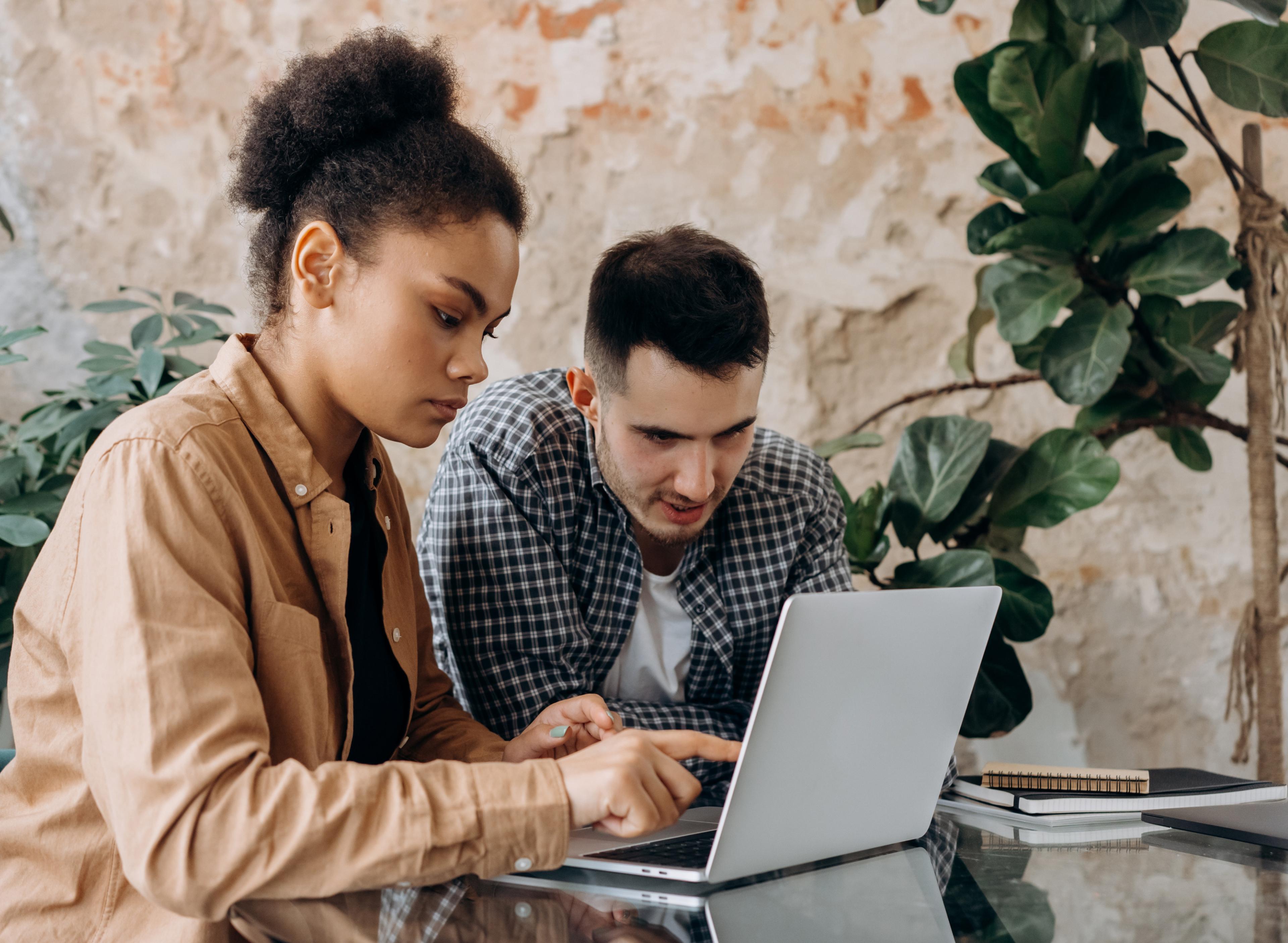
The Future of Software Developers: AI and Prompt Engineering
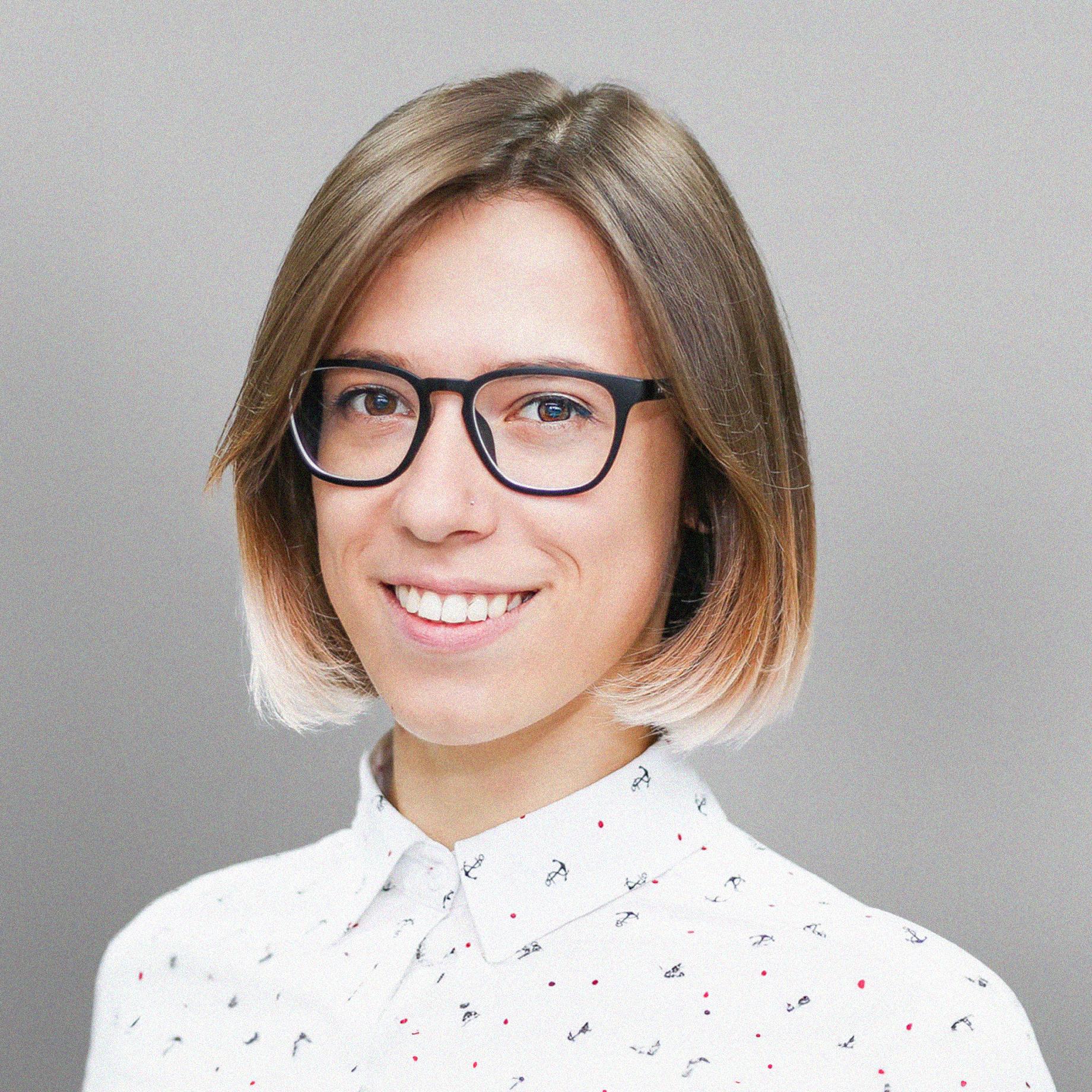
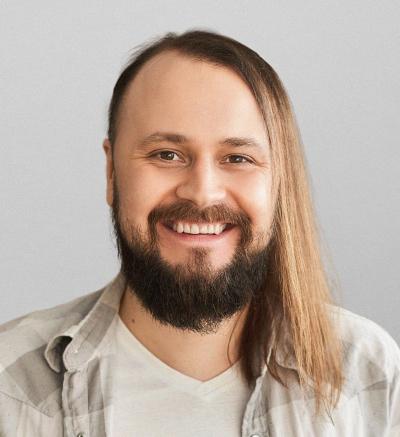
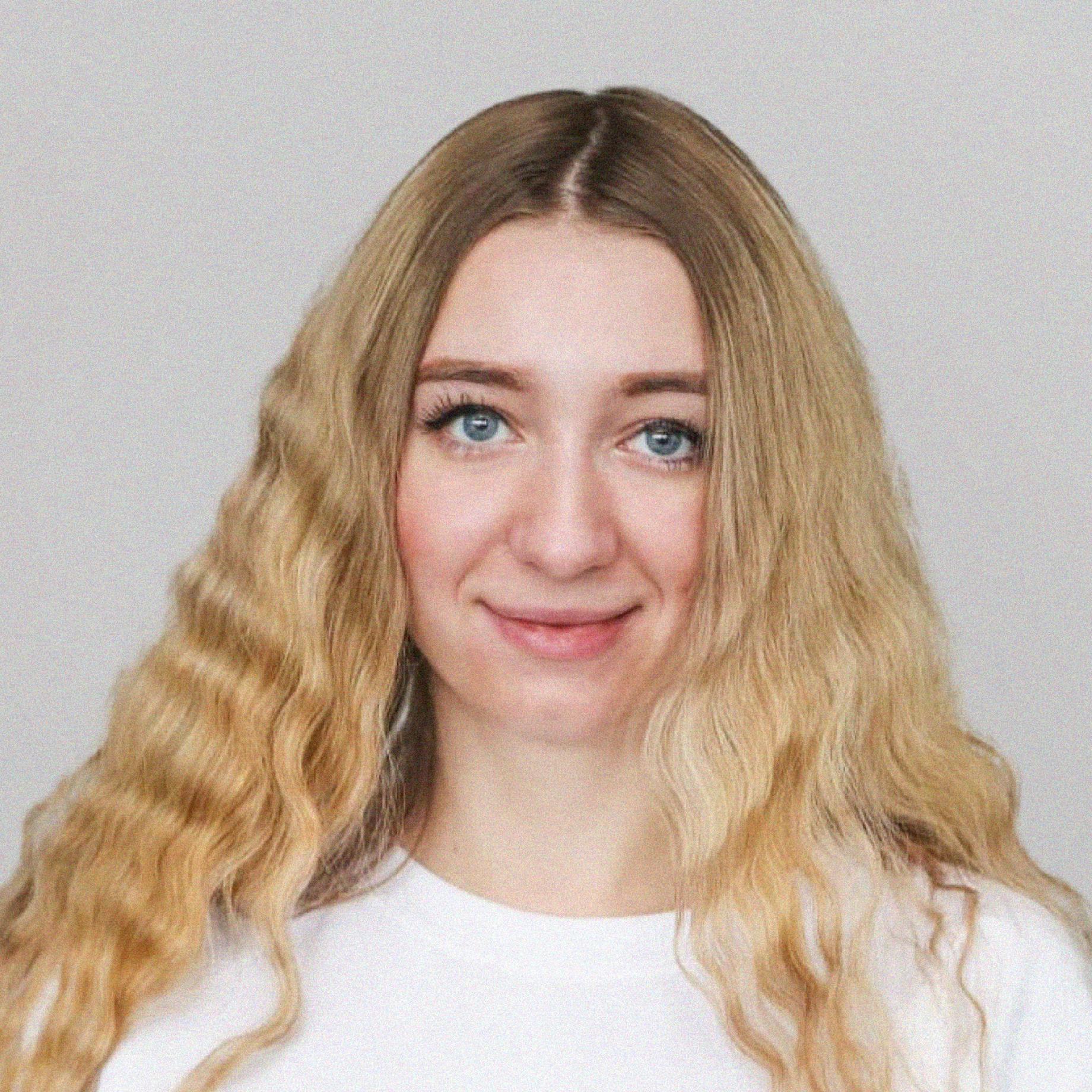
A recent study by Goldman Sachs estimates that one-fourth of current work could soon be replaced by artificial intelligence — with 37 percent of all tasks within engineering on the brink of complete automation. This reflects the broader trend of AI adoption across industries.
But is AI really coming after tech jobs? Or is it more a matter of AI making existing jobs easier, rather than eliminating them?
To get the answers, we went straight to those on the front lines — Vention AI engineers.
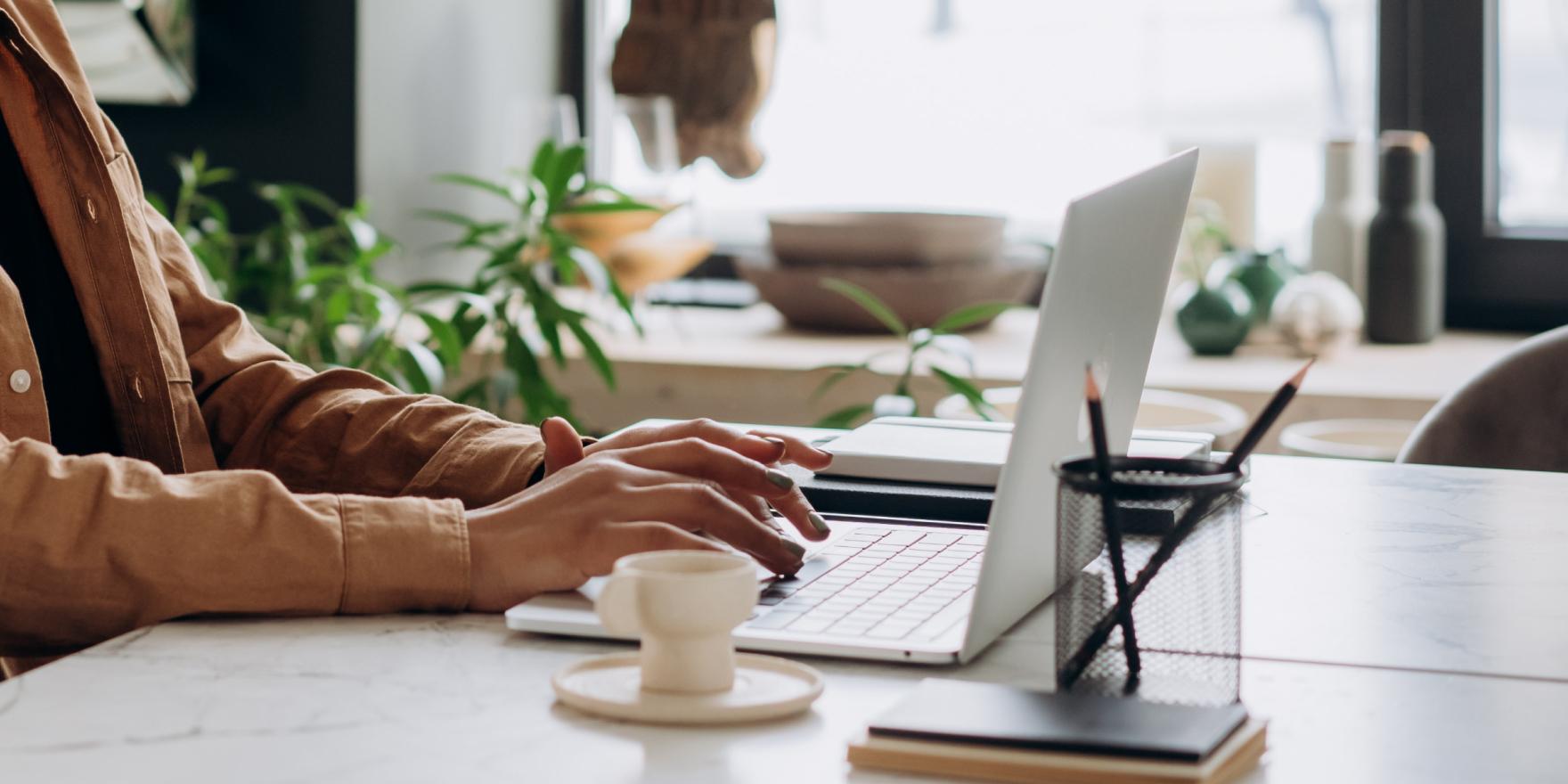
AI still needs a supervisor
As a data engineer, I work with large volumes of data on a daily basis, and I find ChatGPT and other AI agents based on large language models (LLMs) helpful for generating code snippets and sample files. Not without help from humans, though: As AI lacks context awareness and familiarity with specific data, it’s prone to errors and inaccuracies that could disrupt your entire project. That means you have to spend significant time and effort writing and fine-tuning a prompt.
Plus, real-world AI and ML datasets are quite heterogeneous and fragmented. And while machines can assist in data cleaning, only data engineers can trace the entire pipeline from start to finish and spot potential issues.
Another catch: Even if you like AI output, the system won't spill the beans on how it came up with the idea, which makes debugging and customization a real head-scratcher.
Nina Selezneva, Senior Data Engineer
The rise of the 'prompt engineer'
AI technologies can free up developers to work at a higher, more abstract level and focus on problem-solving and solution design. In an ideal scenario, AI could assist engineers just as calculators help mathematicians perform complex calculations.
AI's evolution will be driven mainly by advancements in natural language processing (NLP) advancements and computer vision. And as the performance of any AI model heavily relies on the quality of the prompts they receive, prompt engineering (read: enhancing AI instructions) will be another critical component shaping the future of AI.
Andrew Ng — AI trailblazer and co-founder of Coursera — has recently launched a signature course in prompt engineering, so the art of communicating with AI may soon become one of the most sought-after technology jobs.
Tsimur Kakhanovich, ML Engineer
ChatGPT for computer vision is on its way
My work revolves around helping businesses automate specific, routine parts of their operations. But it’s a bummer that AI's automation capabilities in computer vision are still pretty limited. Gathering high-quality visual datasets is hard to automate, as it requires a lot of input from human experts and a vast amount of universal human knowledge — resources even tech titans have yet to secure. Besides, high-dimensional images used in image-based models demand substantial computational power and memory capacity.
There’s work similar to ChatGPT underway, however, that would develop comparable visual models for computer-driven image recognition.
Existing visual datasets aren’t diverse enough for training yet, but we may soon witness a ChatGPT equivalent for computer vision — and that’s when ‘real’ artificial intelligence will come to life.
Victoria Davydovich, ML Engineer
AI copilots propel productivity to new heights
I’ve experimented quite a bit with Github Copilot and Microsoft 365 Copilot, and I can see why they’re considered the most advanced AI-powered code completion tools. These programs suggest code, write snippets, and draft entire functions and methods — all in real-time.
Sounds promising, yes, but keep in mind that ML models are inherently probabilistic, so there’s no guarantee that the suggestions provided are 100 percent accurate.
Also, most software developers would agree that reading someone else's code is just as challenging as writing it from scratch. So, the need to proofread AI-generated snippets and verify the existence of the API used for code generation adds another layer of complexity.
Still, in a year or two, I'm betting nearly all engineers will hop on board with AI copilots due to their time-saving nature and ability to spark initial project ideas and foster creativity. AI software will continue to improve, but it’s unlikely to be based on the current iteration of LLMs: They don't play nice with the strict rules of programming languages.
Andrei Popov, Group Manager
AI democratizes access to quality data analytics
While it’s true that AI tools often give low-quality responses due to the lack of context in the request, some tools have already made good progress in incorporating contextual data directly from data structures in the pipeline, which significantly boosts prediction quality. AI code-writing assistant Sketch is a good example.
Junior Python engineers can ask a few questions about their data, receive remarkably good code in response, and actually use it, which would have been unimaginable just six months ago. This breakthrough could revolutionize the entire industry, allowing specialists with limited-to-no Python or SQL skills to seamlessly perform their own data analytics autonomously.
What’s more, AI excels in taming tabular data. With code assistants by your side, tasks like tidying up data and conjuring up eye-catching charts, maps, and histograms become an absolute breeze.
Imagine another scenario: A data analyst with limited knowledge of the subject matter has just joined a project. That’s not a problem anymore, because you can effortlessly retrieve highly accurate explanations and descriptions of data elements or entire data frames by querying AI APIs. Of course, LLMs are not a single source of truth, but integrating these tools saves a lot of time for the whole team.
Kirill Lepchenkov, Data Science Engineer
Have AI-nxiety? AI is an opportunity, not a threat
AI is often compared to the Industrial Revolution for a reason: Just as machines freed humans from manual labor, AI assists in uncovering hidden patterns, simulating scenarios, and predicting outcomes, which will lead to even more groundbreaking discoveries in major engineering disciplines in the future.
However, automation of some parts of the code-writing process and accelerated delivery mean not only considerable productivity gains, but also technical debt. Lots of it. This calls for adapting existing methodologies around how developers can work in tandem with the new tools’ capabilities.
AI will also elevate the standards of engineers' expertise. With AI taking over common tasks, engineers will need to consistently expand their knowledge to complement and enhance the capabilities provided by AI solutions. But even so, remember that we made AI, and not the other way round, so treat it as a reliable partner and not as an enemy.