Computer vision in healthcare
The fundamentals of computer vision in healthcare
Computer vision is a subset of artificial intelligence that enables computers to interpret and derive meaningful insights from visual input. By combining machine learning and neural networks, computers can detect anomalies in medical images and other visual data, leading to actionable recommendations or decisions.
In medicine, computer vision proves beneficial by automating regular tasks like scan categorization and tissue differentiation, reducing the workload on healthcare professionals, and minimizing patient wait times.
Essential techniques behind computer vision
Just like any other AI-enabled technology, computer vision features a range of behind-the-scenes mechanisms that do the trick. But despite their black-box nature, computer vision algorithms are no magic: They need diverse, high-quality training data to recognize various objects and scenarios.
Machine learning and deep learning
Both are pivotal technologies at the core of all computer vision models. ML algorithms enable machines to improve with experience and identify unknown images accurately, while deep learning empowers computer vision models to analyze visual data in a human-like manner.
Image recognition
Computer vision applications allow machines to identify objects, attributes, and other variables in images or videos, serving as a fundamental task often combined with image classification and object detection.
Object detection
This specialized subset of computer vision locates and identifies objects within an image and draws a bounding box around their perimeter. Object detection is a go-to technique for medical imaging tasks like surgical planning and image-guided therapy.
Segmentation
Segmentation refers to partitioning an image into multiple segments or pixels to simplify its representation and facilitate further analysis. This technique is vital in object recognition, tracking, and medical image analysis tasks.
Pattern recognition
Pattern recognition is employed to detect consistent patterns or repetitions in visual datasets. This technique gives computers human-like recognition intelligence, offering essential tools for healthcare analytics tasks.
Feature extraction
Feature extraction identifies and extracts relevant features and important patterns from raw data. It also reduces data complexity and prepares visual input for classification, prediction, and clustering tasks.
Edge detection
As an image processing method, edge detection identifies the boundaries of featured objects. This is instrumental to image segmentation and data extraction tasks, as well as helps reduce the amount of data and speed up the computer vision workflow.
3D vision
3D vision enhances computer vision by enabling the perception of depth and three-dimensional structure from 2D images, addressing challenges in spatial understanding and lighting variations.
How computer vision works, step by step
In a nutshell, computer vision replicates the complexity of human visual perception. But here’s a twist: Thanks to multiple smart technologies under its hood, computer vision technology in healthcare often beats expert physicians at diagnosis accuracy. Here’s how:
Image acquisition
It all begins with collecting an image or video sourced from cameras, sensors, or other hardware. The quality of these images is critical to computer vision success, as any degradation can impact system performance.
Preprocessing
Preprocessing removes unwilling distortions from the image, enhancing its overall quality. This process involves resizing, cropping, denoising, and image filtering. This stage aims to prepare image data for further analysis and reduce computational complexity.
Feature extraction
Here, key features like edges, shapes, and textures are isolated from the preprocessed image. These features form a more informative dataset, enhancing the accuracy of the subsequent analysis. Feature extraction also reduces the data's dimensionality and increases the learning speed for an ML algorithm.
Detection/segmentation
Depending on the application requirements, the workflow continues with either the detection or segmentation process. Detection identifies and locates objects within the image, often using bounding boxes to highlight detected objects. Segmentation is more granular — it divides the entire image into segments or pixels that belong to different objects or classes.
Classification
At this stage, the algorithm classifies the detected objects or segments into predefined categories. Classification uses the features extracted earlier to assign a category to objects. The accuracy of classification relies heavily on ML or deep learning models that have been trained on large datasets.
Object recognition
The model then matches representations extracted from the visual image with those stored in the database. From a tech standpoint, object recognition combines classification, tagging, detection, and segmentation tasks, enabling the model to interpret the input and accurately identify ground-truth object locations.
Post-processing
After the initial interpretation, the results might undergo post-processing to refine the outputs and discard superfluous bounding boxes. Post-processing also helps the model filter out false positives and leverage additional context to improve accuracy.
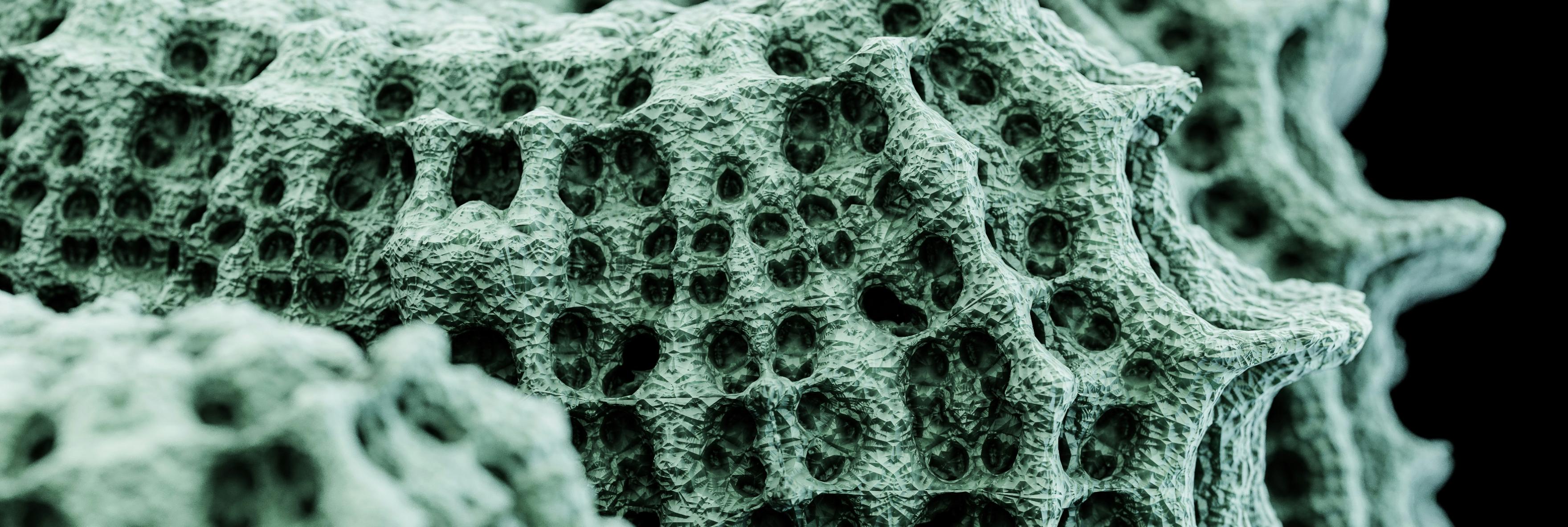
Need unparalleled computer vision expertise?
Top seven computer vision applications in healthcare
The vast potential of computer vision unfolds in the data-intensive realm of healthcare, covering medical diagnostics, surgical interventions, and remote care.
Medical image analysis
Computer vision algorithms comb through X-rays, MRIs, CT scans, and ultrasound images to detect anomalies and early-onset diseases faster than human practitioners. AI-enabled image analysis improves diagnostic accuracy, enhances patient experience, and reduces the time required for interpretation.
Surgical assistance
In robotic surgery, computer vision provides real-time imaging, aiding surgeons in executing procedures with enhanced accuracy. It offers detailed views of difficult-to-access areas, improving surgical precision and contributing to quicker patient recoveries.
Pathology and histology
Computer vision techniques allow for tissue interrogation methods that were unavailable before. By analyzing the shape, size, and distribution of cells and tissues, smart algorithms can identify patterns that indicate specific conditions, including cancer, helping clinicians make accurate diagnoses with less manual effort.
Radiology
The application of computer vision in healthcare is also becoming increasingly common in radiology, where it helps track a patient’s condition progression. The algorithm compares images against an extensive database and predicts the development of conditions based on subtle changes.
Ophthalmology
Computer vision makes the diagnosis of retinal diseases and eye development anomalies less invasive and more accurate. Algorithms can detect early-stage diabetic retinopathy, glaucoma, and age-related macular degeneration, enabling doctors to intervene early.
Telemedicine
In tandem with telemedicine applications and wearables, computer vision can transform virtual care delivery, augmenting traditional apps with advanced capabilities like remote medical picture analysis and vital sign monitoring. This empowers physicians to conduct medical exams and detect conditions remotely, making healthcare more accessible.
Pharmaceutical research
Computer vision has also found its way into drug discovery and development. Machine vision helps researchers automate the analysis of cellular images to identify potential therapeutic candidates, streamlining the drug development process and reducing market entry costs.
How healthcare benefits from computer vision
Here are the unique benefits computer vision brings to the medical table.
Improved accuracy and early disease detection
Computer vision can boost the accuracy of the diagnosis process by up to 99 percent, enabling early detection of life-threatening conditions. Timely detection enhances the efficacy of treatments, mitigates complications, and literally saves lives.
Increased operational efficiency
Computer vision takes a lion’s share of weight off healthcare professionals’ shoulders by automating medical data analysis. It also speeds up diagnostic processes, saving physicians more time for complex cases.
Enhanced patient care and monitoring
The application of computer vision in the medical field enables continuous, non-invasive patient monitoring. This enhances the overall care quality, especially in critical and chronic care scenarios.
Surgical precision and reduced complications
Computer vision equips surgeons with advanced visualization tools to execute accurate, minimally invasive surgeries. This support enhances patient outcomes, fewer surgical complications, and faster recuperation periods.
Accessibility and scalability of healthcare services
In tandem with telemedicine, computer vision applications bring high-quality care to rural and underserved areas. Also, the inherent scalability of AI technologies allows healthcare organizations to deliver services at scale.
Cost reduction and personalized medicine
Early diagnosis and automated data analysis reduce the financial burden on healthcare systems. The technology also supports the development of personalized treatment plans, ensuring that patients receive care tailored to their specific needs.
Computer vision in healthcare: challenges and considerations
Medical computer vision offers promising avenues for enhancing the management and diagnosis of chronic illnesses. However, as with any AI-driven tool, its widespread adoption in healthcare faces regulations and data management hurdles. It's essential to align certain elements before diving in.
Challenge
Consideration
Data privacy and security considerations
In healthcare, computer vision applications collect, process, and share highly sensitive medical data, including diagnoses, treatment guidelines, and personal health data. This increases the odds of data breaches and misuse.
Establish a secure infrastructure with stringent data protection measures. Implement data guardrails to ensure adherence to relevant healthcare regulations such as HIPAA and GDPR.
Ethical considerations in automated decision-making
Given the limited explainability of deep learning, implementing computer vision in healthcare leads to ethical dilemmas, including potential biases, inaccuracies, and unclear accountability for automated decisions.
Regulatory bodies, healthcare providers, and patients should be in the driver’s seat when it comes to AI-generated decisions. Algorithms must also meet ethical and professional standards.
The need for robust datasets to train AI models
Computer vision technology in healthcare feeds on high-quality annotated medical imaging data to recognize patterns and abnormalities. But due to the privacy concerns, data silos, and the diversity of healthcare standards, this data is hard to obtain.
A united effort from healthcare entities is necessary to assemble extensive, anonymized datasets representing diverse demographics and health conditions.
Challenging integration with existing healthcare systems
The existing healthcare IT landscape is a complex governance structure full of silos. Smart algorithms might lack compatibility with current systems and require prior staff training.
Adopt easily customizable computer vision systems using a collaborative, phased approach. The post-adoption stage should be followed by comprehensive staff training and support to maximize the benefits of the technology.
Computer vision use cases in healthcare
Over the last few years, computer vision has proved to be a transformative force that pushes the boundaries of traditional care and brings it beyond clinical settings. Some computer vision healthcare companies were faster than others to jump on the bandwagon of AI-enabled care and reap the early benefits.
A true heavy hitter in the world of AI, SenseCare is a smart health platform developed by SenseTime. It integrates AI algorithms with advanced image processing to facilitate diagnostics, treatment planning, and recovery in various medical specialties.
SigTuple offers innovative screening technologies that combine microfluidics, robotics, AI, and cloud computing for advanced diagnostics. Their technology, Shonit™, automates differential counts in blood smear analysis, aiming to revolutionize medical diagnostics through AI-powered analysis of visual medical data.
Pixee Medical is developing a new generation of computer-assisted surgical navigation solutions powered by augmented reality. The solutions assist orthopedic surgeons in performing total knee arthroplasty, which enhances the accuracy and affordability of computer-aided surgical solutions.
Oxipit offers a suite of chest X-ray image solutions for global medical imaging. Their flagship solution — ChestEye Quality — supports diagnostics of the 75 most common radiological findings, reducing the error rate and allowing clinicians to make informed decisions amidst uncertainty.
Iterative Health is spearheading real-time computer-aided detection and diagnostic tools in gastroenterology. Their proprietary imaging solution aids in detecting and categorizing lesions that often go unnoticed by the human eye.
What does the future hold for medical computer vision?
The computer vision in healthcare market is on an upward trend, projected to reach $4.28 billion by 2030 from $1.16 billion in 2024. And though the path forward tends to be bumpy, the technology adapts rapidly to the unique challenges of medical imagery.
With innovations such as multiple instance learning, 3D convolutions, and spatio-temporal models, computer vision is now geared up to address the specific needs of medical data analysis. More importantly, regulatory bodies have warmed up to the technology: The FDA has approved dozens of AI/ML-enabled medical devices and algorithms across many medical subspecialties, indicating potential for wider adoption ahead.
The outlook for 2024 shows that computer vision will continue to expand and innovate within medical fields, integrating further across diverse modalities.
Meet Vention, your go-to provider of computer vision solutions in healthcare
Vention develops powerful and secure computer vision systems that enable advanced imaging analysis to aid diagnosis, treatment, and patient care. Tailored for the ever-evolving healthcare sector, our solutions enhance care outcomes, optimize healthcare workflows, and drive innovation in robot-assisted surgery, precision medicine, and intelligent asset management.
Our team offers deep insights and strategic planning to ensure your technology fully meets clinical needs and operational requirements.
We create customized healthcare applications that integrate seamlessly with your existing systems to improve functionality and efficiency.
Compliant and robust
Tech-savvy computer vision solutions for healthcare
No matter the complexity of your challenge, our developers possess the necessary tools, technical expertise, and industry knowledge to successfully execute your project, crafting competitive computer vision solutions with exceptional accuracy.
Deep learning
Our developers use popular deep learning frameworks such as TensorFlow and PyTorch to facilitate complex model building and training. This helps us achieve greater accuracy in image classification, semantic segmentation, object detection, and simultaneous localization and mapping (SLAM).
Image processing
Our experts leverage the OpenCV library to solve the challenge of real-time image processing, employing the ITK toolkit for crafting advanced systems tailored to medical image analysis.
Neural network APIs
Vention’s AI team chooses Keras to build complex, fast, and easily deployable deep learning models — in just a few lines of code — that run across mobile, browser, and embedded systems.
Data annotation
Platforms like Labelbox and V7 Darwin provide tools for annotating medical images, which is essential for training accurate AI models. The result is a streamlined data labeling workflow that saves time and effort.
Cloud services
We set up a scalable and secure cloud infrastructure using AWS or Google Cloud to facilitate the training and deploying of AI models and enable healthcare data management at scale.
Computational platforms
By using thousands of NVIDIA CUDA cores on a GPU, our developers enable faster processing of large datasets and facilitate tasks like face recognition, image manipulation, and 3D rendering.
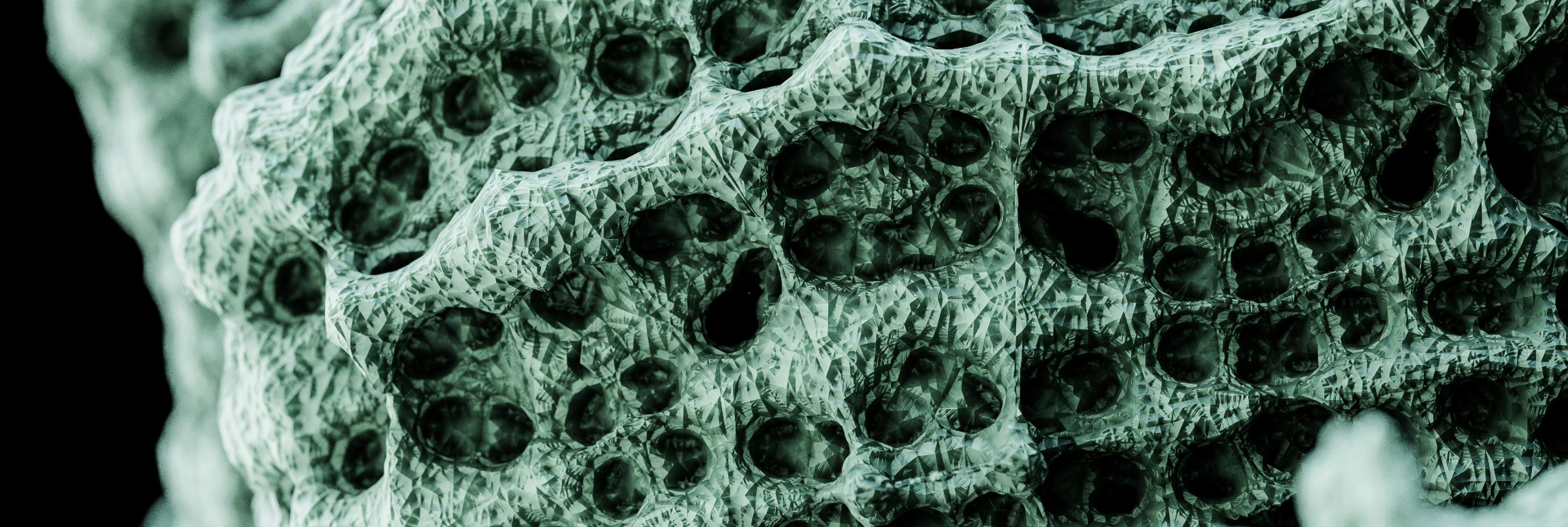