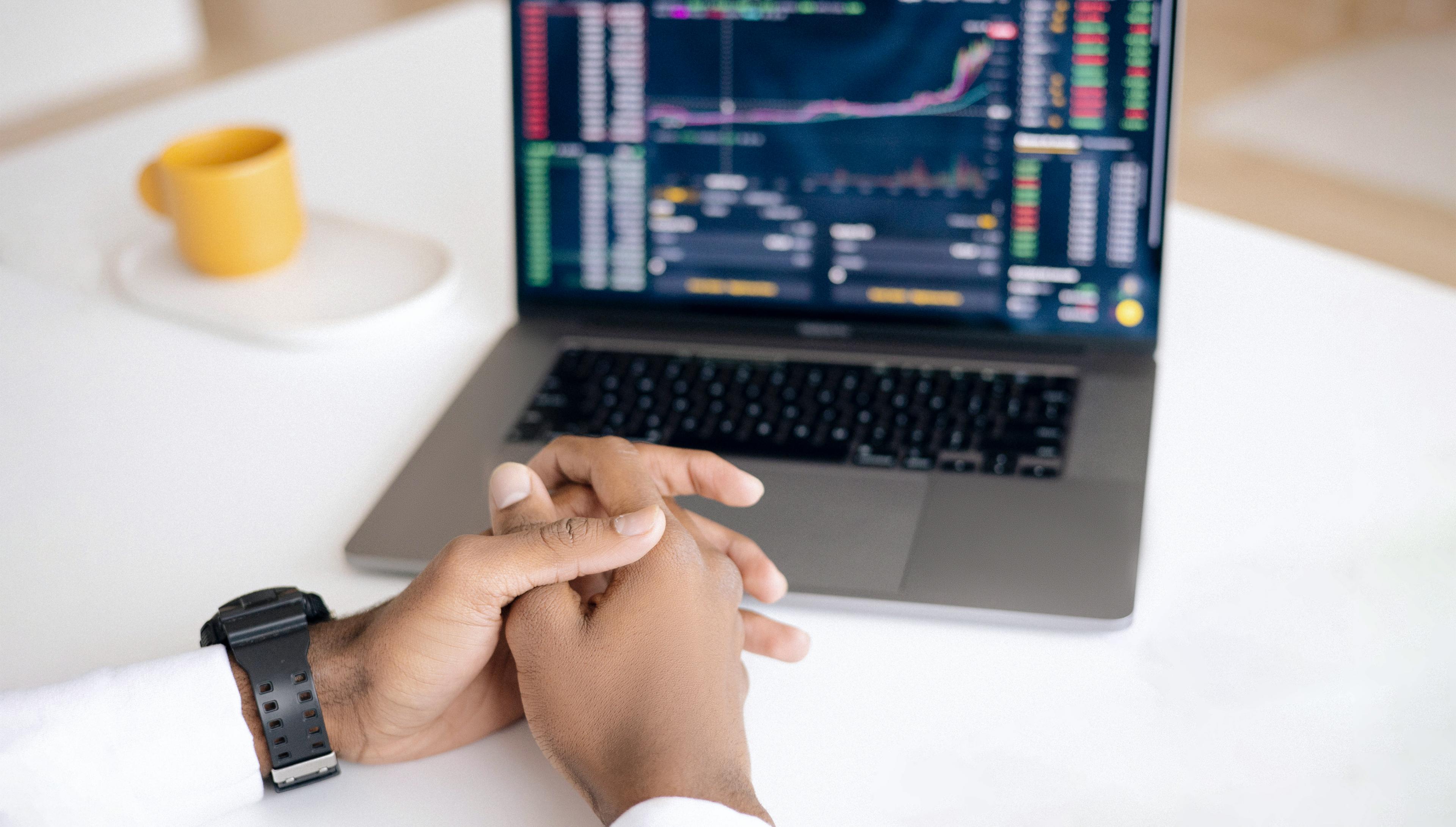
The inevitability of stock market data analytics
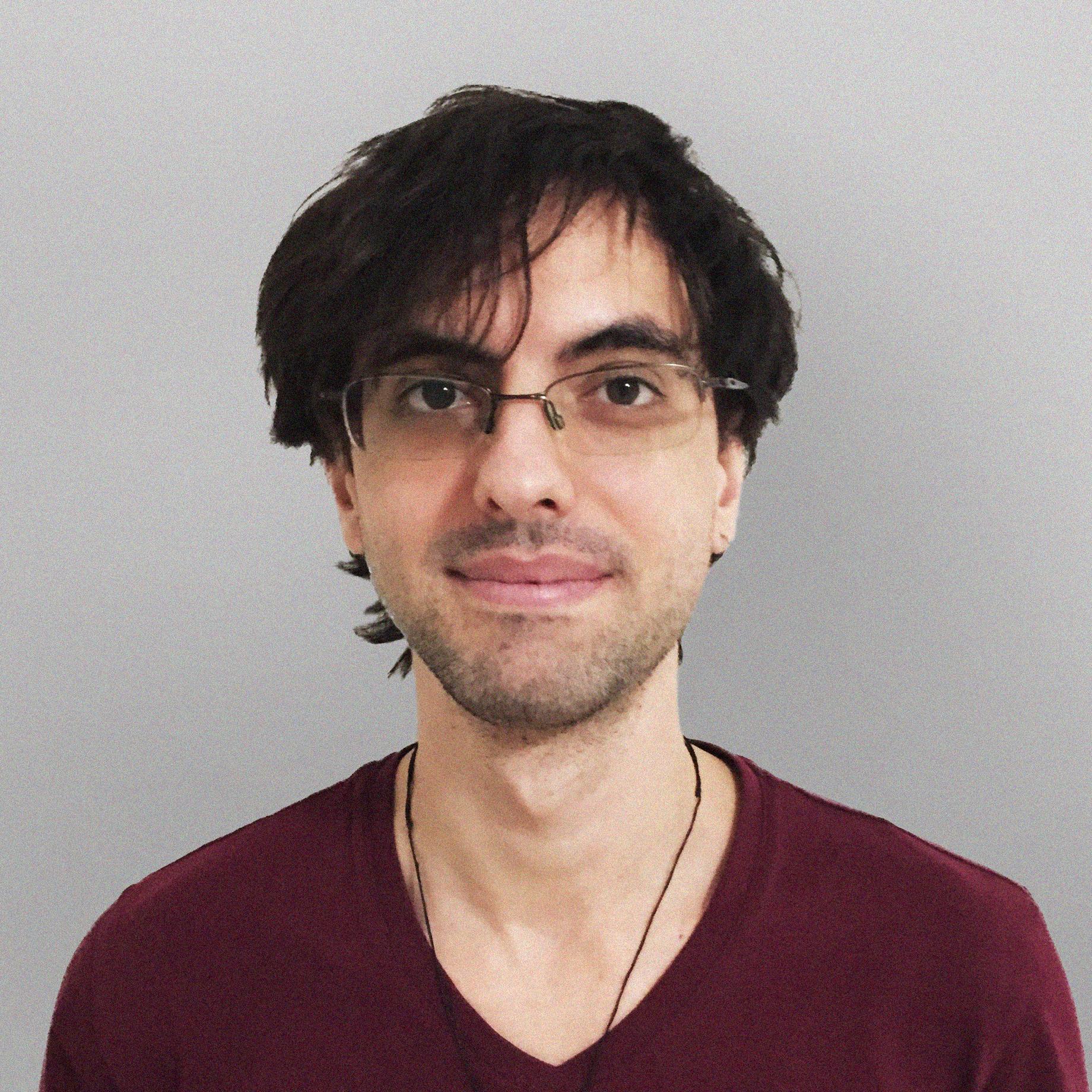
Mystique, esoterism, and all sorts of gut feelings have their place in decision-making, but only when they’re supported by incontestable, tangible evidence. Any company with grand ambitions nowadays, from startups to enterprises in every industry, must tap into modern data science to absorb and act on the insights it provides.
In finance, data analytics are crucial to interpret the ups and downs of capital markets. With great financial data science, traders and investment advisors earn the confidence to make informed decisions about buying, selling, or holding a particular security. It allows them to manage a portfolio based on short-, mid-, or long-term objectives.
How do data analytics work in the stock market?
Data analytics refers to the process of analyzing vast quantities of data to identify commonalities, insights, and trends. While data analytics tools can be used in many industries, including healthcare, politics, retail, banking, and government organizations, they’re vital for competitiveness in modern financial markets.
As with most industry verticals, stock market professionals have become increasingly dependent on current-and-next-gen tech to do their jobs and manage portfolios. Big data is routinely tapped in the financial sector to reduce previously unknown risks as much as possible. AI has already been used to predict stock performance based on quarterly earnings calls. And while data science relied on rigid algorithms in the past, the landscape of financial data analytics nowadays is ruled by machine learning, particularly neural networks.
Natural Language Processing (NLP), for example, relies on neural networks to understand human speech. NLP software can listen to conference calls and read business reports, and then digest the data for traders to capitalize on. As with other machine learning disciplines, NLP self-adjusts based on new information. So, the more qualified the data sources are, the better the outcome of machine learning’s self-updates.
Key uses for stock market data analytics
Whether your goal is to make smart split-second investment decisions, protect existing portfolios, or identify potentially fraudulent behavior, data science will serve you well. With top-tier financial data analytics, it’s possible to:
Get capital market insights in real-time
Let’s start with the basics. Using streaming data feeds, traders can combine historical data with the real-time movements of financial markets to predict patterns and plot their next moves. With immediate access to reliable insights, both day traders and long-term investors can better refine their portfolio strategies based on whichever stock performance criteria they choose.
By building valuation models for data analysis, investment companies can feed test data sets to their data analytics solution and swiftly retrieve the theoretical results. Then, those theoretical results can be accurately tested to analyze stock fundamentals and other data sets once paired against the historical records.
Enable algorithmic trading
At its simplest, algorithmic trading can be found in any respectable stock trading platform: When a retail investor sets his smartphone app to automatically “buy when the price goes below $60 per share,” that’s algorithmic trading in action. Just a straightforward if-then conditional.
But it gets more complicated quickly: Specialized investment platforms allow for commands such as, say, “sell when the 50-day moving average goes below the 200-day moving average” and beyond, with increasing levels of complexity. Those market insights obtained previously can now be automated — and the proper config enables investors to track and execute their trading strategies without the constant need for price monitoring.
Algorithmic trades can also be used to rebalance investment portfolios automatically. For instance, if the application analyzes a portfolio with an 80/20 stock-to-bond allocation and finds it uneven, it can sell stocks to purchase more bonds whenever the stock value reaches the 80 percent threshold.
Provide better strategy for customers
Beyond direct trading, data science is used to get better insights into the customer base of financial institutions. Asset management Empower (formerly Personal Capital), for example, uses predictive data analytics to assess the projected growth of each customer’s investment portfolio based on the amount they expect to contribute over time. With it, Empower can provide a tailored ballpark to its clients indicating how much they can earn on their investments or will need to save for retirement.
By analyzing the customers’ goals and spending habits, companies can build customer profiles and segment them into groups that help estimate how much each customer is likely to invest in — or divest from — their organization over a given period. Furthermore, these profiles can be used to develop personalized marketing content and investment management strategies to keep customers engaged.
Identify fraudulent behavior
Stock market data analytics aren’t important just for investors and advisors, but also for regulators. Security professionals know how to delve into data analytics to uncover abnormalities in stock trading — a potential sign of fraudulent behavior.
Regulators use data analytics to set up algorithms that help them monitor the risk score of capital market transactions, automatically flagging anything that falls outside of the expected range. When a data set is marked as suspicious, human analysts manually take over to investigate whether fraud or market manipulation is taking place. By following the money (in the form of data), regulators may identify which specific investors were able to profit from the price fluctuations and audit their accounts appropriately.
This security effort shouldn’t be, however, exclusive to regulatory bodies. After all, individuals and companies must disclose what they are tracking to curb insider trading, or they risk hefty fines or even jail time. It’s in the direct interest of investment firms to buy into internal risk management to mitigate risks posed by employees potentially engaging in fraudulent activities.
Security-oriented data analytics can identify a company’s key risk indicators, notify whenever there’s a high chance of wrongdoing, and establish a mitigation plan as fast as possible to prevent potential losses or liability.
Curious to see what your stock market analytics solution may look like?
Go ahead and schedule a quick chat with our fintech leaders.
The role of trading indicators
Trading indicators deserve their own spotlight because they’re the part of the backend that makes data analytics in the stock market work.
“Akin to how open banking is enabled by a collection of APIs that can be deployed at will for a small fee, trading indicators are stock market API-of-sorts that monitor and aggregate historical data on any given ticker,” says up Andrew Haines, Global Head of Fintech at Vention.
Once gathered, the data is processed against multiple technical indicators — hard statistics such as stock price and trade volume — to determine the direction in which the wind is blowing. Toward a buy? A sell? Long? Short? That’s what the trading indicators try to answer.
Trading indicators come in all shapes and sizes. Andrew points to some key players in the financial market:
Barchart Opinions
The fintech giant (also a Vention client) boasts a comprehensive data analytics solution for traders and portfolios of all scopes. Barchart’s platform can track and retrieve up to two years of a symbol’s historical data and consult 13 technical indicators to reach its verdict on any given stock
TradingView
Trusted by 30 million monthly users, TradingView, a charting platform and social network, counts on a sleek design and customizable charts to get its message across. It works well on web, desktop, and mobile and comes with 100+ pre-built popular indicators, plus over 100,000 community-built ones (yes, you read that right).
Apache Kafka
The open-source Kafka excels at simplifying scrambled data feeds into actionable insights. Used by top enterprises globally across industries, Kafka’s low-latency, high-throughput data analytics systems are near-indispensable for traders operating on multiple investment fronts.
MATLAB
Mathworks’ MATLAB is a data analytics platform that caters to developers and computer scientists. MATLAB enables fast testing of novel algorithms and models to optimize the creation of personalized trading strategies. Its data analytics solution encourages the automation of data pipelines such as equity trading order workflow.
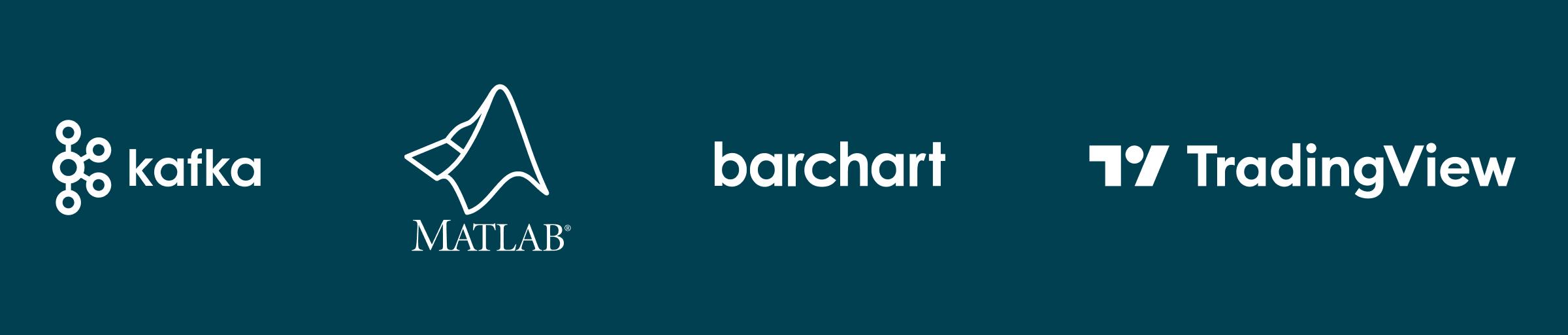
Choosing the proper stock market data analytics framework
The best stock market data analytics solution can track, centralize, integrate, and process all the relevant data needed — a financial institution’s own organizational data, the broader capital markets data, and other streaming data feeds. They can be roughly divided into pre-built models and personalized solutions.
While some pre-built data analytics frameworks don’t require experience to use, those still need some level of technical support with implementation and data integration to set up and onboard.
Alternatively, if the business calls for a custom financial software solution, advisors and data analytics platform developers must work side-by-side to turn the company’s economic models into algorithms for the framework AIs to work with.
Proprietary data analytics solutions take longer to implement than an over-the-counter SaaS product, but provide code ownership and flexibility to create the unique features needed by the enterprise. Plus, there’s no need to deal with the uncertainty of changing rates or shifts in product offerings that are so common in subscription-based services.
Chance, after all, is better left at the fortune-teller’s tablecloth.