We are witnessing a remarkable increase in both the output and quality of AI-driven solutions. AI is no longer an isolated tool but is becoming seamlessly embedded into everyday applications.
While some users may need to adopt new tools to leverage AI's full potential, for many, it will be softly integrated into the platforms they already use, enhancing productivity without disrupting workflows.”
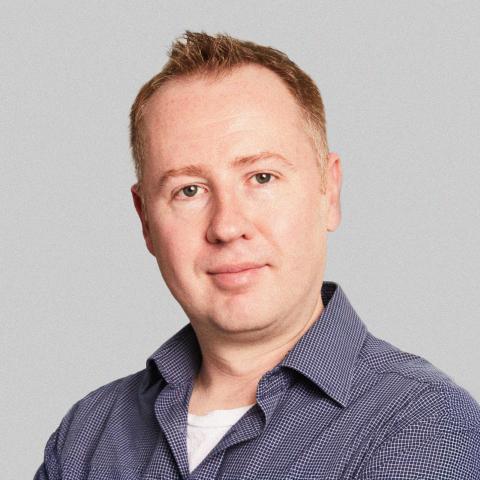
Glyn Roberts, CTO at Vention
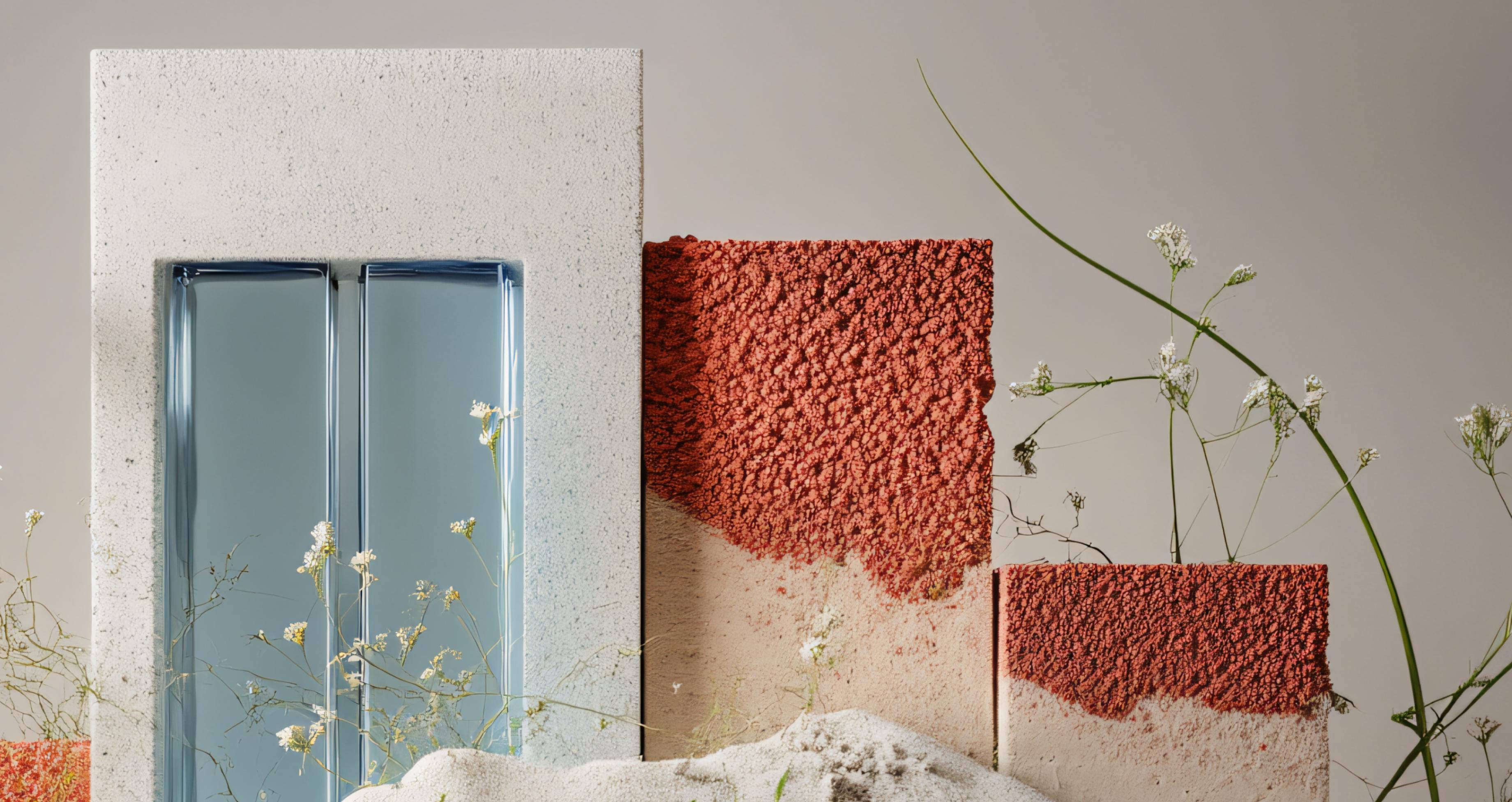
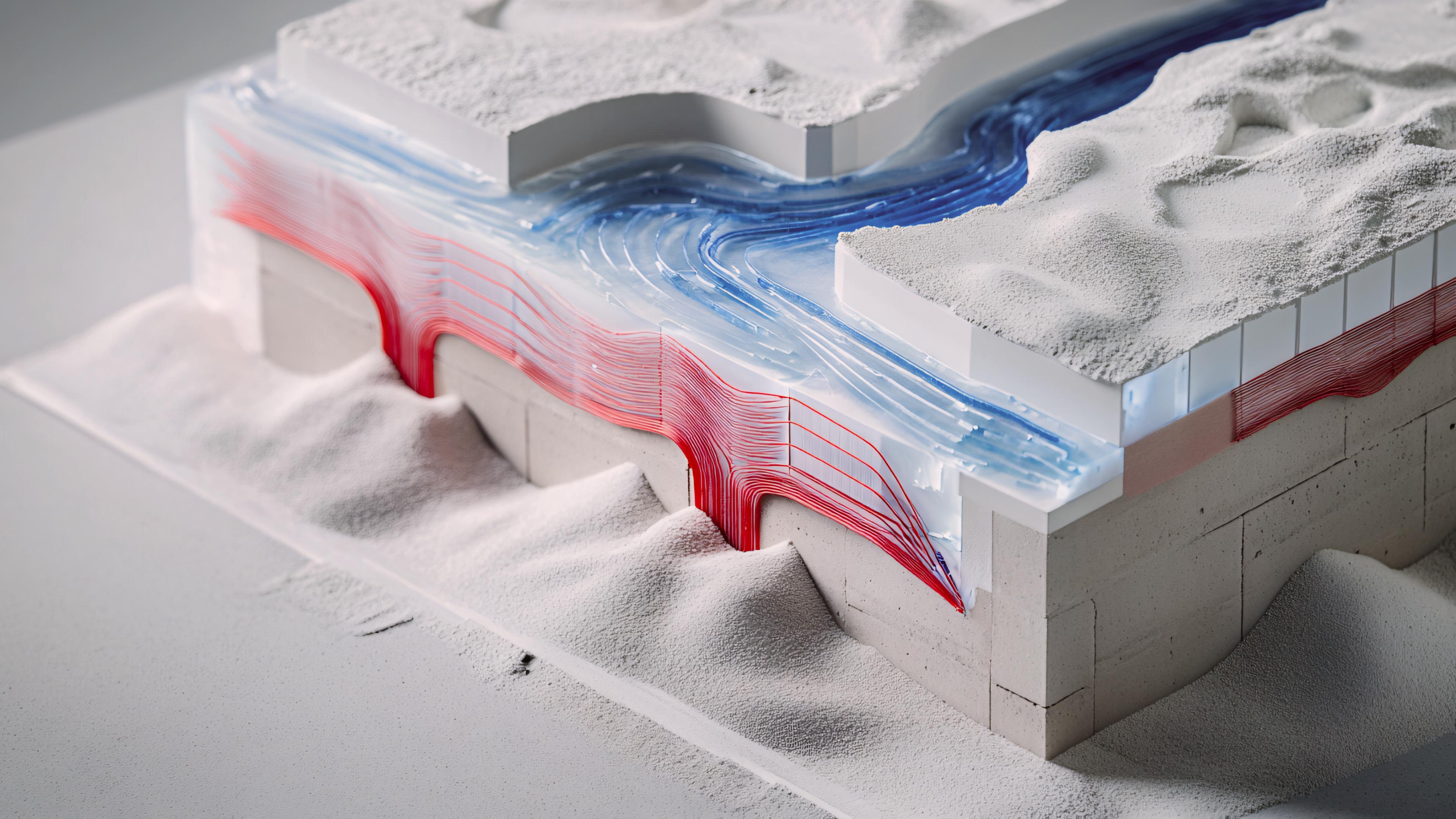
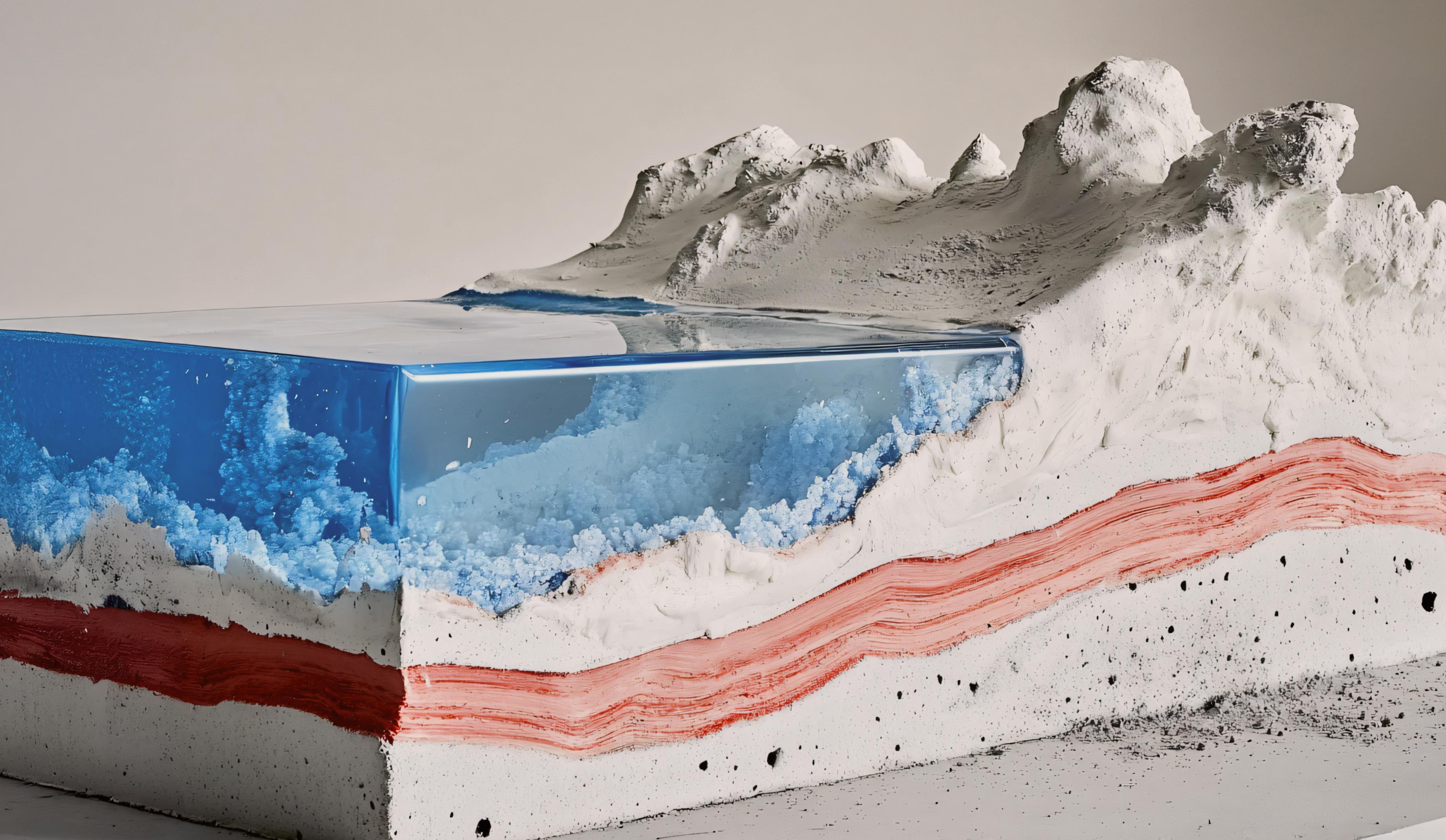
The rapid advancements in AI solutions have led to a predictable outcome: AI adoption rates are soaring across industries. Whether it's optimizing workflows or transforming customer interactions, AI has quickly become a vital asset for organizations aiming to innovate and lead in their fields.
AI's role in boosting productivity
In a 2023 IBM survey, 64% of businesses reported that AI significantly improved their overall productivity, with tangible benefits ranging from workflow automation to enhanced customer support.
G2 data reveals that in 2024, 83% of organizations implementing AI platforms saw a positive ROI within just 3 months. These figures highlight AI’s immediate impact on business efficiency and bottom-line growth.
Additionally, businesses that adopt AI are projected to increase their profitability by 38% in 2025.
Key areas where AI boosts productivity:
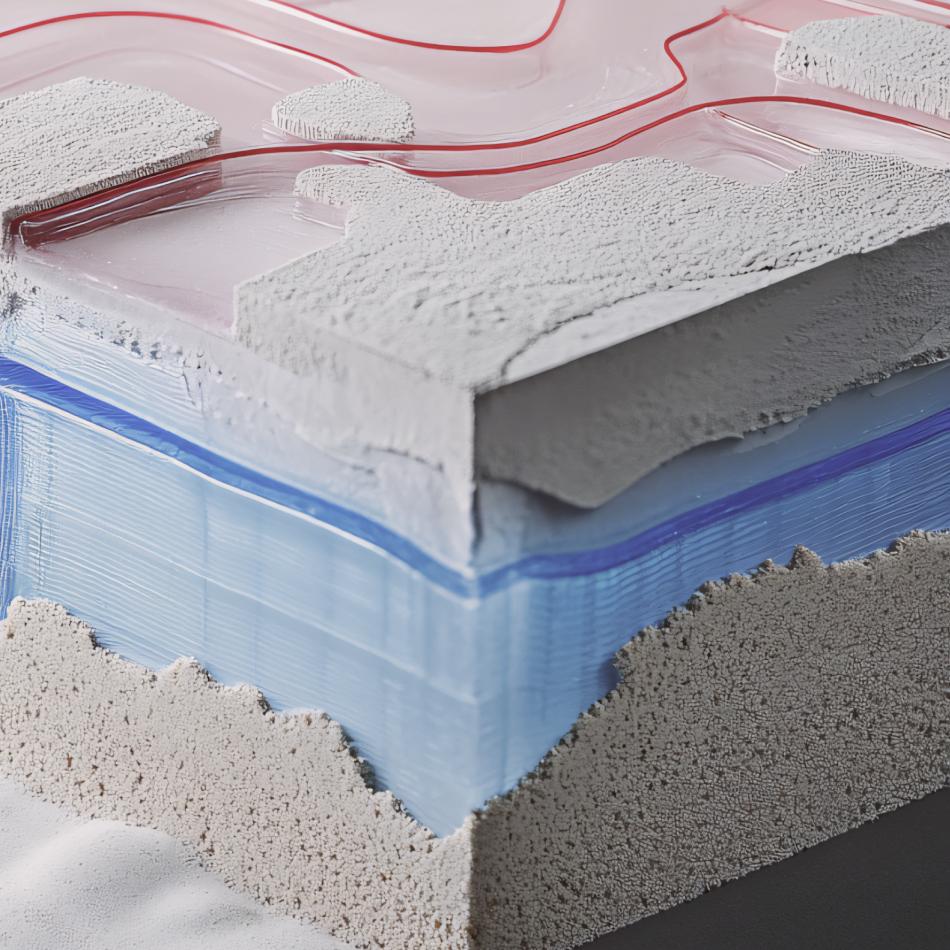
Automating repetitive tasks in customer support and HR — a practice estimated to save businesses over $80B annually by 2025
Leveraging AI-powered predictive analytics in marketing and sales — a market expected to reach $30B by 2025
Optimizing supply chains in retail and manufacturing, with AI expected to save businesses $2T annually in operational costs by 2030
“AI is empowering organizations to make better, more informed decisions by leveraging new data sources that were previously overlooked. This expanded access to insights enables businesses to refine their strategies and operations with unprecedented precision and foresight.”
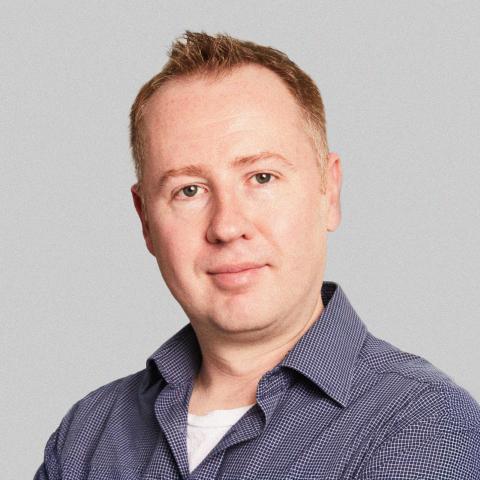
Glyn Roberts, CTO at Vention
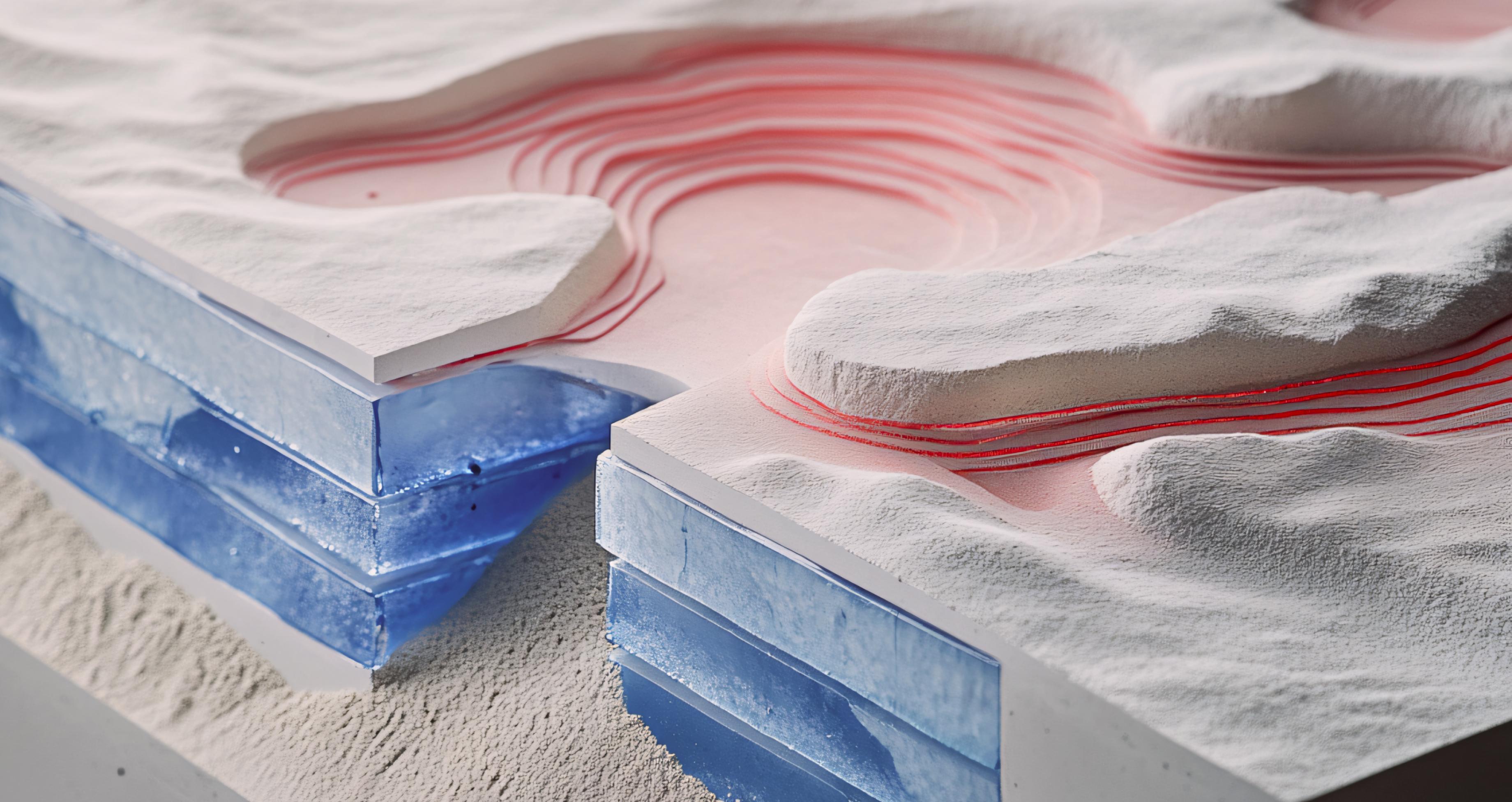
Financial services
Automotive
Retail
Entertainment
Manufacturing
Energy
Transport and logistics
Personalized financial services: 71% of customers prefer AI-powered interactions in banking.
Advanced fraud detection systems are expected to prevent $26B in fraud losses globally by 2026.
Complex process automation beyond back-office functions could save the financial industry $400B by 2030.
The global market for autonomous vehicles is projected to reach $1.64T by 2030.
Semi-autonomous features are now integrated into 75% of new vehicles produced globally.
Personalized design and production: AI-driven design tools can reduce production times by 30%.
Predictive analytics for customer demand are anticipated to increase profit margins in the retail sector by 10-15% by 2025.
AI-enhanced inventory and delivery management usually enhance efficiency by 20-30% across supply chains.
Improved content recommendation systems: Streaming services typically see 40% of user engagement driven by AI-powered recommendations.
Customized content creation: By 2027, 80% of all marketing content is projected to be generated by AI.
Personalized marketing: AI-powered marketing campaigns typically increase average conversion rates by 35%.
AI-driven monitoring and auto-correction improve production efficiency by 25%.
Optimized supply chains are set to save the manufacturing sector $1T annually by 2030.
AI-powered smart grids could improve energy distribution efficiency by 15-20%.
AI-driven predictive maintenance is projected to save the energy sector $1.1B annually by 2028.
Autonomous trucking and delivery is estimated to grow into a $166B market by 2030.
AI-enhanced traffic control systems could reduce urban congestion by 30%.
Security improvements powered by AI analytics are forecasted to reduce transportation incidents by 40% by 2027.
AI in everyday life: beyond ChatGPT and self-driving cars
While much of the AI conversation centers around popular tools like ChatGPT or autonomous vehicles, AI’s reach extends far beyond these headline-grabbers.
Take Google Maps, for instance — its advanced AI algorithms help 1 billion users navigate the world monthly. Many industries quietly leverage AI, transforming their core processes and services in game-changing ways.
In human resources, AI helps automate routine tasks such as candidate sourcing, onboarding, and ongoing HR support, leading to:
- Enhanced hiring efficiency, reducing time-to-hire by 45%
- AI-powered onboarding systems improving employee retention by 20%
- AI-powered training programs cutting corporate training costs by 30%
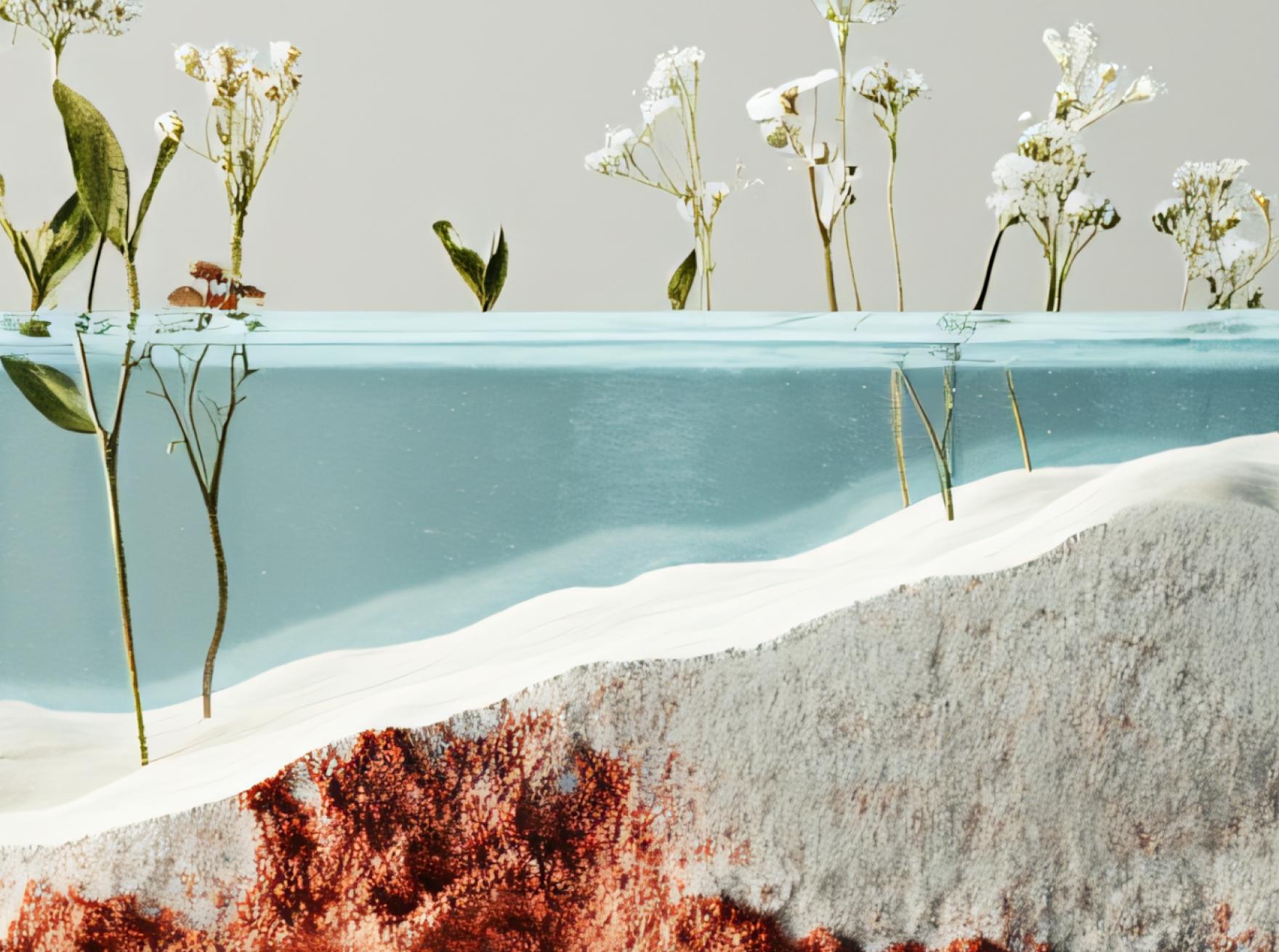
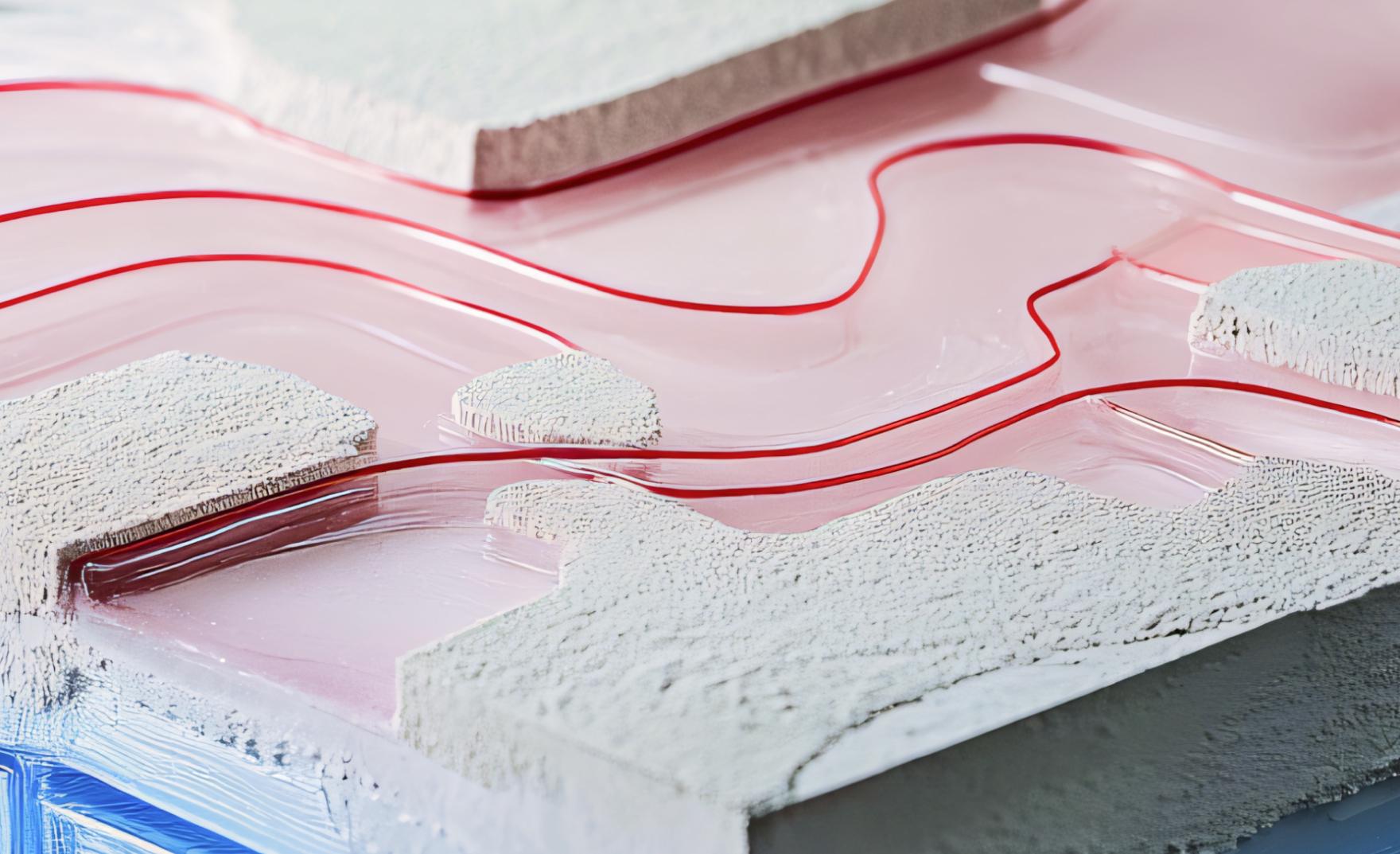
AI is transforming healthcare as a powerful assistant for medical professionals and researchers.
With AI, patient interactions evolve from lengthy procedures to efficient, responsive chatbots, while administrative tasks like appointment scheduling and billing are seamlessly automated.
More critically, AI enhances diagnosis accuracy by analyzing patient data against vast datasets and recommending personalized treatment plans based on a patient's history. For instance, AI can reduce diagnostic times by 25% and improve patient outcomes by 30%.
In research, AI predicts the interactions of new medical compounds, expediting drug discovery by 30-40%, potentially saving $500M per drug in development costs.
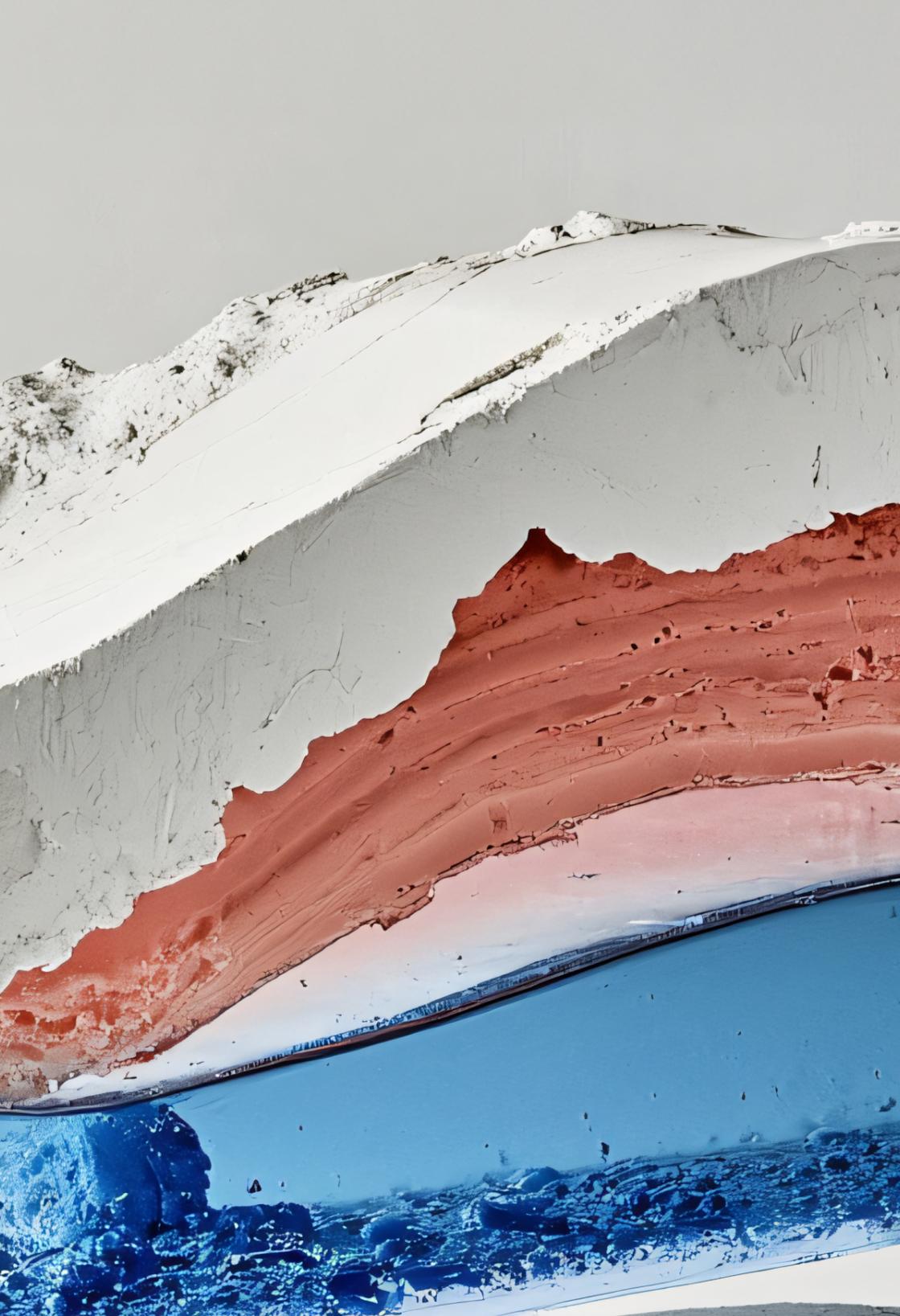
AI is transforming the education landscape by delivering personalized learning experiences that adapt to individual student needs.
With AI:
-
Students can access tailored learning tracks, leading to a 20% improvement in student performance
-
Smart grading systems can reduce administrative burdens on educators by 50%
-
Automated feedback mechanisms increase learning engagement by 35% and provide real-time support to students
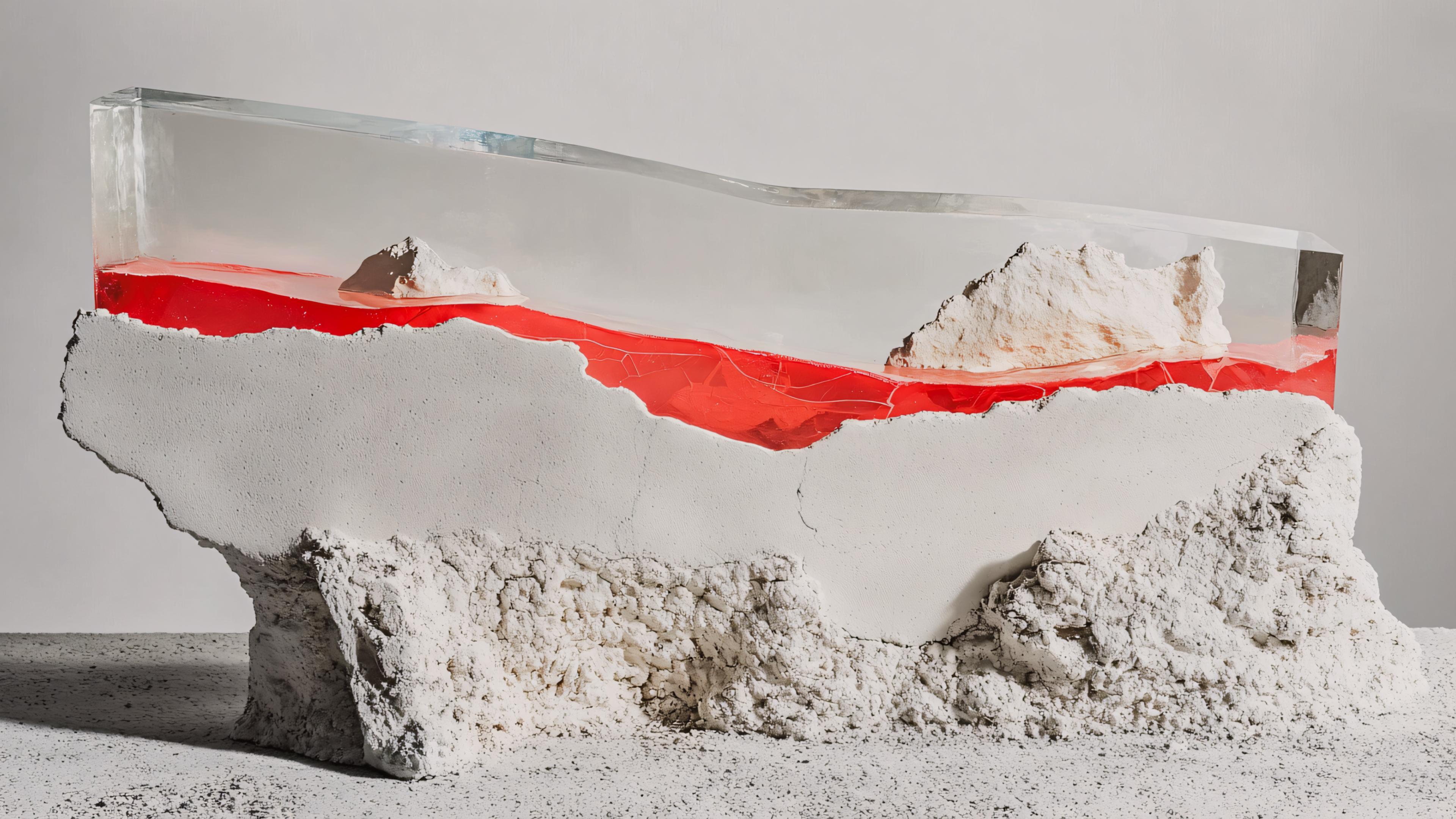
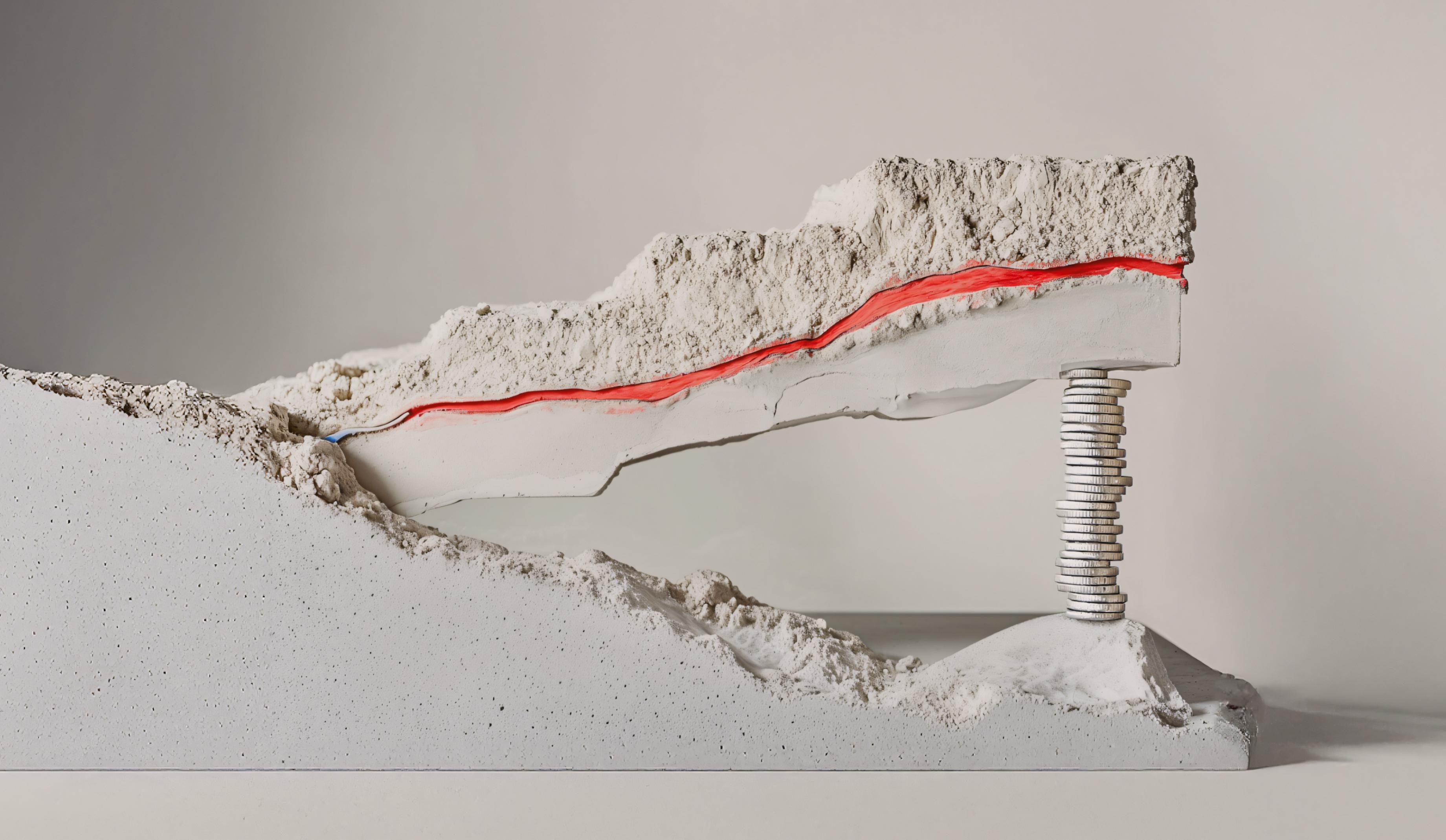
AI adoption is reshaping industries, but it comes at a cost. Developing and maintaining AI applications is often pricier than traditional software development due to the critical need for data in training models, advanced infrastructure, and added steps like data preparation and model optimization.
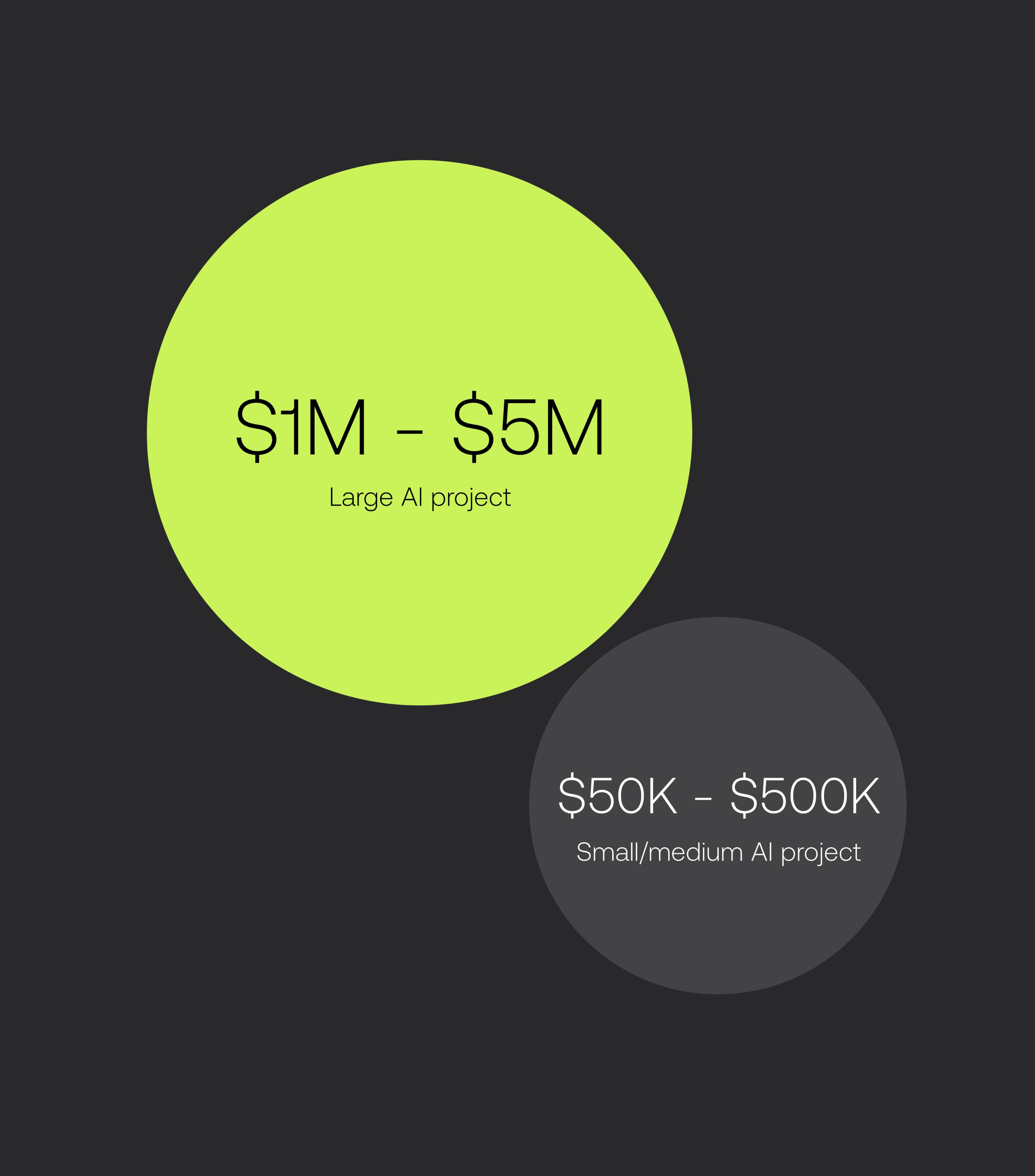
AI software development costs
Small to medium AI projects usually cost between $50,000 and $500,000, while large-scale initiatives can exceed $5M.
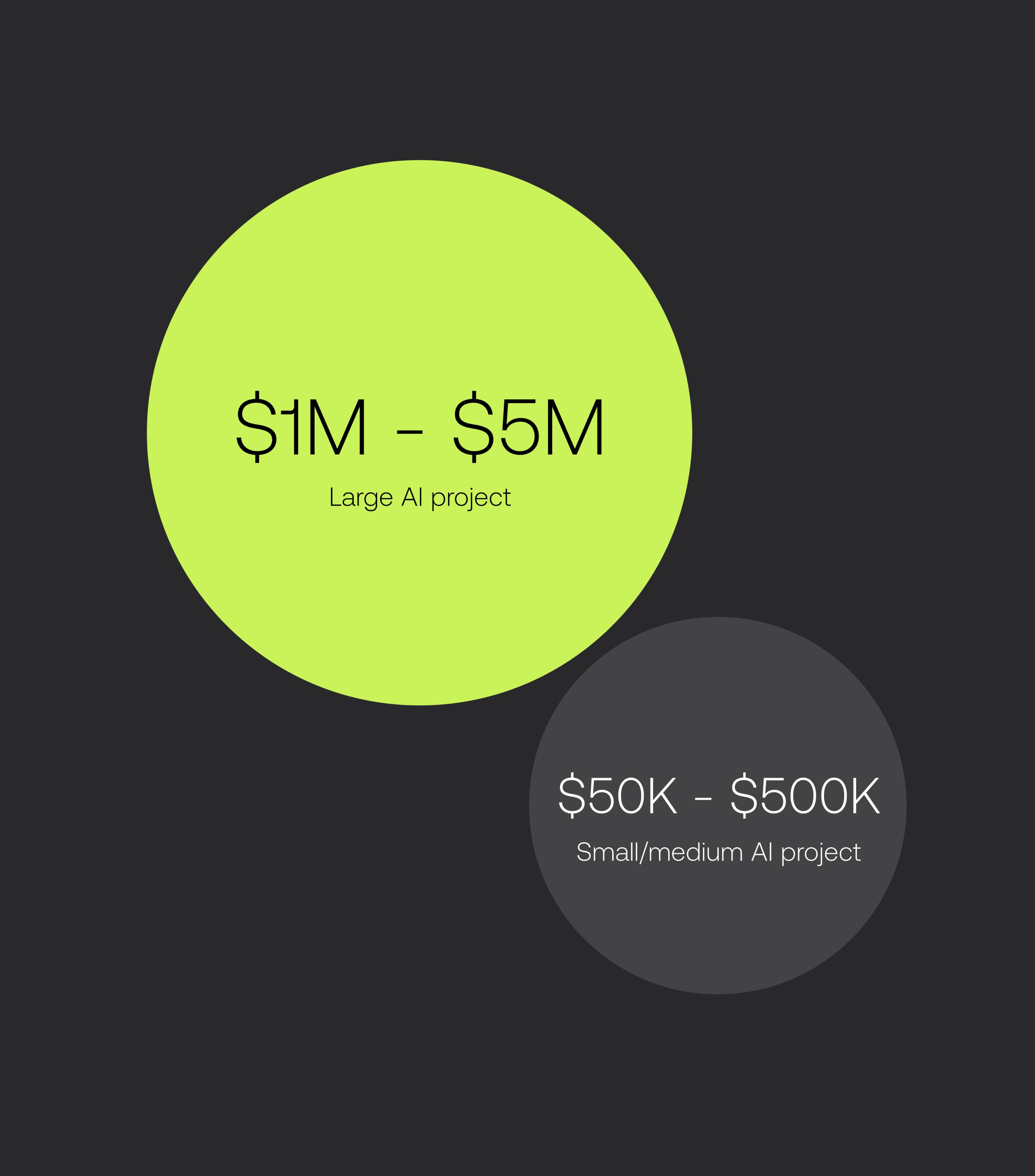
But what drives these costs? Factors like extensive data collection and processing, specialized infrastructure, and additional steps such as data preparation and model optimization all contribute to the overall expense.
The final price depends on the complexity of the solution, the user base, and whether existing AI models are integrated or custom-built from scratch. Example: integrating a GPT model into a chatbot is more affordable than creating a custom large language model (LLM) for your specific needs.
Despite the investment, AI solutions often deliver significant returns through revenue growth from premium subscriptions, partnerships, and increased investment opportunities. Companies investing in AI solutions typically see a 39% revenue boost and a 45% increase in customer retention rates.
The risks of AI
These AI costs also include managing the risks associated with AI. AI projects face challenges similar to those of traditional software development, such as data security and privacy concerns. However, AI-specific risks can introduce additional expenses, including inaccuracies in model predictions and potential intellectual property violations.
A lack of oversight can lead to significant financial repercussions. Between 2022 and 2023, AI-related incidents increased by 1,278%, as reported by the OECD, which affected industries like finance, healthcare, and manufacturing.
These incidents, often linked to AI model failures or data breaches, highlight the importance of comprehensive risk management strategies.
Number of AI incidents from January 2021 to December 2024
Q1/21
Q2/21
Q3/21
Q4/21
Q1/22
Q2/22
Q3/22
Q4/22
Q1/23
Q2/23
Q3/23
Q4/23
Q1/24
Q2/24
Q3/24
Q4/24
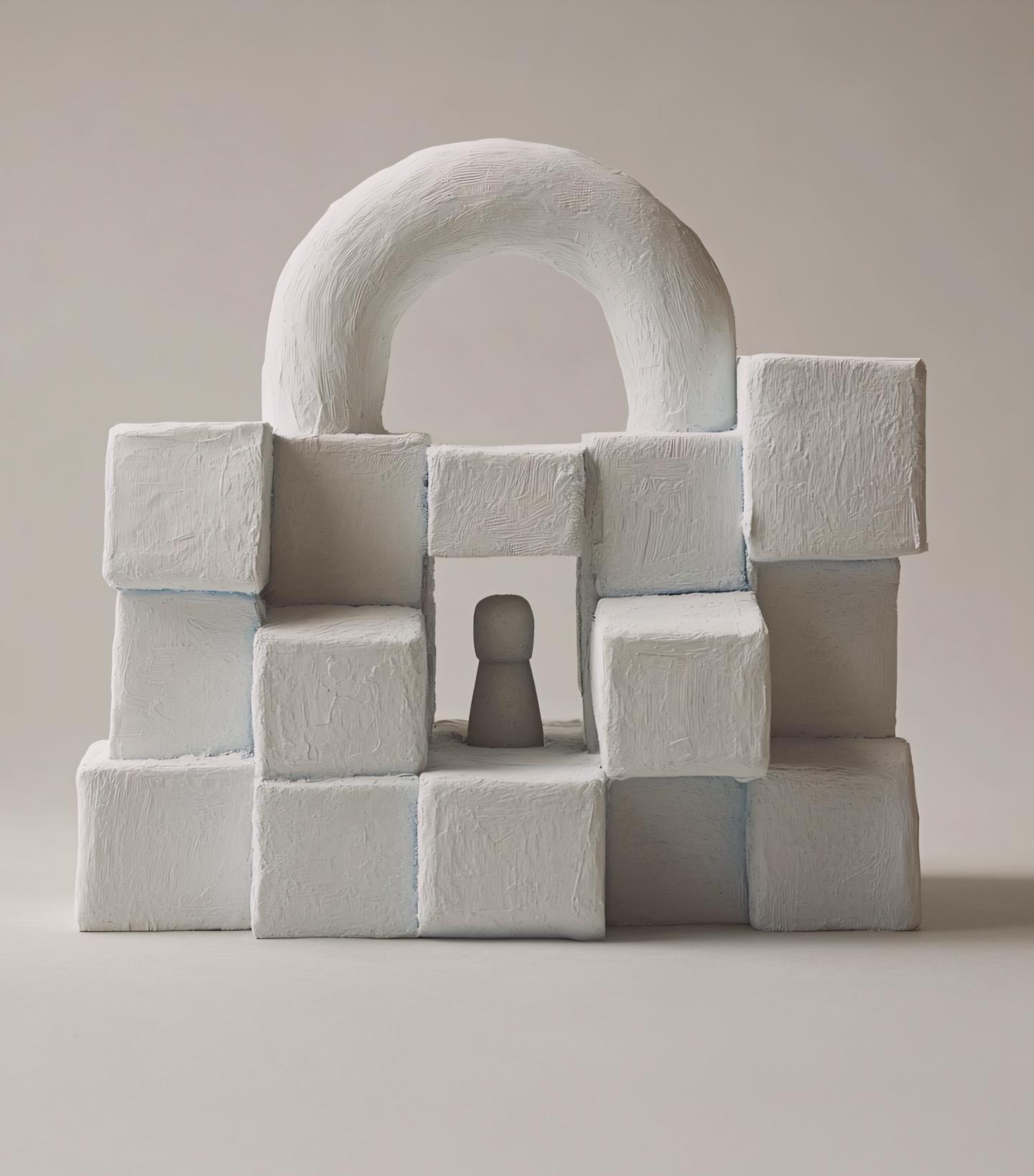
AI and security breaches
Security breaches in AI systems represent one of the most costly risks. In 2023, 77% of businesses reported a breach involving their AI systems, highlighting the critical need for enhanced security measures.
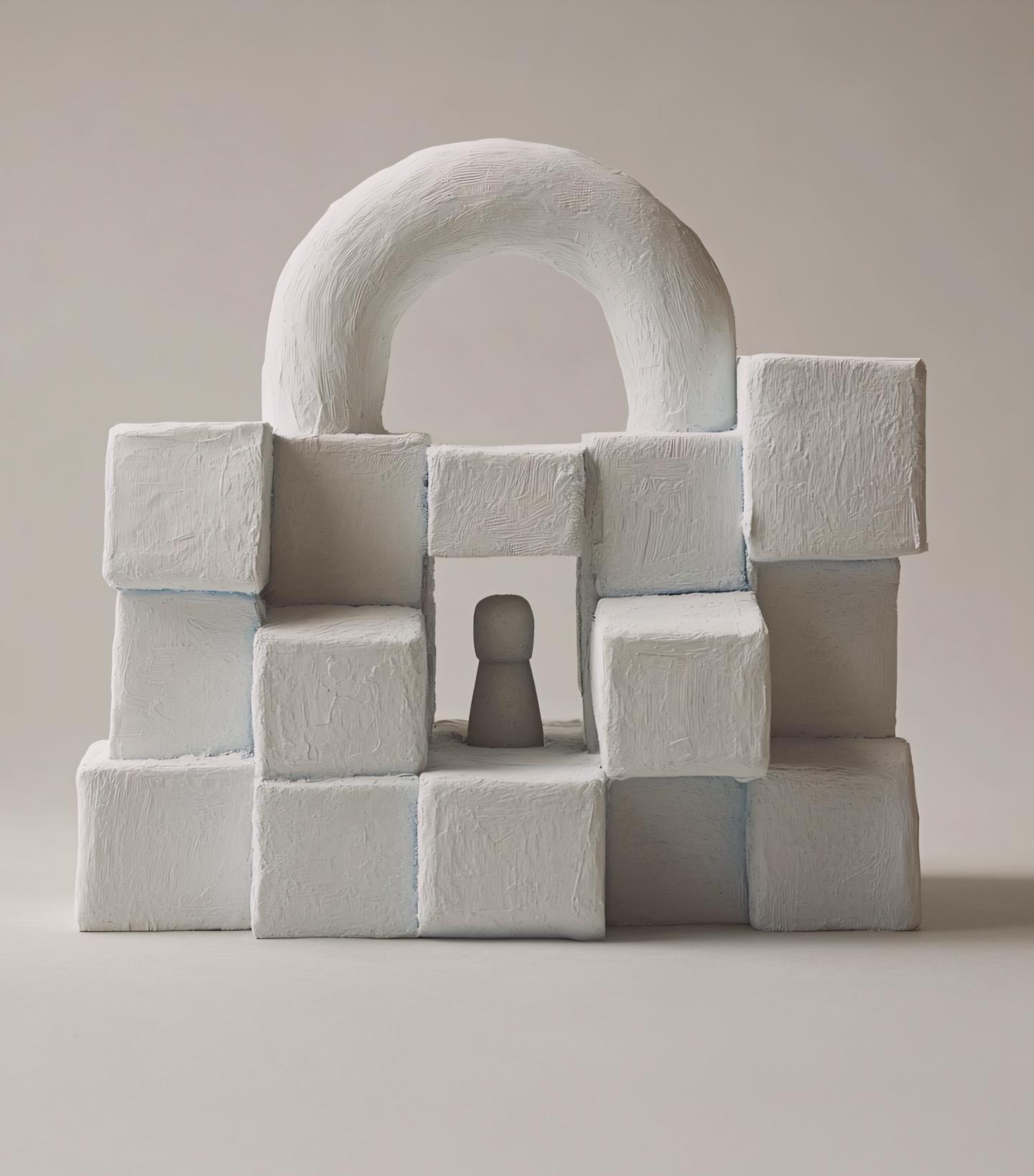
IBM estimates that each AI-related data breach costs companies an average of $4.35M, with 60% of these costs attributed to lost business and recovery efforts. Failure to secure AI models and the underlying data can result in operational disruptions, reputational damage, and significant financial losses.
Naturally, organizations are increasingly prioritizing cybersecurity for their AI systems. According to Gartner, 69% of businesses are deploying AI tools to detect and address cyber threats more effectively, aiming to protect their digital assets. However, this comes with its own investment costs, as organizations spend an average of $1.2M annually on securing AI infrastructures.
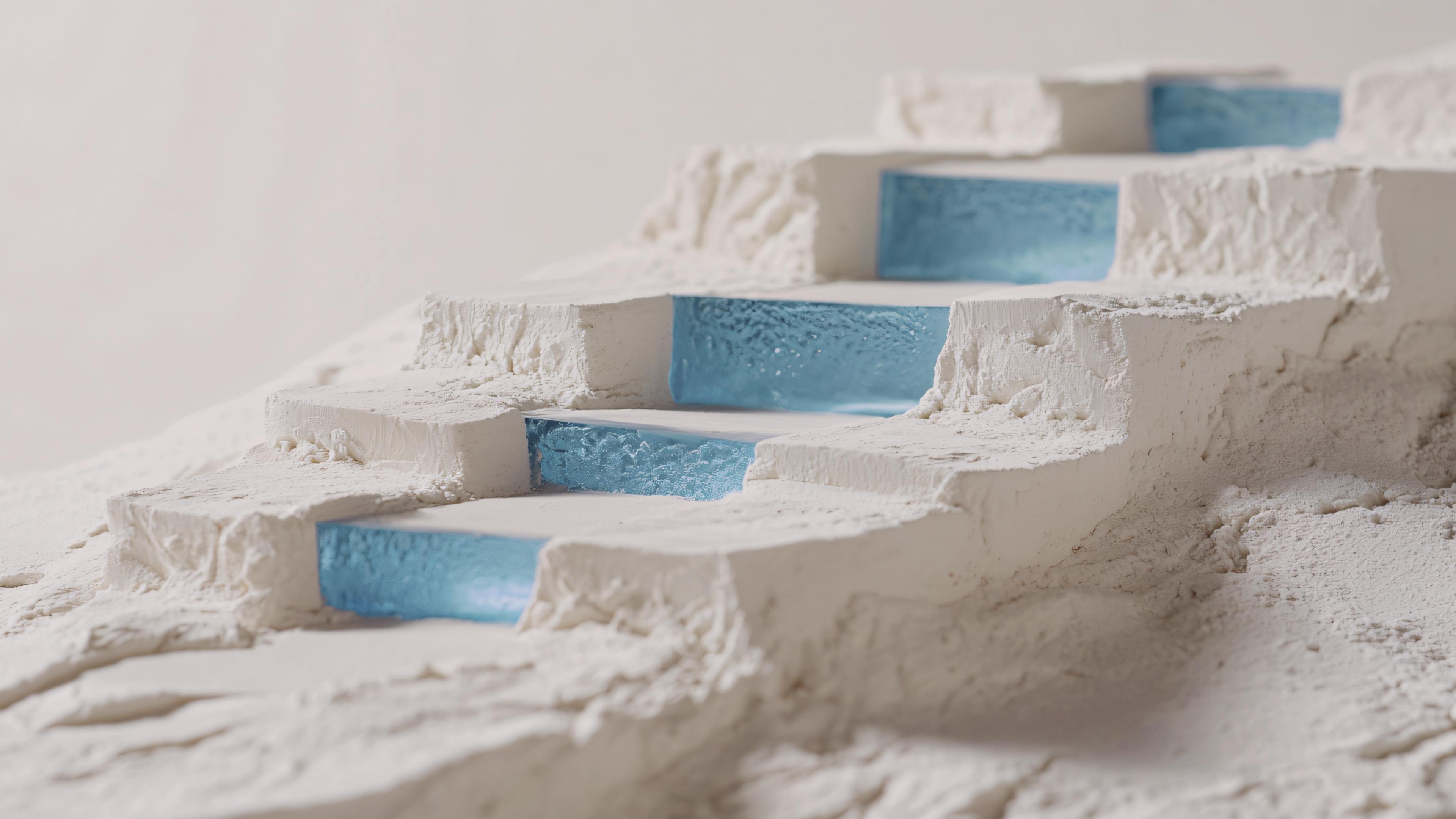
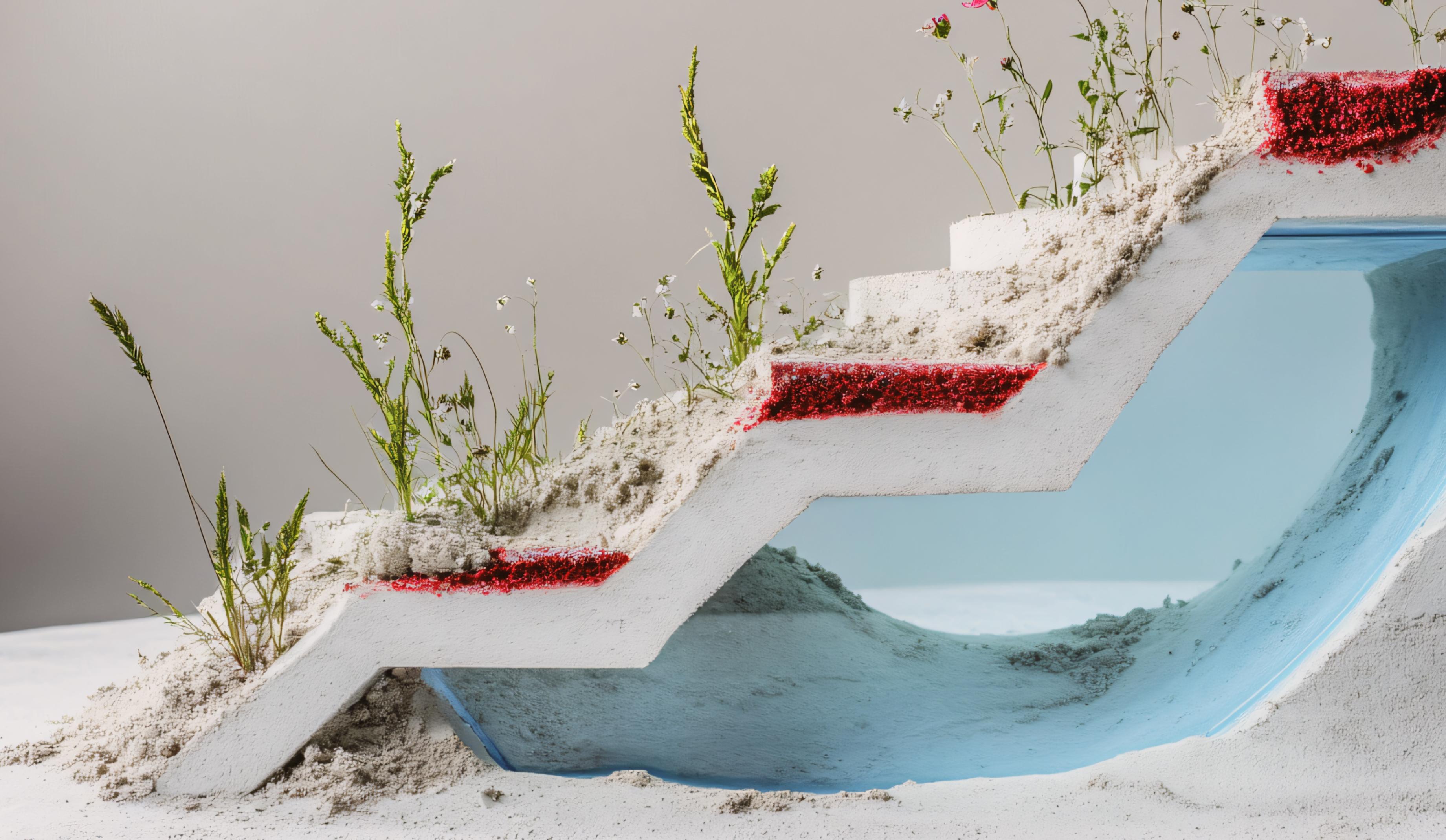
AI has already reshaped markets and redefined business operations — it’s no longer about "if," but "how much."
Companies hesitating to integrate AI risk losing their competitive edge. So, the imperative is clear: act swiftly to harness AI’s potential or… face irrelevance.
Adapting to this landscape means viewing AI as a strategic asset. From process automation to customized learning solutions, AI is becoming a versatile tool. The challenge? Navigating the expanding ecosystem, much like the early days of the internet. Businesses must differentiate valuable tools from gimmicks, maintain safety, and use AI effectively.
“AI, like any technology, is a tool. When used professionally, it enhances quality and boosts efficiency. For example, in healthcare — one of the most sensitive fields — we help our clients implement solutions that improve operational quality, reduce time and costs, and ultimately enhance patient recovery.
We handle complex data structures and multivariable scenarios, especially in global industries like logistics. With the right AI tools, optimization reaches new levels, cutting expenses and driving business success.”
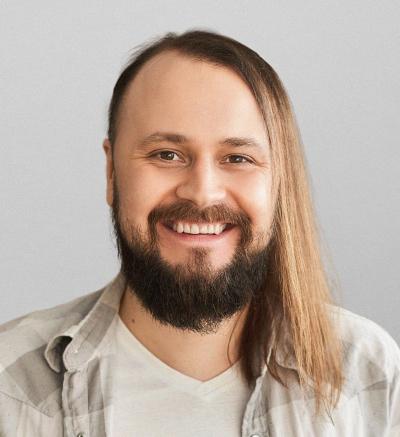
Pavel Nekrasov, AI Group Manager at Vention
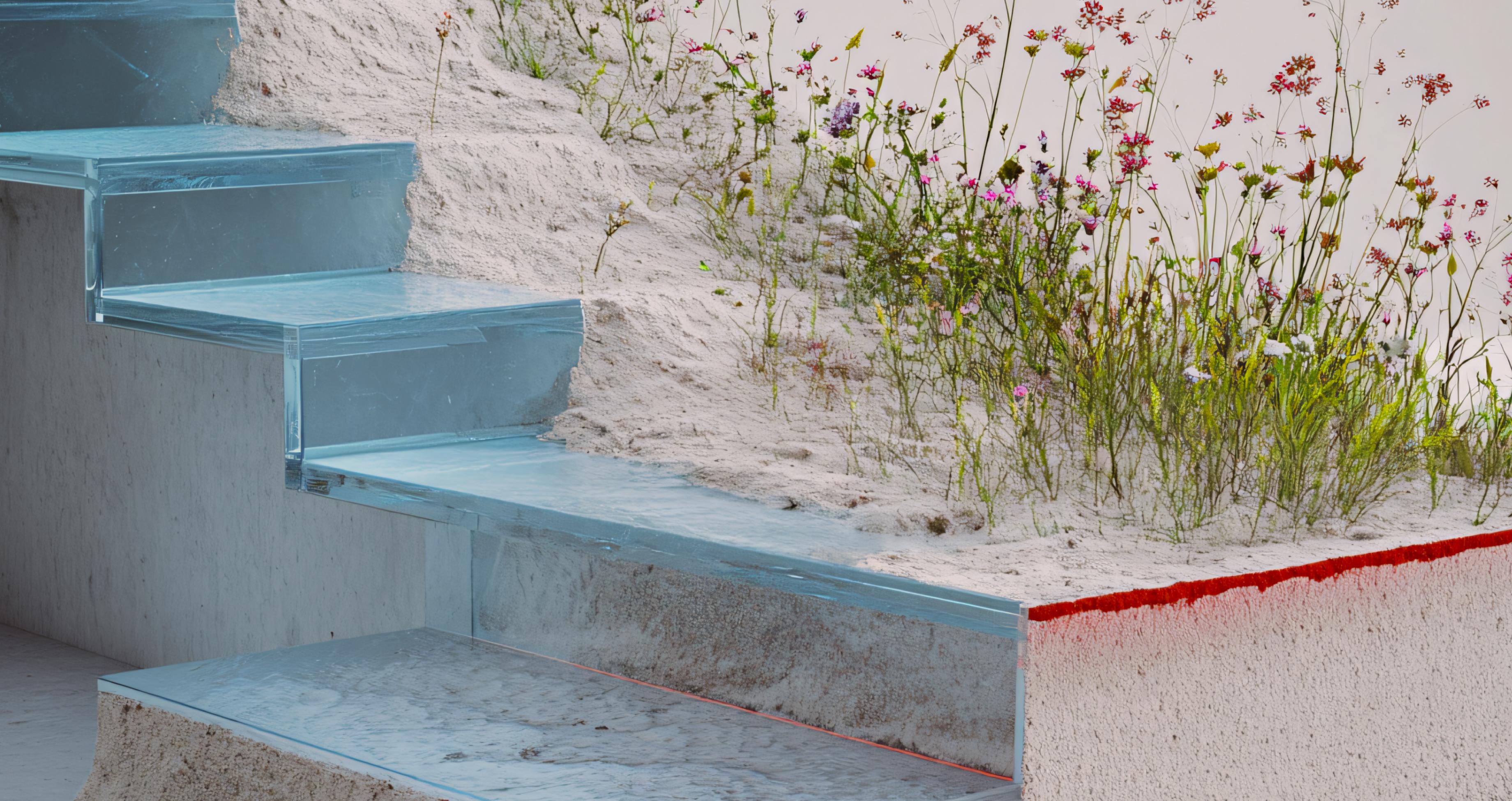
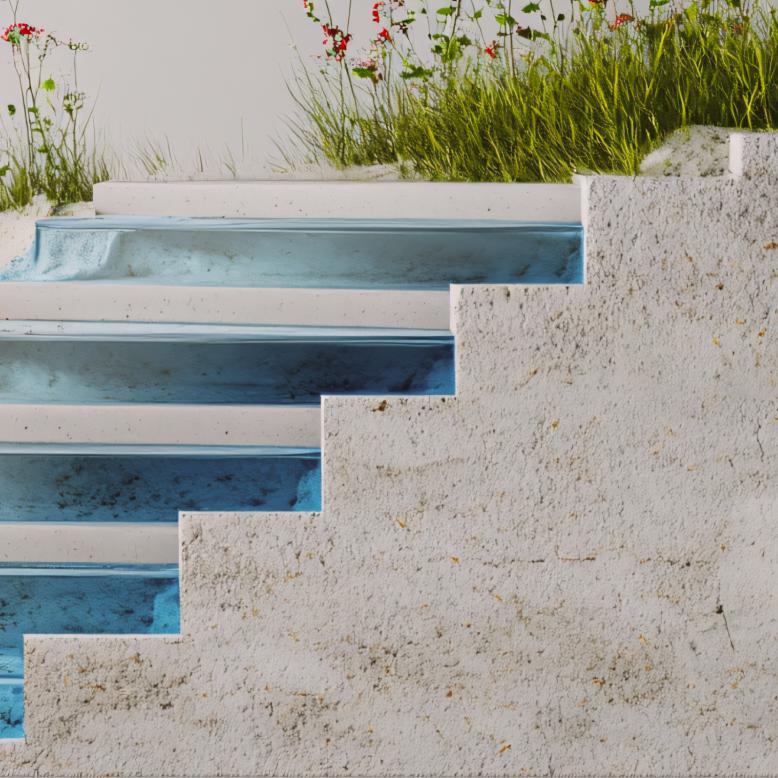
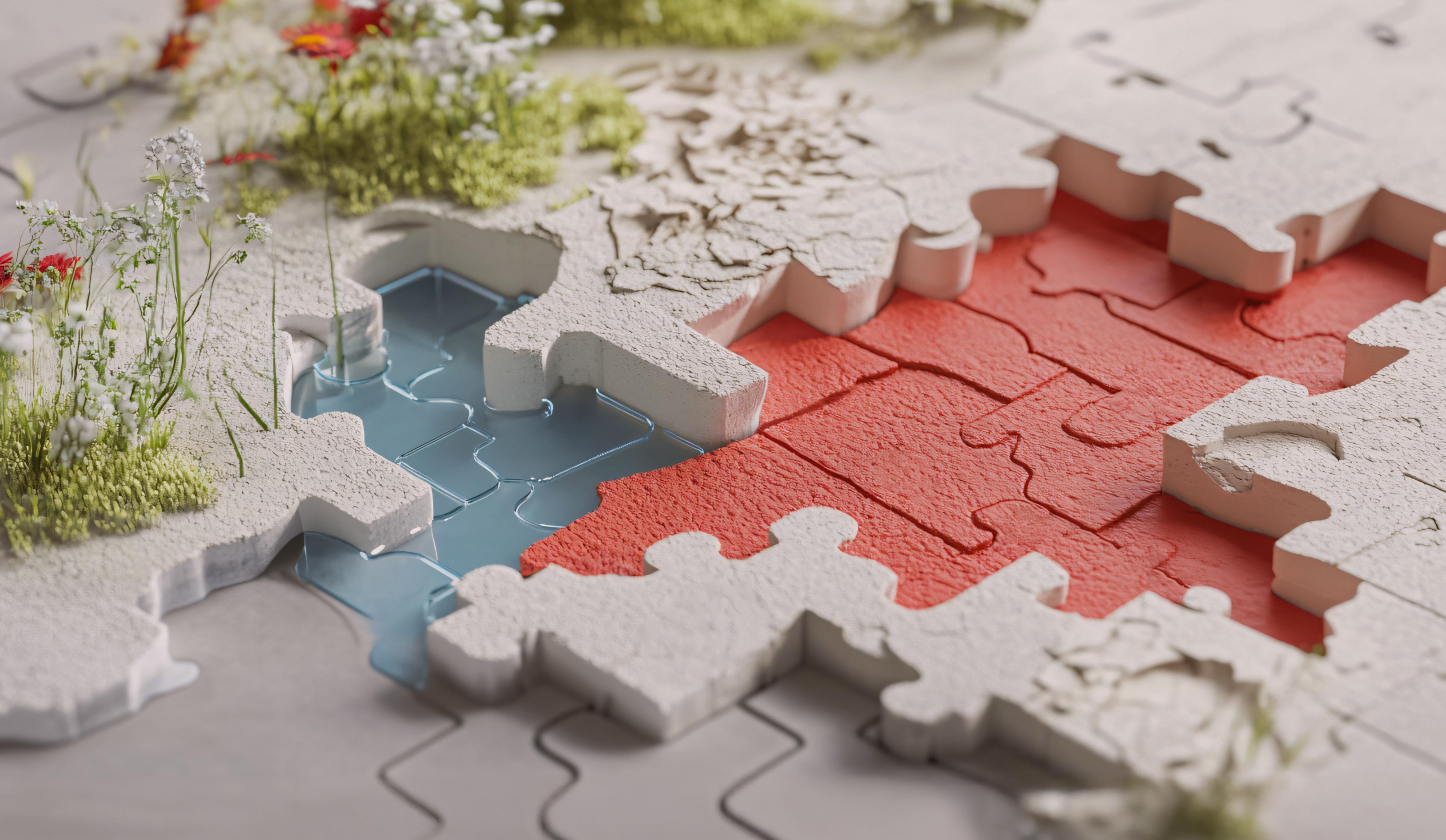
Vention is here to guide the disruptors and visionaries of tomorrow.
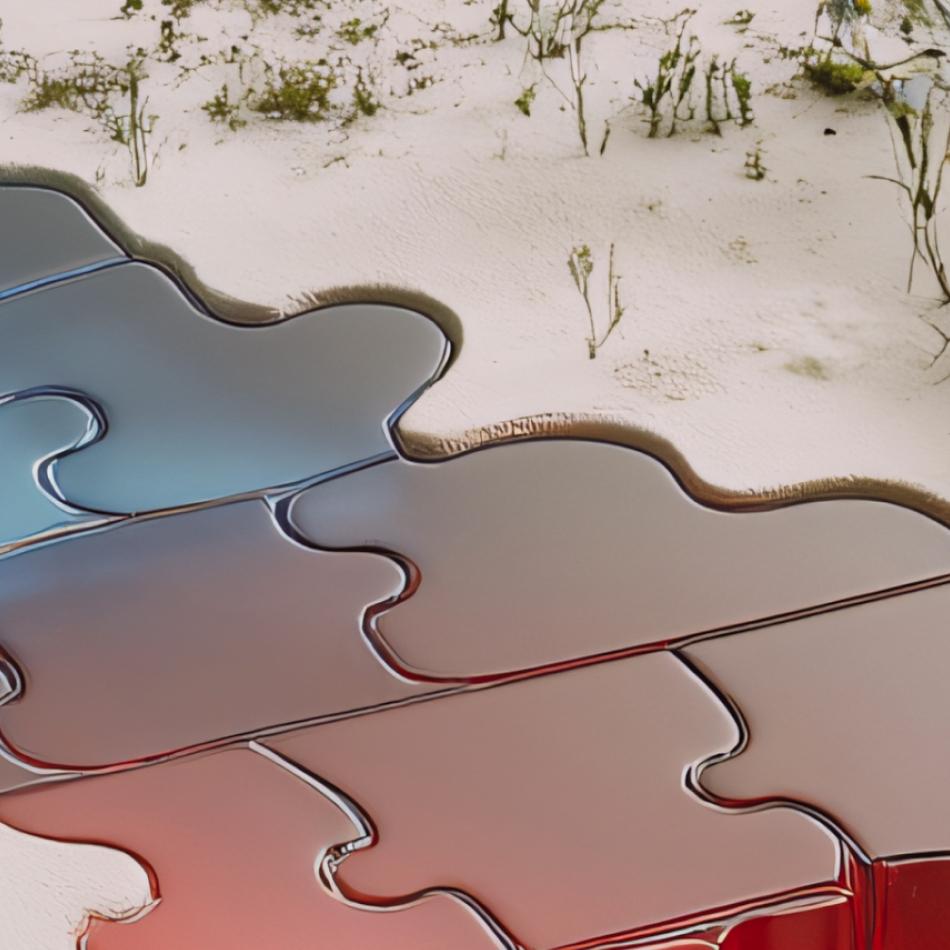
Looking for more insights or want to hear our experts' take on the state of AI in your industry?
Sources:
All artwork featured in this report was generated with AI (MidJourney).