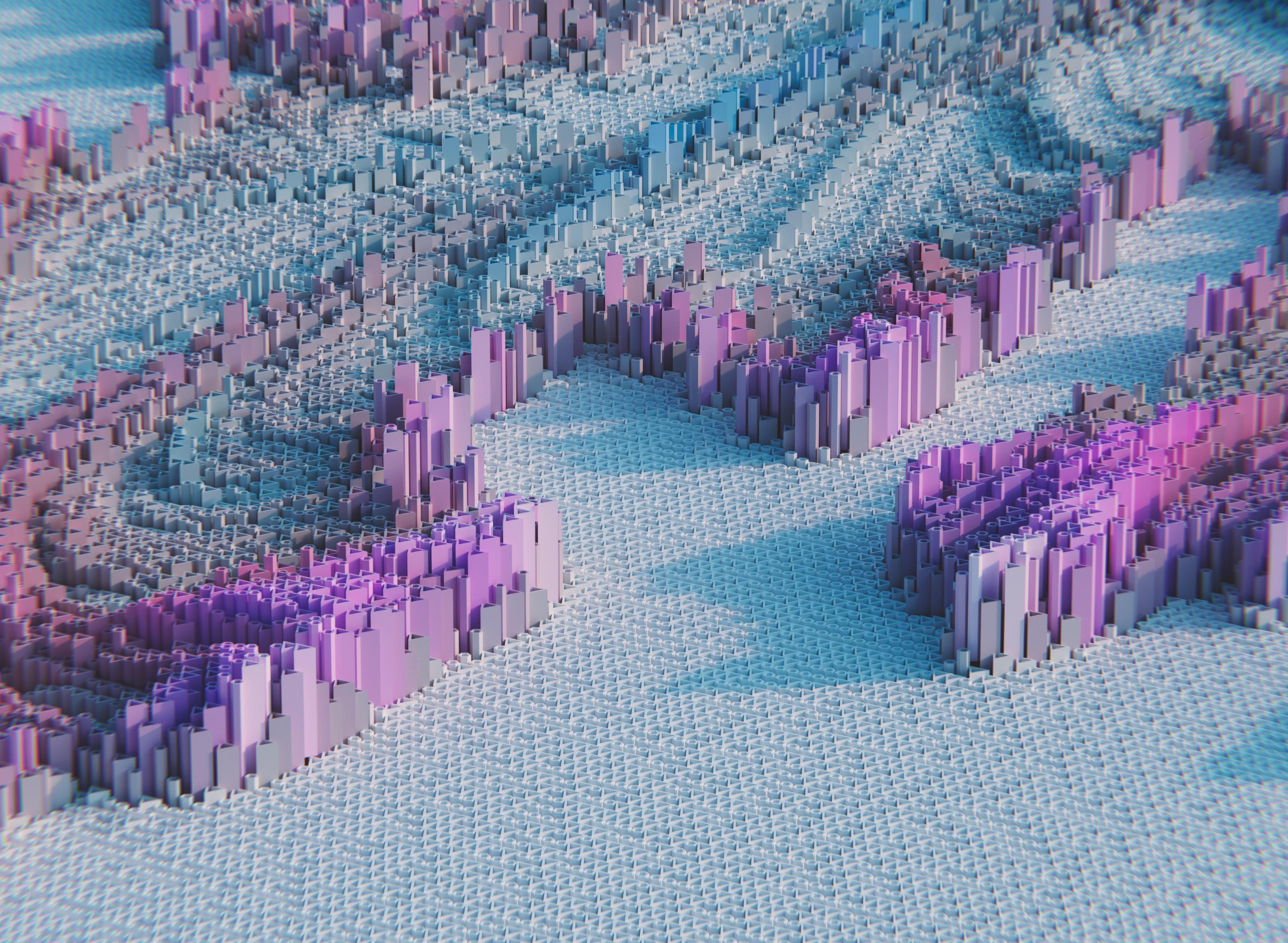
Machine learning in fintech and why it matters
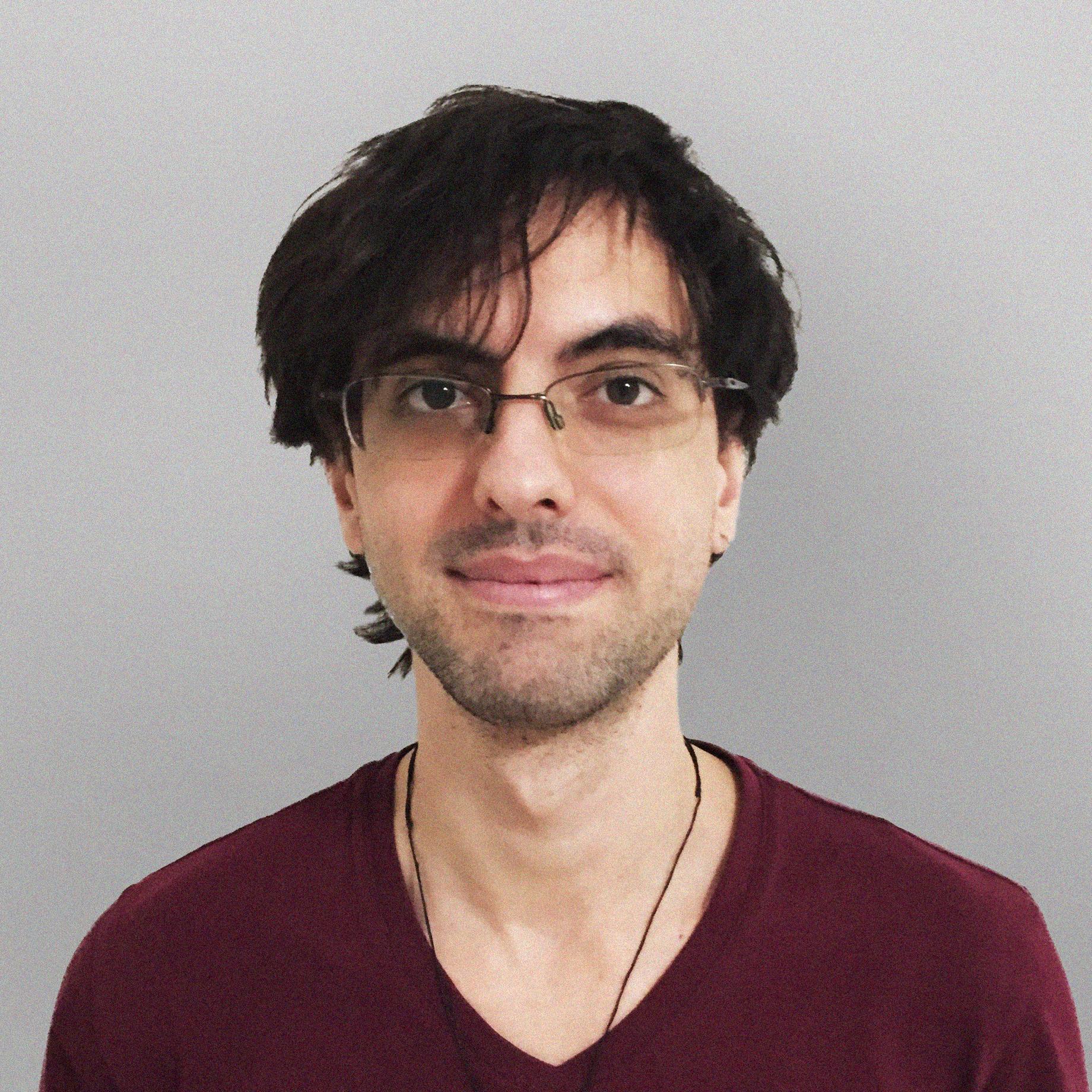
Key takeaways:
-
Machine learning in fintech is not just a temporary trend; it's a fundamental force driving the industry, with a projected market growth from $158B to $528B by 2030.
-
ML is transforming challenges into profitable opportunities across various fintech verticals, improving everything from fraud detection to algorithmic trading and customer service support.
-
As noted by Andrei Papou, Lead AI Engineer at Vention, every key fintech company has already introduced or is actively developing AI solutions, meaning not following suit could be a strategic mistake.
In finance’s tech-driven world, where staying ahead hinges on efficiency and innovation, machine learning stands as the pivotal enabler. It revolutionizes essential aspects like risk management, fraud detection, algorithmic trading, and compliance, transforming challenges into profitable opportunities.
What are we hinting at, you ask? Easy: Integrating machine learning for fintech firms of today (banks, brokerage firms, insurance firms, hedge funds, credit unions, you name it) isn't just a strategic move, it's a fundamental shift to streamline operations, unlock growth, enhance customer satisfaction, and improve cost-effectiveness and ROI.
The market state of machine learning in fintech
In the year when artificial intelligence (AI) broke out to the mainstream with the loudest bang, it can feel like machine learning (ML) has been relegated to just a blip.
That, however, couldn’t be furthest from the truth. Generative AI itself depends on deep learning to be trained, which means that despite not sharing the spotlight, machine learning thrives in every single industry right now. And fintech is no exception.
Boasting a global market size projected to rapidly expand at an 18 percent CAGR, from US$158bn currently to a whopping $528bn in 2030, machine learning is one of the greatest assets for financial institutions to optimize their processes. And ultimately — as described in detail in our recent State of AI report — shave costs.
Our specialists at Vention know it all too well: Machine learning is one of our core AI-related disciplines, particularly in finance. From predictive analytics and big data to natural language processing (NLP), we follow market movements closely to stay on top of both the technical and the business side of machine learning in fintech.
“AI was always a favorite tool of developers to experiment with,” states Andrei Papou, Vention’s AI specialist. “The reason why it became popular among wider audiences is due to certain generative AI tools which, if you think for a moment, are actually generative ML models for computer vision and NLP. Their reverberation forced even the most conservative fintech companies to consider the advantages,” concludes Papou.
With 70 percent of all financial firms tapping into ML at the start of this decade, the high adoption rates of AI and machine learning in fintech reveal a clear pattern — it’s here to stay. Whether to increase their risk assessment effectiveness, automate manually intensive tasks, or just achieve better customer satisfaction, machine learning works.
Here’s why it does.
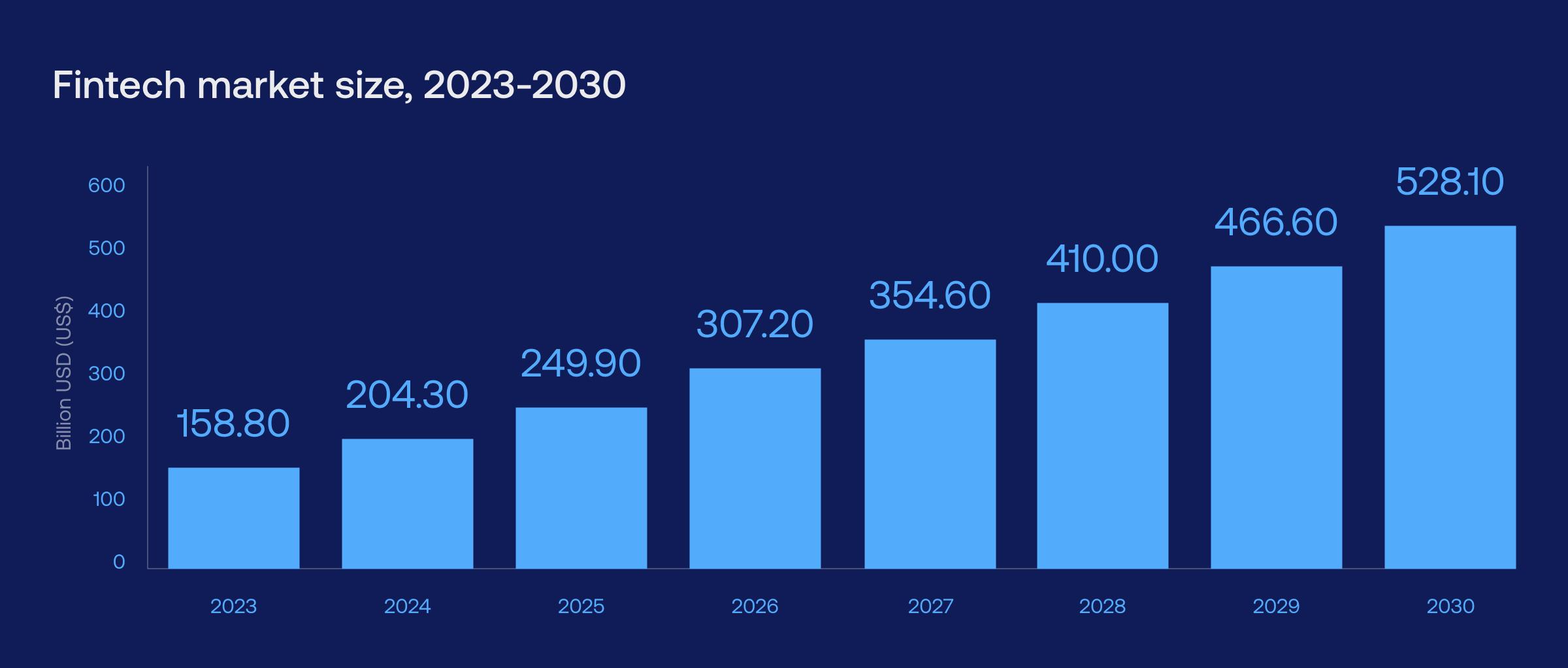
Source: Statista
Top 7 applications of machine learning for fintech
Go ahead and pick just about any vertical within fintech, and you’ll see that machine learning will serve it well. Insurtech? Yep. Tradetech? Sure. Regtech? Definitely.
That’s because machine learning is solving, or at least greatly improving, issues fundamental to the industry as a whole. Fraud, for instance, isn’t a problem limited to insurance or crypto. Nor is the need for robust regulatory compliance confined to selected regions.
No matter your market segment and business model, machine learning in fintech presents diverse solutions to turn worries into profits.
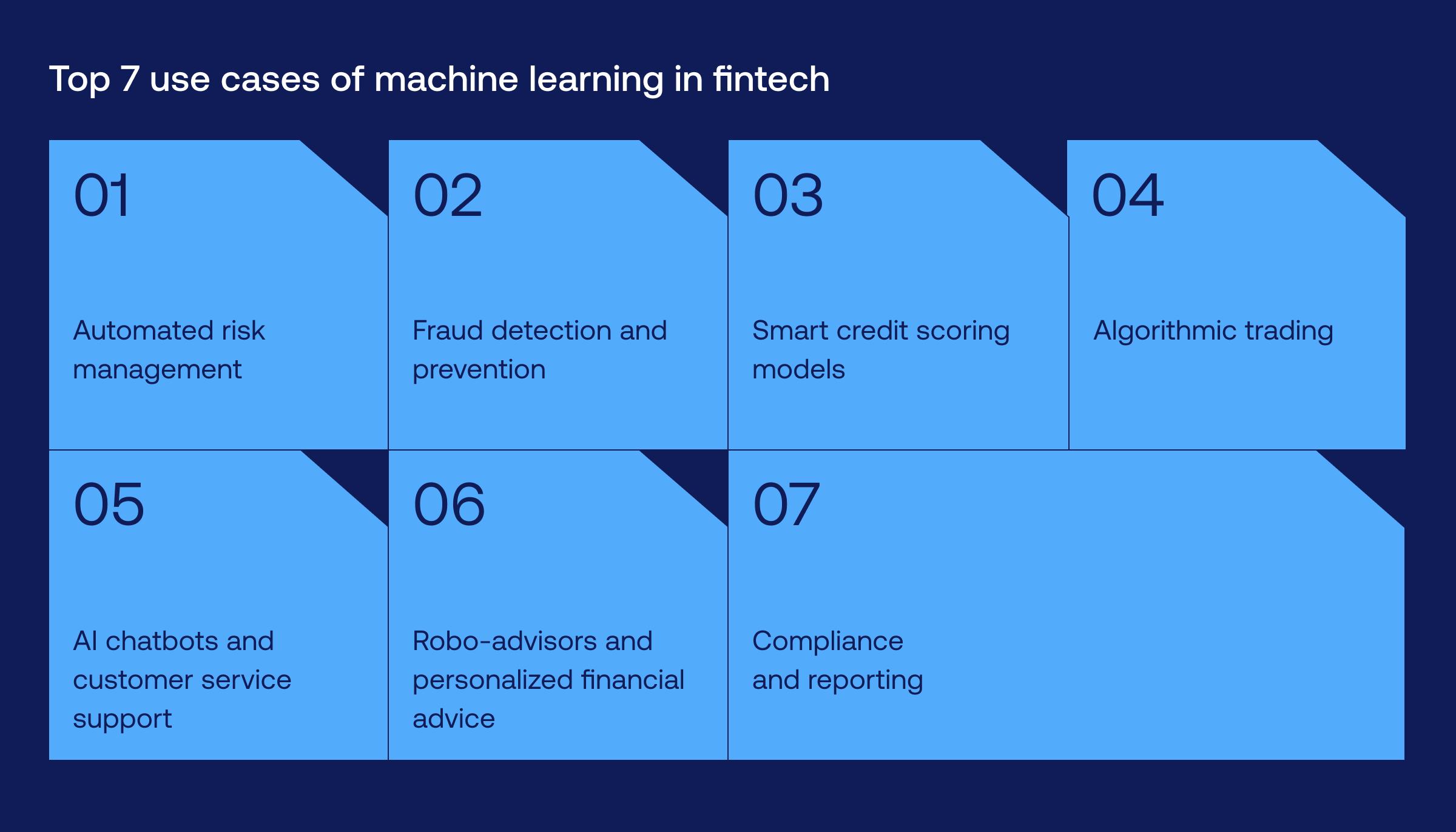
Risk management
Unlike conventional risk management, which often relies on manual analysis, machine learning algorithms can process vast amounts of data in real time, proactively identifying subtle patterns and correlations that human analysts might miss.
-
Market trends prediction – Machine learning models analyze historical market data to forecast trends so that financial institutions can make informed investment decisions and better manage market risks.
-
Operational risks and threats mitigation – ML in banking tools such as Santander’s Kairos can identify anomalies in operational and transaction data, helping detect and prevent fraud, cyber threats, and operational failures.
-
Client segmentation – By clustering customers based on their risk profiles, financial institutions can tailor precise risk management strategies to specific client segments and optimize resource allocation.
-
Collection strategies optimization – ML models can identify the most effective approaches to recover overdue payments all while minimizing customer churn and overall losses.
Fraud detection and prevention
In contrast to the static nature of traditional rule-based fraud detection, machine learning applications in fintech continuously learn and adapt to new scam patterns, which ensures better protection for your company’s operations and customers.
- Complex fraud pattern identification – Machine learning algorithms pinpoint complex fraud patterns through the analysis of vast datasets, identifying suspicious activities with a high degree of accuracy.
-
Real-time operability against fraud – As IBM demonstrates, ML already enables real-time fraud detection in up to 100% of transactions, allowing financial institutions to respond swiftly to potential threats and minimize financial losses.
-
Simultaneous detection – ML in fintech systems can simultaneously identify multiple types of fraud, such as payment fraud, credit card fraud, identity theft, and account takeovers, providing comprehensive protection against various threats.
-
Reduction of false positives – The accuracy of ML detection helps reduce false positives, relieving the burden of fraud analysts and streamlining their workload so that they can focus their efforts on the most relevant alerts.
Credit scoring
ML-enhanced credit scoring pairs up with big data to create an accurate picture of a borrower's risk profile, offering a more inclusive evaluation that reduces bias and opens up financial opportunities to a broader demographic range.
- Accurate credit risk assessments – Machine learning in fintech assesses credit risk with remarkable precision by analyzing a wide range of data, from traditional credit scores to alternative sources like social media activity and payment history, for more informed lending decisions and reduced default rates. (Check out how credit tracking agency FICO leverages AI and ML to build better credit risk models.)
- Faster loan approvals – Automated credit scoring models speed up the loan approval process significantly, making it possible to provide borrowers with quick decisions, which is crucial for personal loans and small business financing.
- Greater reach to unbanked prospects – ML helps extend financial services to underserved populations, a key business concern in developing countries, by enabling the evaluation of creditworthiness for individuals without a traditional credit history.
- Reduced lending bias – ML algorithms can mitigate bias in credit decisions by relying on data-driven objective parameters and reducing the reliance on subjective human judgment.
Algorithmic trading
Machine learning and fintech together create a perfect match for the realm of algorithmic trading. This mix allows for more efficient risk management and the potential for higher returns, setting it apart from the slower, more manual processes of traditional trading.
- Analysis beyond human possibility – Machine learning algorithms process and analyze vast amounts of market data at unattainable speeds by human traders, enabling quicker decision-making with accurate confidence levels.
- Support tool for traders – ML algorithms aid traders in identifying, assessing, and managing market risks so that they can make more informed trading decisions.
- Higher predictive power – Well-trained ML models greatly enhance the accuracy of trading predictions, improving the likelihood of profitable transactions.
- Adaptation to market conditions – Algorithmic trading systems adjust to changing market conditions, learning from past transactions to continuously improve their performance.
Chatbots and customer service support
In fintech, script-based chatbots are rapidly becoming a thing of the past. ML-based conversational systems understand and respond to customer inquiries more accurately and contextually, enhancing user experience and streamlining operational efficiency.
- Round-the-clock support – Chatbots powered by machine learning offer 24/7 customer support, reducing the operational costs associated with maintaining large service teams.
- Customer interactions management – ML chatbots efficiently handle a high volume of inquiries so that no request goes unanswered.
- Improved response times – Crucial to minimize those long and universally disliked wait times, chatbots can provide rapid responses to client queries to prop up overall customer satisfaction.
- Data collection on customer interactions – ML chatbots collect and cross-analyze different data points on customer interactions, offering valuable insights for improving products and services.
Robo-advisors and personalized financial advice
Robo-advisors are ML-fueled chatbot “cousins,” which continuously learn and adapt to both market changes and client needs, providing more accurate and personalized financial guidance.
-
Portfolio management – Robo-advisors use machine learning to build and manage investment portfolios tailored to individual risk tolerance and financial goals. Users can enter their present financial assets and expected income, set their goals (whether short- or long-term), and have their robo-advisors allocate the assets across investment opportunities according to the objectives defined.
-
Financial recommendations – Machine learning-driven robo-advisors can recommend financial products and services based on customer profiles and preferences. It allows users to receive personalized suggestions about savings, investments, and insurance solutions while eschewing the higher fees associated with personal financial advisors.
Compliance and reporting
Machine learning taps into efficient data processing and analysis to automate regulatory reporting and monitor compliance violations. It ensures that financial institutions always meet regulatory demands through comprehensive oversight capabilities, while also anticipating scheduled changes in legislation so companies can course-correct adequately.
-
Processing of regulatory reports – A huge boon in regulatory technology (regtech) applications, machine learning can read and learn from regulatory documents with unparalleled speeds. This allows for the automation of regulatory reports generation and reduces the time and effort required to ensure fintech regulatory compliance with financial regulations.
-
Monitoring for compliance violations – Moreover, machine learning can correlate the documents and guidelines they read. When paired with the continuous monitoring of financial operations, ML systems can automatically track and identify potential compliance violations as they occur, ensuring regulatory adherence and significantly reducing the compliance cost of financial institutions.
Ready to adopt machine learning, but not sure where to start?
Our expert consultants are ready to guide you.
How businesses benefit from machine learning applications in fintech
All of the above speak to where machine learning helps increase process efficiency and profitability, but what about how? If one had to condense the core benefits of AI in fintech into just a few objective bullet points, how could those benefits be best described?
Here’s a great summary:
Automation of repetitive tasks
Out of all machine learning benefits to fintech, automation is arguably the most noticeable on multiple fronts. For instance, ML algorithms can streamline customer onboarding processes to validate client information in real-time without manual input. Additionally, automating the reconciliation of financial transactions eliminates the need for manual data entry, saving both time and resources.
Automation also has more subtler benefits for the rest of your human team. Burnout can impact productivity in a way hard to quantify — and in a field as demanding as fintech, it’s no surprise that 58 percent of finance professionals feel overworked. ML-driven automation eliminates the busy work standing in the way between your experts and better tasks.
Predictive analytics for cost reduction
Predictive analytics powered by machine learning supports fintech businesses in identifying areas where cost reduction is possible. For example, in lending, ML can forecast loan defaults, allowing lenders to allocate resources more efficiently to mitigate potential losses.
A similar scenario happens elsewhere in fintech through customer pattern analysis. By predicting churn, ML can help businesses proactively retain clients and reduce the cost of acquiring new ones.
Resource allocation
Through pattern recognition, machine learning optimizes resource allocation by determining the best allocation of capital, human resources, and technology. Robo-advisors, as mentioned, use ML in fintech investment management to assess individual risk profiles and allocate investments accordingly, ensuring that each client's portfolio aligns with their financial goals and risk tolerance.
In addition, machine learning-driven chatbots provide always-on customer support, efficiently distributing resources to manage a high volume of client inquiries. By doing so, fintech businesses can scale their services without significantly increasing operational costs.
Data processing and analysis
Machine learning can handle large-scale data processing and analysis, so fintech businesses can extract valuable data-driven insights through technologies such as optical character recognition (OCR) and other automated document processing systems.
That’s invaluable not only to reduce a business’ reliance on extensive data analysis teams, and hence costs, but also to automate processes such as loan application processing, Know Your Customer (KYC) checks, and regulatory compliance.
Successful fintech machine learning projects to learn from
Machine learning has been, in one way or another, delivering value to fintech for well over a decade. Some great cases from around the world, and in multiple verticals, include:
JPMorgan Chase’s Contract Intelligence
Back in 2017, the US’ biggest bank launched a contract intelligence (COiN) platform that heavily leverages natural language processing (NLP), the ML discipline for computers to understand human speech and handwriting.
COiN’s main purpose was to automate repetitive manual tasks, such as the review of commercial credit agreements that, in JPMorgan Chase’s case, was calculated to take up to 360,000 labor hours. COiN could do it in a matter of seconds.
Credgenics
Credgenics, the SaaS-based debt recovery and legal automation company from India, reached an overall loan book worth $47 billion in 2022, handling over 40 million retail loans to date.
Through their machine learning-powered solutions, over 100 enterprise customers have benefitted from higher resolution and collection rates, reduced costs and time for collections, and enhanced legal efficiencies.
Wells Fargo
Wells Fargo is a US-based global financial services company that uses machine learning solutions such as NLP, deep learning, neural networks, and predictive analytics enablers to process single customer data points and data in bulk.
What makes this remarkable? The ability to discern the intent behind a customer's wording in complaints, which might be overlooked in a standard transcript reading. That allows the company to improve processes, deploy more efficient services, and nurture better client relationships.
DealCloud
DealCloud, the leading finance CRM and deal management platform, sought our expertise back in 2016 to modernize their legacy CRM infrastructure. Our experts formed seven teams across web development, DevOps, and QA, scaling from six to 126 professionals and delivering a world-class digital transformation process that helped DealCloud to be acquired by Intapp in 2018.
In 2020, Vention’s team doubled in size to work on DealCloud’s AI and cloud technologies modules to scale their deal sourcing, business development, fundraising, and general operations.
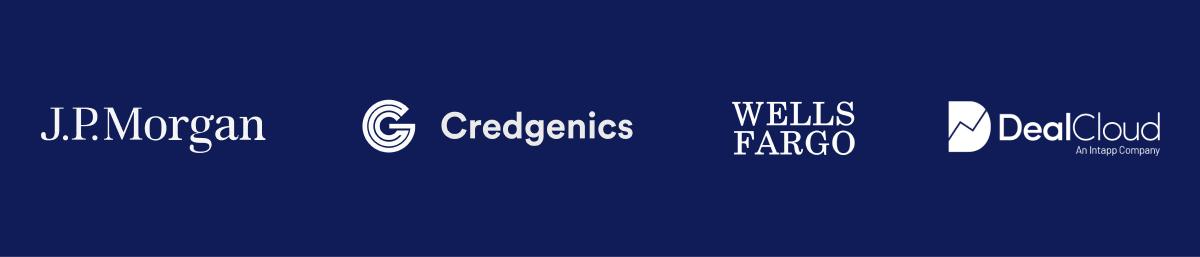
The AI revolution, machine learning solutions, and fintech
Out of the many professional industries scared about AI doomsday scenarios, fintech isn’t one. That’s not to say that trading companies don’t fear the potential repercussions of AI-fueled fake data — or that fintech workers aren’t looking warily over their shoulders.
But the truth is that none of this breakneck pace of modernization imposed by tech is new to fintech. It’s literally in the industry name: technology is what makes fintech so advanced and hyper-connected. It’s what makes the fintech workforce one of the most technologically qualified of any industry. To many, it’s what attracts them to fintech in the first place. Our specialists know it all too well.
“To those outside fintech, the industry may feel as if lagging behind in terms of machine learning applications since fintech companies tend to benefit more from classic ML solutions such as linear regression, SVM (support vector machine), SVR (support vector regression), and GBM,” observes Andrei.
“To those outside fintech, the industry may feel as if lagging behind in terms of machine learning applications since fintech companies tend to benefit more from classic ML solutions such as linear regression, SVM (support vector machine), SVR (support vector regression), and GBM,” observes Andrei.
“But the truth is that more and more advanced models find their way into fintech applications day after day,” he says. “Every major company has either already launched or is working on one right now, and most are doing a great job of keeping their cards close to the chest.”
Our Vention teams understand how concepts like AI and ML in fintech enable the tools that propel the entire industry forward — that’s why we strive to be on top of the latest tech breakthroughs. Having completed 100+ fintech projects and helped fintech companies raise $1.5B in funding, we know the drill when it comes to both fintech software development and AI solutions. If you're looking to enhance your ROI and operations in these domains, we're just one 'contact us' form away.