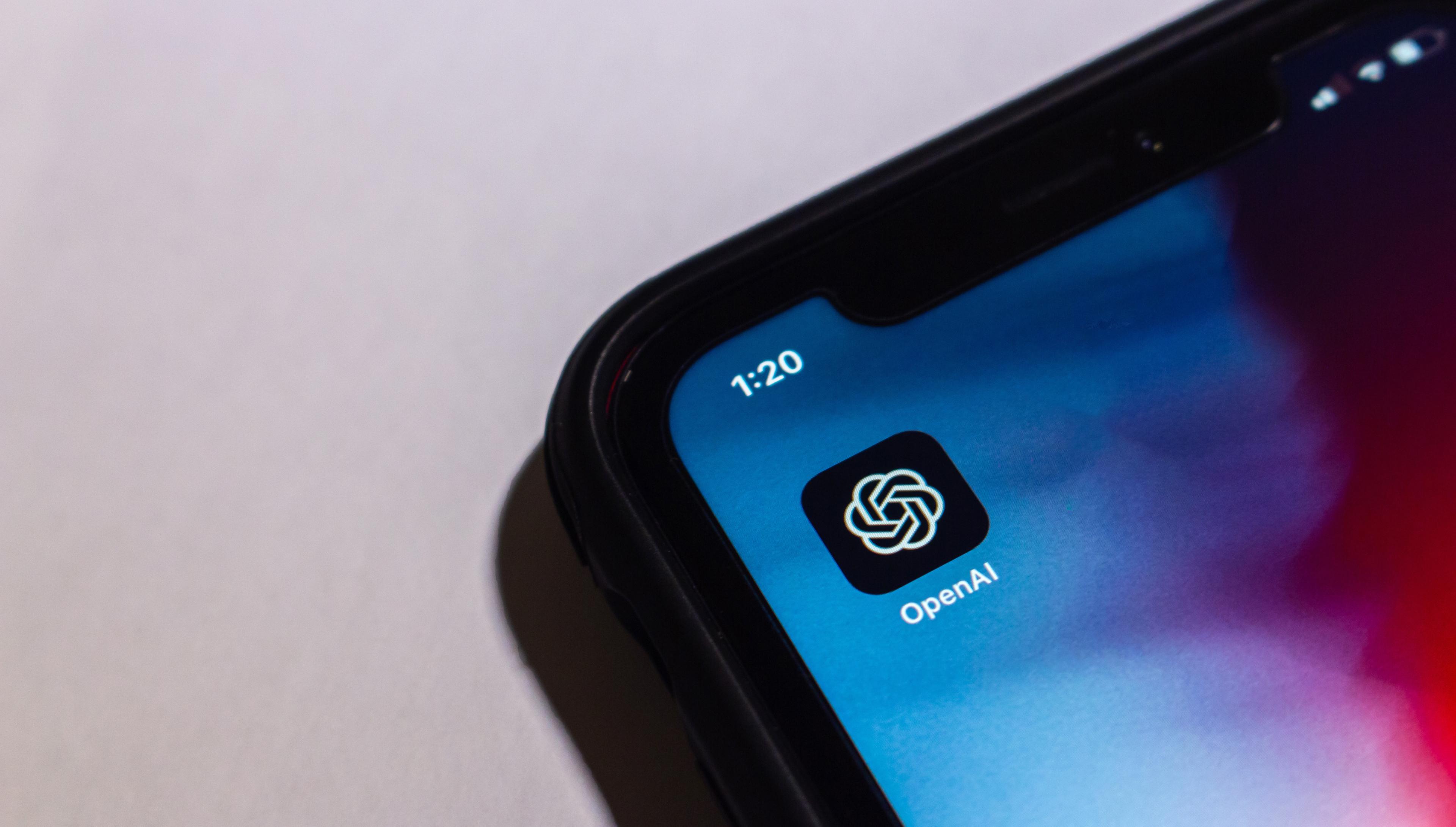
How to handle challenges in artificial intelligence
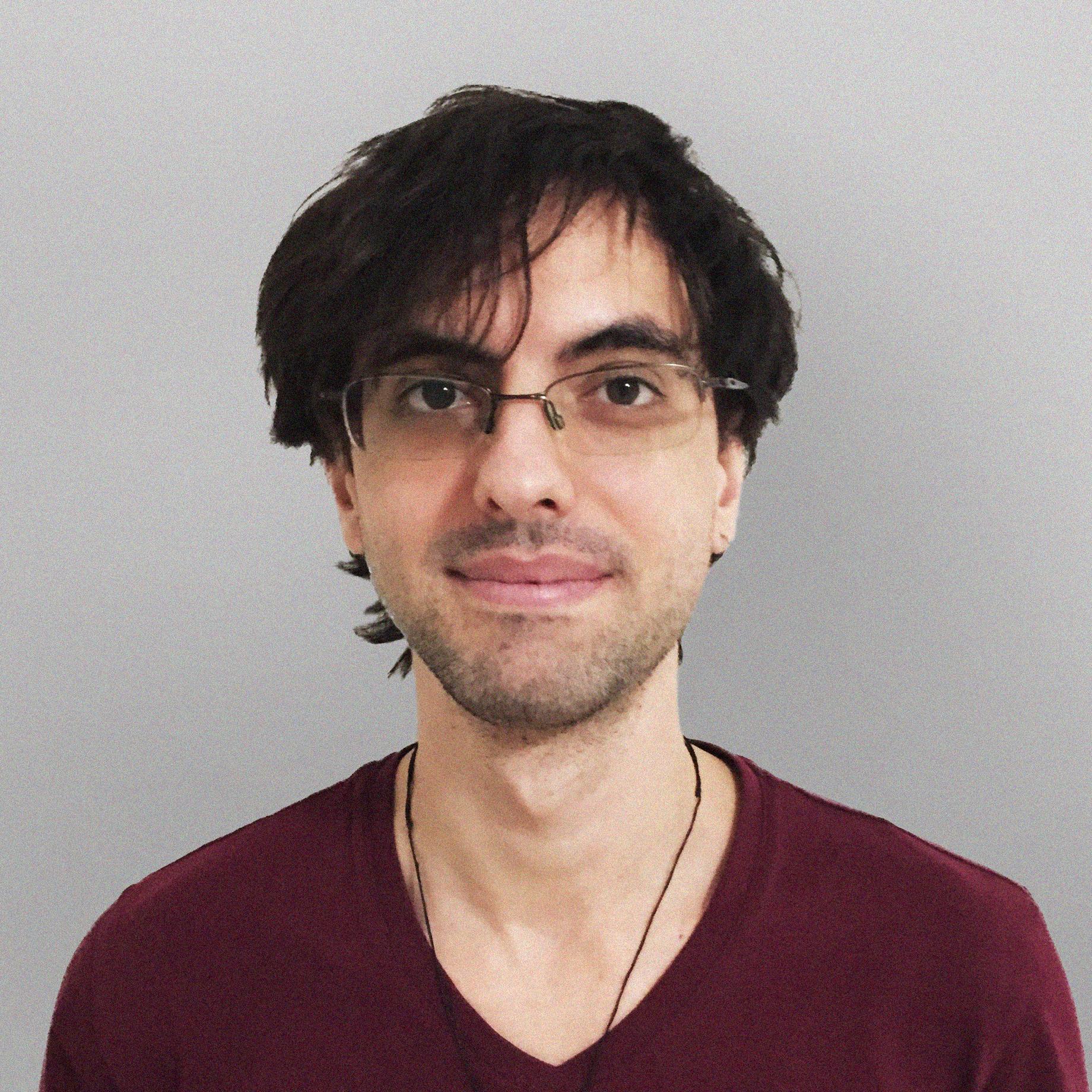
Key takeaways
-
AI is increasingly moving from theoretical to practical use in customer interactions and creative fields, raising both potential and complexities.
-
AI development faces technical and social challenges, including the need for enhanced transparency, better data security, and navigating possible biases and ethical issues.
-
With AI's growing presence, it’s crucial to balance tech innovation and regulatory compliance, emphasizing ethical coding and human oversight.
“Hi there! Got any questions? I’ll be happy to assist!”
Hark the robotic cheer of support chatbots, the closest touchpoint most consumers have with artificial intelligence (AI) — and one of the simplest applications to process. Humans input a (basic) question, and AI outputs a (basic) answer.
Now, with AI-generated art to ChatGPT fever, the applications — and anxieties — of artificial intelligence have put a spotlight on the technology’s potential and complexity. While creative AIs and their latest explosion in popularity might feel disorientating (as if they arrived “too soon”), they’re part of a larger trend: Artificial intelligence is, on a technological scale, transitioning from theory to practice. AI’s seemingly limitless playbook of possibilities has gone full-steam, converting potential use cases into reality again and again.
That leaves us in a world where AI is everywhere and legislation is attempting to play catch-up with technology breakthroughs (dig the White House’s Blueprint for an AI Bill of Rights). Suffice it to say, companies building AI and machine learning-based solutions have plenty of pressing questions surrounding the tech.
What are the biggest artificial intelligence challenges of today?
Most of AI’s major hurdles today fall into one of two categories: technical or social. Technical hurdles revolve around the practical goals of AI development, and if it’s adding value and solving business challenges. Social hurdles are rooted in the legal, ethical, and behavioral impact of widespread AI usage on society at large.
To an experienced AI developer, these concerns are intertwined: Not only must you code artificial intelligence well, you gotta do it so none of the results are unfortunate at best and evil at worst. Autonomous car companies, after all, may earn nice guy points by having their machine learning algorithms optimized to reduce emissions, but that’s irrelevant if “one-in-a-million-chance” system bugs trample pedestrians.
Put another way, genius AI coding must solve the problems its own implementation creates.
Common technical AI challenges
Lack of transparency for customers
Imagine that your avant-garde money lending app uses artificial intelligence and a natural language processing (NLP) chatbot to decide which applicants get their loans approved, but it doesn’t explain to customers who don’t get approved why they didn’t make the cut. Give them the silent treatment on that — without setting expectations up front — and you’ll likely have some rightfully bitter users. Rather than operating as the crown jewel of your customer support network, your NLP is going to drive prospects right into the arms of your competitors.
Internal stakeholders don’t know what they’re getting and how to leverage it
All the data sources your AI app certainly is tapping (it is, right?) from user interactions is only as valuable as your ace data analytics and machine learning experts make it. For example, great NLP-based applications can read customer sentiment based on word choice and tone of voice, something that “classic” AIs, like regular chatbots, can’t — but only if the NLP solution is built to extract the right data from inputs and then digest it to produce genuinely helpful, actionable insights the marketing team can use. Trained, knowledgeable humans need to coordinate on the inputs and the outputs.
It isn’t magic
No artificial intelligence will magically solve your most pressing problems by itself. Assist, sure; not solve. A smart algorithm is still an algorithm. Since machine learning hasn’t reached singularity levels (yet), everything that an AI solution is intended to fix must be anticipated so that the devs can plan and code it properly.
The same applies with deep learning: Its algorithms are developed to figure out patterns within highly specific tasks (like muscle movements in facial recognition, or paths to victory in the game of Go) and thus need highly specific datasets and instructions to attain maximum flexibility in their limited field. Tell the AI to “improve sales” or “optimize inventory” and, well, the results will be underwhelming.
It can’t replicate human judgment
How should your artificial intelligence behave when it finds deviations from expected patterns? The same way a human would, of course: by calling their supervisor.
An AI is only worth the cost of developing it if both its received inputs and returned feedback are sound — and often only a human can be the judge of that. No matter how advanced your algorithms are, they must be checked and tweaked over time to ensure that new company or market circumstances aren’t rendering their data quality, and thus feedback, obsolete.
Moreover, your human team may catch things AI doesn’t. As much as it is convenient to box customer data into archetypes to be processed in bulk by machine learning algorithms, the reality is that not all prospects are created equal. Some indeed carry a lot more potential than others, something that a data management AI might overlook when parsing data sources alone to suggest your next move.
Over-reliance on AI may impact productivity
Why learn new languages when there’s Google Translate? Why memorize directions when there’s Waze? Why familiarize yourself with your company’s ins and outs when AI does the heavy lifting?
Because as convenient as those tools are, they’re also flawed, and when you don’t catch the flaw, you waste time. Google mistranslates something? Oopsies, it’s going to take time to identify the mistake and fix it. Waze miscalculates the quickest route? Oopsies, you spend another ten minutes on the road (or even get sent to the wrong city.)
The value of AI tools rests in part on the technology’s ability to cut routine tasks short, but that doesn’t mean it eliminates the need for a human brain. And they shouldn’t have to: AI is at its best when integrated into existing business practices and applications — which are very much born of human brains — not the other way around.
Of course, tools evolve, and so does our reliance on them — no one needs to know how an abacus works to use calculators. However, we’re not there yet with AI. People across your enterprise who have a hand in your AI, either the input or the output, must be able to speak the same language to a certain degree.
It’s vital (and near-mandatory in agile workplaces) for teams to be aware of how others operate. Long term implications aside, artificial intelligence is, for now, a time-saving and value-adding tool for your staff. It doesn’t replace them.
Isaac Asimov’s First Law of Robotics states: “A robot may not injure a human being or, through inaction, allow a human being to come to harm.”
There, done. Just follow it and we should be all right.
If only the world were so simple. While Asimov meant "harm” as in “direct physical trauma” (and by actual androids), there are plenty of indirect ways by which disembodied AIs can hurt people. Worst of all, in many cases, it can be hard to evaluate if the pain comes from unintended consequences of good intentions, indifferent corporate greed, or a mix of both. We’re getting better at it, though.
Legal gray areas
Legislation should act as the primary safety net against the toxic use of technology, but it’s never been faster than app updates. Tech-related lawmaking is notoriously reactive, giving tech companies leeway for bad and even predatory behavior. Consumers are left with little more than boycotts and noise on social media to defend themselves.
But as society becomes more tech-literate, our collective trust in the goodwill of tech companies wanes in favor of the letter of the law. And AI regulations are coming fast — the first round is already here, with Europe’s groundbreaking GDPR leading the way regarding data management, privacy, and security concerns. Newer laws meant to safeguard against various aspects of AI, from discrimination to autonomous weapons that blur or outright dismiss parameters of agency and decision-making accountability, are just around the corner.
An AI app that doesn’t take this regulatory landscape into account during development will have to be rebuilt once policies kick in. Make no mistake: More and more, companies that play fast and loose (perhaps even the giants of the industry) will face having their entire business models outlawed, particularly with regards to personal data protection.
Recognize the legislative trend and future-proof your AI projects today by anticipating the protections of tomorrow as much as you can. Of note, having a dedicated ethics specialist on board is a great way to traverse legal murkiness.
Code riddled with human biases
Artificial intelligence is created, managed, and updated by imperfect, biased humans. Naturally, these faults often end up in the technology’s fabric — and they can be hard to catch.
The issue was recognized by the US federal government in May 2022, with a warning for employers to not blindly trust AI and machine learning algorithms to act like Human Resources recruiters — lest they breach the Americans with Disabilities Act. It turns out that optimizing AIs to evaluate a candidate’s fit solely based on standardized metrics (such as keystrokes-per-minute) ignores potential handicaps like mobility impairments.
Then there are the inevitable racial issues. Remember the stir caused by wearables that didn’t accurately monitor the heart rates of consumers with darker skin? The solution was to update the algorithms to recognize odd readings and automatically increase the light’s electric current, thus amplifying its ability to penetrate the skin.
While the problem got (mostly) solved, the fact that the update had to be rolled out instead of being a factory default demonstrates just how insidious these situations are. A glaring lack of data sources and proper testing across a larger spectrum of the populace can tumble AI just as easily, so it’s crucial that developers and regulators be extra aggressive about identifying what’s overlooked.
A breeding ground for disinformation
Social media is another realm where artificial intelligence’s optimization of metrics — in this case, for engagement — has dire consequences for society. With the evolution of machine learning and creative AIs, the potential for engagement is virtually infinite, and so is the potential disinformation.
Deep learning creative AIs already span multiple genres. Music composition is represented by Chopin nocturnes fused with Bon Jovi; writing has featured a robot earning a byline in The Guardian; art, well, has been thoroughly demonstrated by Lensa. Add deepfakes to the mix and realistic video AI is right there alongside other creative media.
This AI challenge is compounded by the fact that deep learning creative AIs can now create and spread disinformation by themselves: The same GPT-3 algorithm that wrote for The Guardian is also capable of convincing people that its AI-generated fake tweets about foreign policy and climate change were real. And if fully automated data-gathering AIs can’t pinpoint right from wrong, their datasets become corrupted, and their value is entirely compromised.
As with any other tools, AI automation and their metric optimization potential are terrific assets only when employed side by side with critical thinking. Having a capable human team acting as the curators of your AI’s output — whatever that output is — still is the safest way to prevent AI-generated blunders.
AI has only just arrived, and yet it’s everywhere
Due to the immense scope of applications and new solutions (or updated versions) to old problems coming along at every blink, grasping precisely where and how artificial intelligence and machine learning can assist your company can be overwhelming. Operationally? Logistically? At post-lunch meditation breaks?
For many businesses, the answer will be all of the above. But always with moderation: AI is a tremendous tool with Swiss Army knife applications across every possible industry and vertical, yet a tool that demands the human touch in order to be safe and useful. The true value of any AI is always dependable on how knowledgeable its corporeal handlers are.
When it comes to avoiding pitfalls, it’s clear that AI development is far more advanced in the technical field than the social one. Few emerging technologies have become as omnipresent in such a short amount of time as AI — and even fewer have the potential to completely wreck humanity if we don’t pay due attention.
From our right to data privacy to job offerings and security, to credit scores, and perhaps dream interpretations, one would be hard-pressed to find an area where AI hasn’t yet made inroads. Much like cars pushed the horse and carriage out of business, AI is poised to redefine entire professions and perhaps even make them obsolete — again, in due time.
We can’t be sure what will be the long-term consequences of this level of AI development for society, and attempting to do so is but an exercise in divination. As those can backfire spectacularly (“no computer network will change the way government works” is a personal favorite), we’ll play safe and call it a day here.
Puzzling over how your business can make the most of AI?
Our experts can help you find the way forward.
Social AI challenges . . . thus far