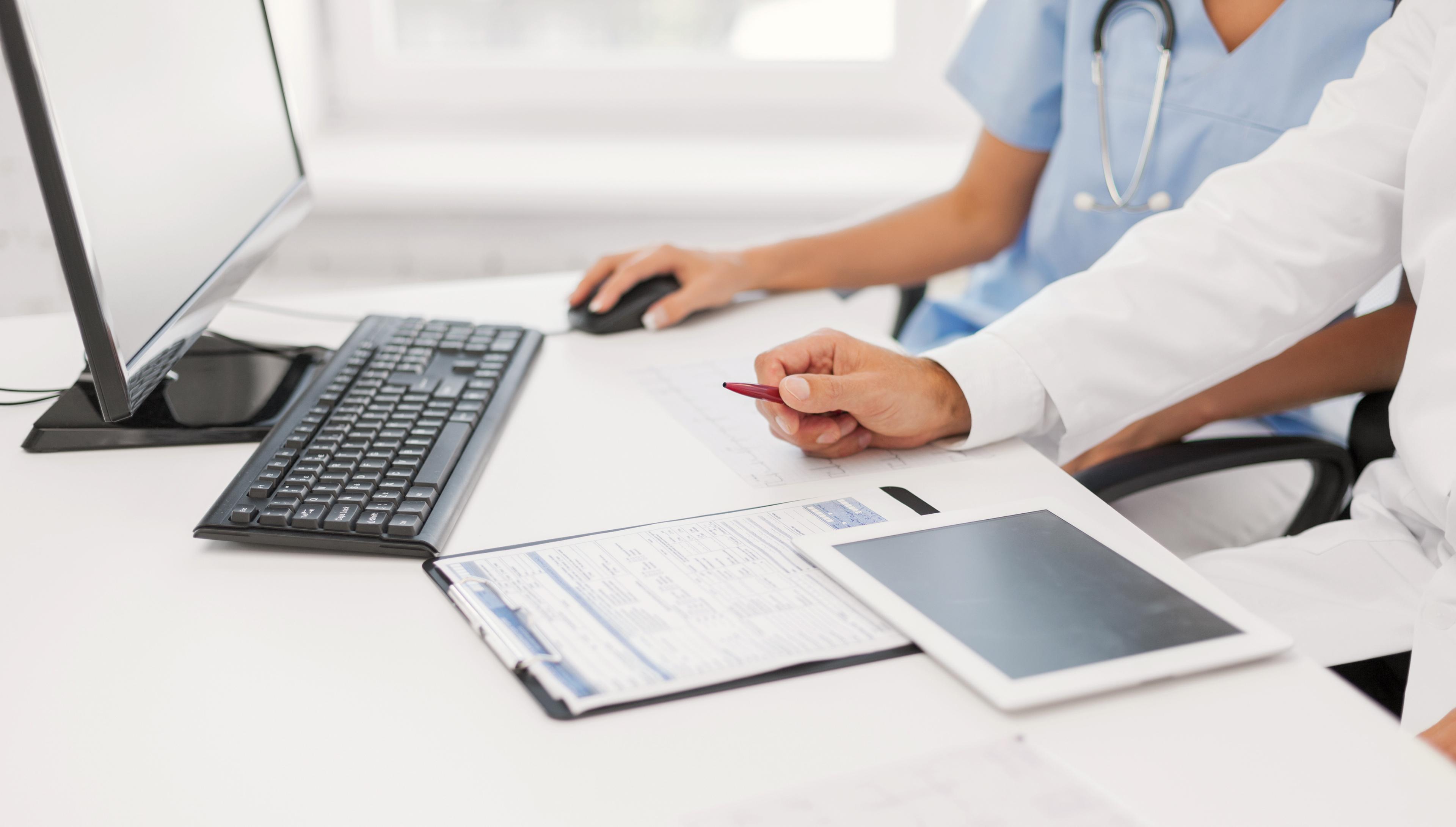
How big data is transforming healthcare
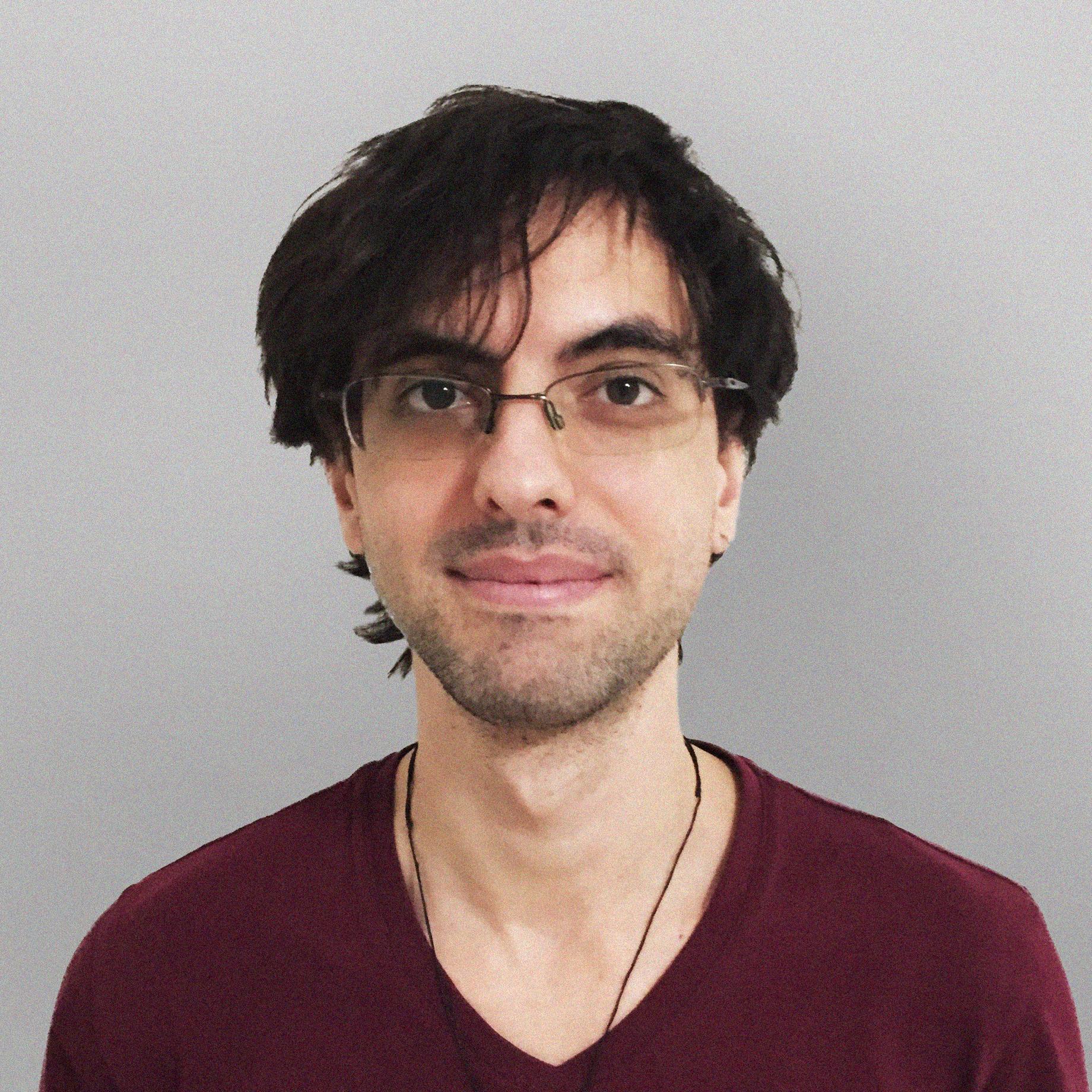
Any beating heart is a potential source for a constant stream of data. Yes, including your heart, right now. And as with any data source, every thump and bump has value for those who know how to use it.
That's why, in recent years, the healthcare industry has developed a plethora of wearables, smart devices, and apps designed to keep us healthy by delivering those bodily bits and bytes on a massive scale. Big data in healthcare had momentum before COVID-19, but in the post-pandemic world, the industry's appetite for the technology has generated enormous opportunities for both startups and established enterprises.
While the methods to collect, analyze, and employ big data differ from industry to industry, healthcare is unique in its goals of directly improving, or even saving our lives — goals that everyone, including both those motivated by profit and those who aren't, can get behind. Big data applications in medicine are immense: Recent market research shows that in 2021, the market reached a value of $21B, and it's projected to reach $79B by 2028. That reflects a CAGR of nearly 30%.
If the overall aim of collecting that data is to keep our hearts beating longer, the data itself has proven to be the vital spark of the modern healthcare industry: Increasingly, data drives ROI in tech, and with big data experts, the returns become exponential.
Where does big data come from in healthcare?
Big data is acquired by collecting and processing an astonishing amount of health-related minutiae that comes from countless sources, including daily-use smartphone apps and specialized devices in healthcare facilities.
Companies that rely on big data then analyze the data gathered and convert it into actionable insights down to the individual level, helping hospitals and doctors to provide better services and patients lead healthier lives.
That's how big data is helping hospitals cut costs by reducing the need for ER visits; it also helps medical professionals deliver efficient, affordable care to more people while empowering patients to boost their health by themselves through lifestyle changes.
Some major big data sources in healthcare include:
Electronic health records (EHRs)
EHRs are fundamental for doctors to access their patients' medical history. However, when analyzed in bulk (and always anonymized due to HIPAA and GDPR), EHRs offer vital information about the effectiveness of clinical trials, disease prediction and prevention, immunity rates from specific pathogens, and demographic indicators including age, gender, and income.
Medical devices and imaging
Medical devices are perhaps the most crucial source of big data when it comes to predictive analytics. The sheer amount of data that smart healthcare devices generate can be correlated with multiple sources, such as EHRs, to anticipate individual patient conditions and risks and then recommend best courses of treatment accurately.
Wearables
The popularity of fitness trackers, smartwatches, and even basic pedometers has led to consumer obsession with personal health monitoring, with over 25% of adult Americans owning smart wearables. Together with apps, the data collected and analyzed by these devices provides users with an easy way to track and improve their daily health habits and head off various diseases.
Genetic research
Big data plays a key role in genomics, a market poised to be worth over $100 billion by the end of the decade. Services such as Ancestry DNA and 23andMe generate data analytics for healthcare that can be used to better understand and fight hereditary diseases. Big tech companies have noticed: Investors such as Microsoft and Google helped set record-breaking funding rounds for DNA research startups, including the recent $200 million financing for cloud-based biomedical software provider DNAnexus.
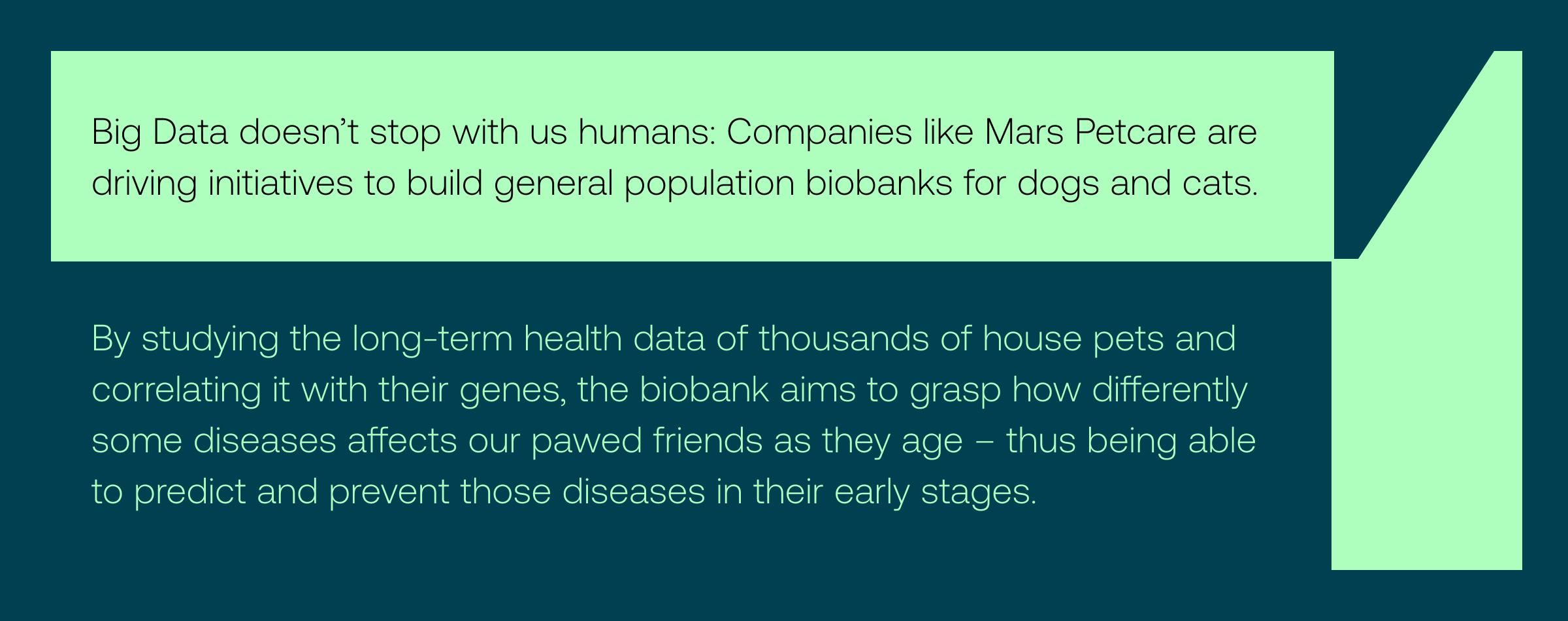
Primary big data applications in healthcare
Every relevant piece of information matters in big data analytics. Even when a patient is completely healthy, their Little Data is still crucial in the big picture.
This collection of data can then be employed to improve:
Speed of diagnosis
A correct, fast diagnosis paves the way to successful treatment. Big data empowers doctors to reach accurate diagnoses by cross-referencing huge amounts of medical information, narrowing a particular case down to the most probable conditions. For example, an image of a patient's eye can be rapidly compared to masses of similar (and already classified) images to zero in on a probable diagnosis such as macular degeneration.
Preventative care
Most major stakeholders in healthcare, including insurance companies and especially patients, would rather prevent than cure. When leveraged efficiently, the individual characteristics of a patient's data — genetics, biometry, pre-dispositions — can be paired with a general demographics database to predict (and prevent) future medical conditions and diseases.
Precision medicine
The more doctors understand a patient's condition, the more spot-on treatment will be — down to the individual level. Big data enables healthcare professionals to eschew the one-pill-fits-all approach and prescribe fully personalized treatments according to the patient's genetic traits, medical history, age, and disease. At their best, these treatments can be tweaked to combat side effects and improve a patient's response to them.
Resource optimization
Big data analytics in healthcare can also improve management within the industry. Hospitals, for instance, can tap into big data to anticipate which hours and days patients are more likely to need their services and scale accordingly.
What can we expect from big data in healthcare tomorrow?
Big data isn't the only modern piece of tech becoming commonplace in hospitals and care centers. AI, machine learning, IoT, and blockchain are constantly mixed and matched with big data analytics to engineer new — and in many cases, revolutionary — use cases for healthcare.
Machine learning-fueled predictions
If big data is revolutionary in the ways data is acquired, machine learning (ML) is the counterpart in data processing. Algorithm-based predictive models execute data analysis and learn from big data's enormous vault of possible scenarios to anticipate the unexpected, minimizing human errors in situations where these might not be obvious — like the chances that COVID-19 patients will suffer respiratory failure or during triage assessment after a natural disaster.
Imaging analytics
ML algorithms specialized in visual pattern recognition can sift through big data repositories to assist in the diagnosis of diseases without the need for invasive procedures. At scale, these techniques reduce treatment costs and improve the healing chances of time-sensitive conditions such as tumors.
IoT integration
Wearables are often IoT's first touchpoint for consumers and patients, but their potential extends far beyond wristbands. Every year, millions of IoT medical devices ranging from diabetes meters to hospital apparatus (think heart monitors and ingestible sensors) are manufactured to take advantage of big data and improve the decisions made by healthcare professionals.
Telemedicine improvement
Online healthcare has been around for a while, but big data analytics and AI have vastly improved its scope and efficacy. Patient records, symptom analysis, and demographic data all play a part in improving the information available to doctors for better results — coupled with the data-collecting wearables and smartphone apps.
Big data dashboards
Teams use dashboards to interpret data and communicate better, as well as to monitor ups and downs in performance and other metrics. Big data dashboards can do the same in healthcare; their metrics of interest are individual patients' clinical data and the facilities' organizational capacity. These dashboards can track staff numbers and ratio to patients, costs per department, patient satisfaction levels, and other KPIs.
With the steady adoption of these interactive big data dashboards by healthcare facilities, doctors and nurses become better equipped to gauge an individual patient's situation as well as the facility itself — holistically and by the minute. This translates into faster reaction times to head off emergencies and improved recovery times.
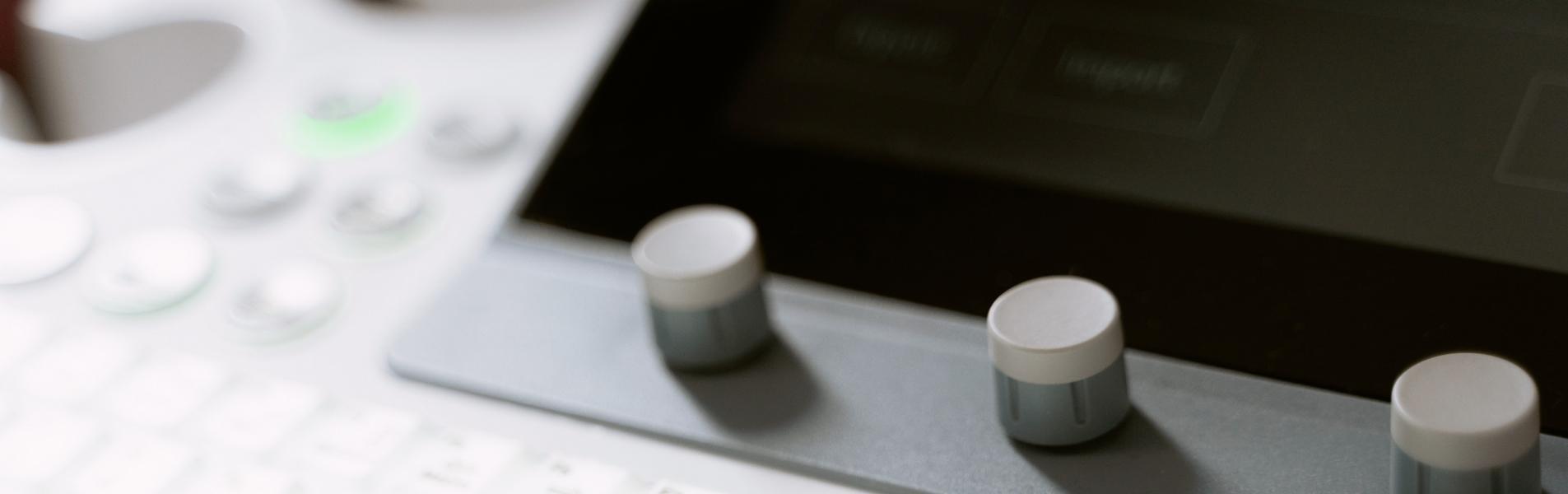
Finding the way forward
Managing big data, however, isn't a trivial task. While the technology evolves by leaps and bounds every year, there are challenges to be taken into account when wrangling big data in healthcare.
Data security and privacy
Privacy concerns are relevant no matter the industry segment, but those come with an additional layer of relevance in healthcare: Few things are more personal than one's body and genetic information. Healthcare providers, in particular, accounted for over 72% of all healthcare-related data breaches in the second half of 2021 — courtesy of wrongdoers taking advantage of outdated or insufficient security protocols to perform ransomware attacks.
Encryption, as always, comes into play as the first line of defense against criminal exposure, aided by HIPAA-like mandatory privacy compliance that all healthcare app developers must abide by. Anonymization also plays a key role in ensuring the patients right to privacy by treating their health data as pure statistics, making it impossible to single out individuals. And, more recently, even blockchain technology has made strides toward the prevention of patient data leaks.
Data storage and priority
Storage is a perennial topic when it comes to big data, but it comes with an extra layer of gravitas in healthcare thanks to privacy issues, yet again.
Large enterprises might prefer to store their data in the cloud — it's flexible, cheaper, easily scalable, and safer in the face of catastrophes like floods and fires. But different countries have different privacy laws, some of which require that their citizens' data be physically stored in their territory. This forces companies to adapt their data storage logistics and, if they choose on-premise servers, to invest in personalized security solutions to protect that data.
How data is prioritized also matters: Smokers, for example, generate relevant data for both dentists and pulmonologists, but for different uses and with different priorities. While it may be somewhat optional for a dentist to know that his patient carries the "smoker" data tag, it is absolutely mandatory for pulmonologists to do their job properly. Therefore, establishing parameters for the importance of data depending on how it will be used greatly helps its future stakeholders.
Data cleansing
Data-based results and insights are only as good as the data's quality. As such, data cleansing — ensuring the data's accessibility, relevance, accuracy, and redundancy across sources — is paramount to generating usable results.
Beyond zeroes and ones
Healthtech is a business, just like any other. But it's set apart from other industries because it strikes at the core of our needs as humans. It's personal, urgent, and particularly vulnerable to bad actors.
A heavily-regulated healthcare industry isn't a bad thing. Instead, when properly deployed these protections direct the creativity of entrepreneurs towards the development of ethical (and profitable) solutions. Here, big data may play a part in solving logistic challenges taxing our healthcare systems today, for instance, by increasing the data interoperability among healthcare providers or public and private entities. Of course, it needs to do so while protecting the general public against multiple forms of health data exploitation.
Since tech historically advances in larger strides than legislation does, all stakeholders bear responsibility for ethical healthtech development — including big data service providers. With novel use cases emerging on a constant basis to push the boundaries of experimentation, the rightful application of big data in healthcare is bound to make our hearts, literally and otherwise, beat better.